Sample Efficient Reinforcement Learning Using Graph-Based Memory Reconstruction.
IEEE Trans. Artif. Intell.(2024)
摘要
Reinforcement learning (RL) algorithms typically require orders of magnitude more interactions than humans to learn effective policies. Research on memory in neuroscience suggests that humans' learning efficiency benefits from associating their experiences and reconstructing potential events. Inspired by this finding, we introduce a human brain-like memory structure for agents and build a general learning framework based on this structure to improve the RL sampling efficiency. Since this framework is similar to the memory reconstruction process in psychology, we name the newly proposed RL framework as Graph-Based Memory Reconstruction (GBMR). In particular, GBMR first maintains an attribute graph on the agent's memory and then retrieves its critical nodes to build and update potential paths among these nodes. This novel pipeline drives the RL agent to learn faster with its memory-enhanced value functions and reduces interactions with the environment by reconstructing its valuable paths. Extensive experimental analyses and evaluations in the Grid Maze and some challenging Atari environments demonstrate GBMR's superiority over traditional RL methods. We will release the source code and trained models to facilitate further studies in this research direction.
更多查看译文
关键词
Experience replay,graph model,memory reconstruction,reinforcement learning,sample efficiency
AI 理解论文
溯源树
样例
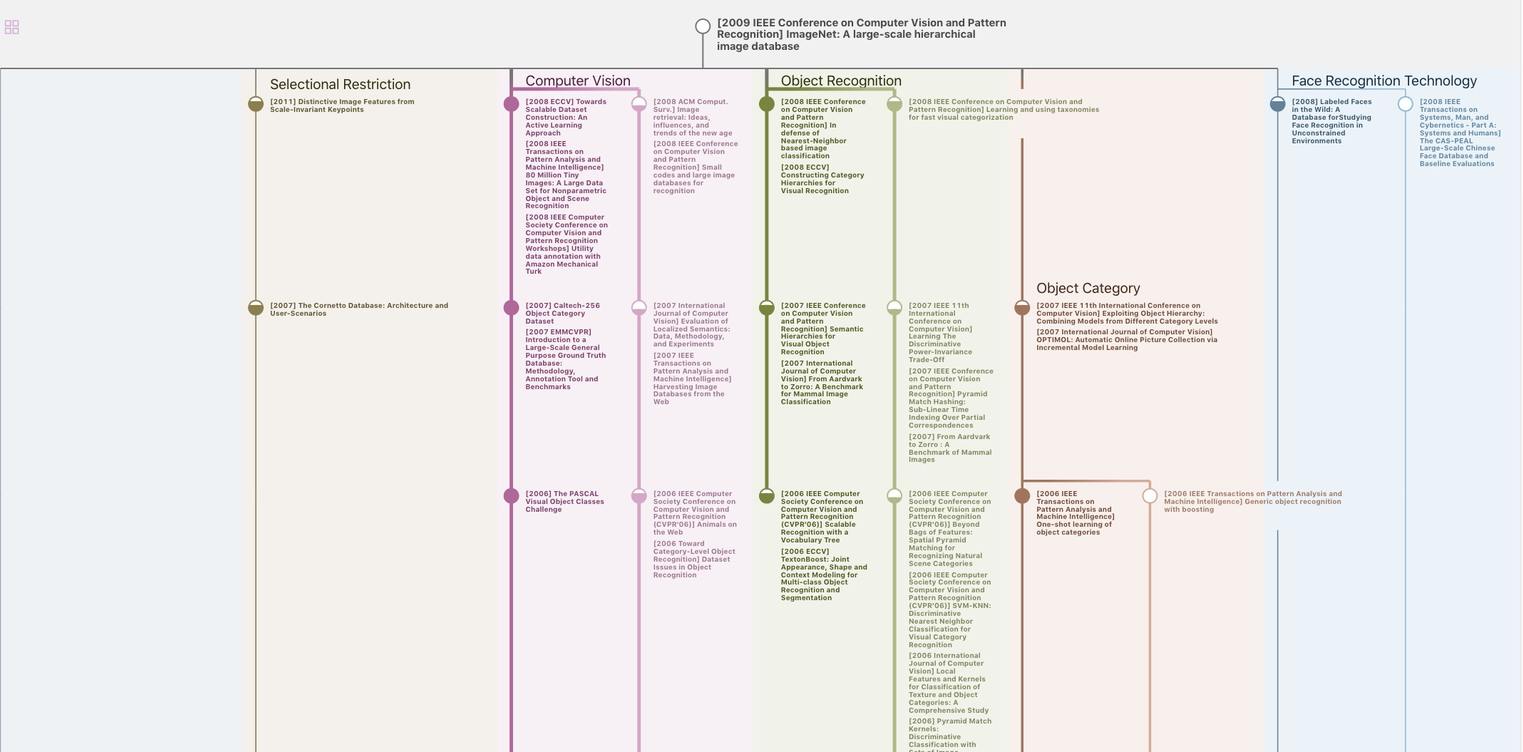
生成溯源树,研究论文发展脉络
Chat Paper
正在生成论文摘要