Abstract 5440: Deep-learning model for tumor type classification enables enhanced clinical decision support in cancer diagnosis
Cancer Research(2023)
摘要
Abstract Background: Knowledge of a patient’s tumor type is essential for guiding clinical treatment decisions in cancer, but histologically-based diagnosis remains challenging for a subset of cancers. Genomic alterations are highly indicative of tumor type and can be used to build classifiers that predict diagnoses, but most genomic-based classification methods use whole genome sequencing (WGS) data which is not feasible for widespread clinical implementation at present. Clinical sequencing is typically performed using cancer gene panels that target individual mutations, often drivers, but previous tumor type classifiers developed using driver-based features alone perform poorly. We hypothesize that a classifier developed using state-of-the-art deep-learning methods and a sufficiently large training cohort would be able to overcome previous accuracy limitations and support the development of a clinically-relevant tumor type prediction model. Methods: We present Deep Genome-Derived Diagnosis (GDD-ENS), an ensemble-based deep-learning tumor type classification method trained using data from cancer gene panel sequencing. We specifically use data from MSK-IMPACT, an FDA-authorized clinical sequencing assay that reports genomic alterations including mutations, indels, copy number alterations, and gene fusions across 505 cancer-associated genes. We aggregated a discovery cohort of 35,372 patients with solid tumors profiled with MSK-IMPACT across 38 common cancer types and used this set to generate 4,487 somatic mutation features for development. Results: GDD-ENS achieves 78.8% accuracy on a held out validation cohort of 6971 patients. For the 71.9% of predictions assigned a high confidence by the model, accuracy increases to 92.7%, rivaling WGS-based models. We use Shapley Values to report prediction-specific feature importance, and aggregate them across cancer types to show GDD-ENS identifies known cancer type-genomic alteration trends. GDD-ENS also, with high accuracy, identifies patients with cancer types not included in the 38 common types using metrics derived from ensemble statistics. For patients where non-genomic information could further guide predictions, we implement a customizable prediction-specific adaptive prior distribution and report improved accuracy after adjusting predictions to account for features such as metastatic sample biopsy site. Finally, we apply GDD-ENS to a set of 1,123 patients with Cancers of Unknown Primary (CUP) and return high confidence predictions for 49% of these patients, in some cases matching predictions on CUP samples with diagnoses that were later confirmed through additional sampling and disease progression. Conclusions: Integrating GDD-ENS into prospective clinical sequencing workflows will enable clinically-relevant tumor type predictions that can guide treatment decisions in real-time. Citation Format: Madison Darmofal, Shalabh Suman, Gurnit Atwal, Jie-Fu Chen, Anna Varghese, Jason C. Chang, Anoop Balakrishnan Rema, Aijazuddin Syed, Quaid Morris, Michael Berger. Deep-learning model for tumor type classification enables enhanced clinical decision support in cancer diagnosis. [abstract]. In: Proceedings of the American Association for Cancer Research Annual Meeting 2023; Part 1 (Regular and Invited Abstracts); 2023 Apr 14-19; Orlando, FL. Philadelphia (PA): AACR; Cancer Res 2023;83(7_Suppl):Abstract nr 5440.
更多查看译文
关键词
tumor type classification,cancer diagnosis,deep-learning deep-learning model,deep-learning deep-learning
AI 理解论文
溯源树
样例
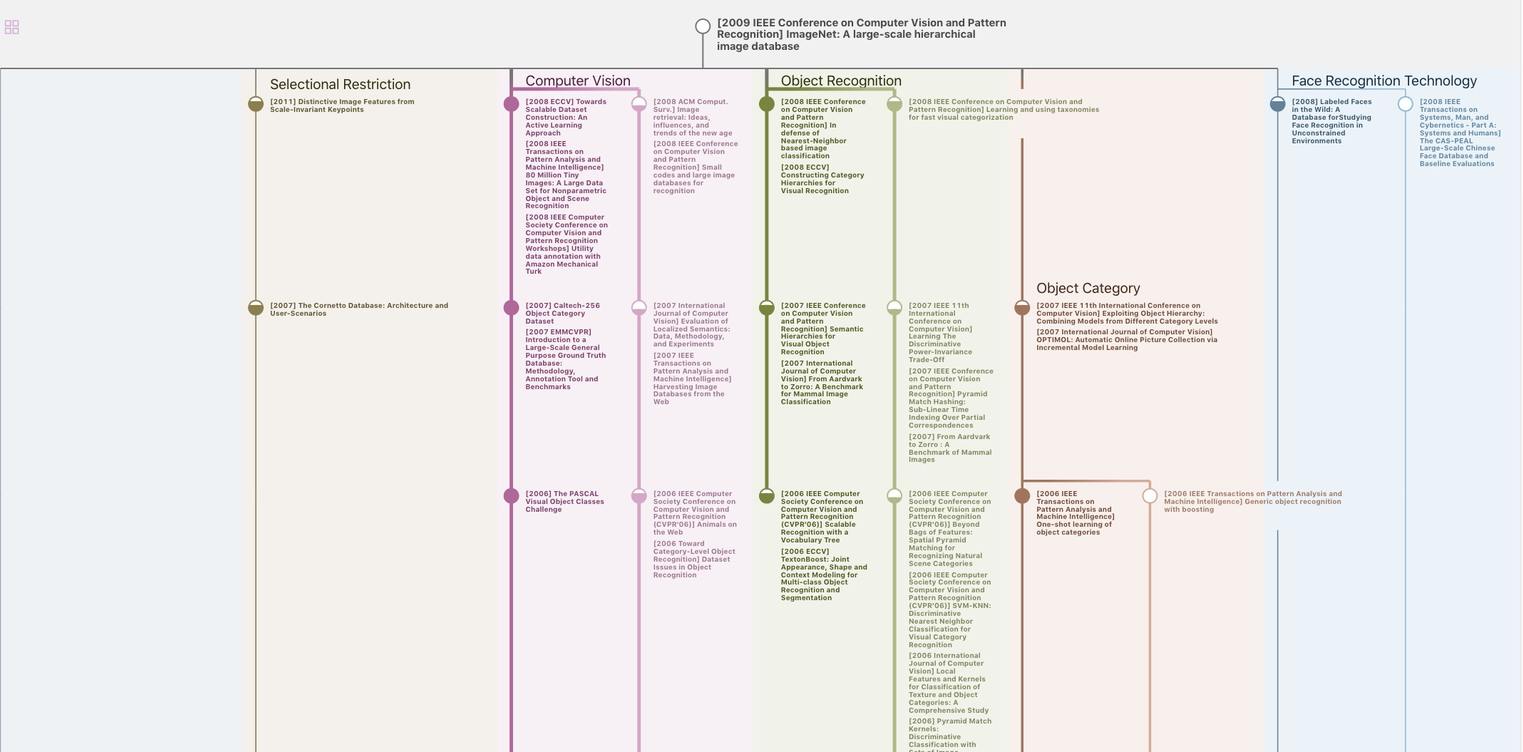
生成溯源树,研究论文发展脉络
Chat Paper
正在生成论文摘要