Abstract 5701: Predictive biomarker models of immunotherapy response in patients with metastatic melanoma: genomic, transcriptomic, and immune profiles from the Personalised Immunotherapy Program (PIP)
Cancer Research(2023)
摘要
Abstract Background: While immune checkpoint inhibitors (ICI) have become the standard-of-care for advanced melanoma patients, only half of treated patients will survive beyond 5-years and many develop significant toxicity. The PIP study is combining pre-treatment clinical, molecular, and immunological profiles of melanoma patients to provide accurate prediction of response to ICI therapies. Methods: 504 patients with advanced melanoma who received anti-PD-1±CTLA-4 ICI were studied to develop predictive models of resistance to ICI. Resistance was defined as patients with progressive disease as best response, or partial response/stable disease with <6 months progression-free survival (RECIST 1.1). Machine learning models were developed using clinicopathological characteristics, tumor mutation burden (TMB, Qiaseq TMB IO), gene expression profiling ((GEP), Nanostring Pancancer 360 IO) and spatial quantitative pathology immune profiling (multiplex immunofluorescence, (MIF)) of baseline melanoma biopsies. Models were developed in a discovery cohort (n=247), validated in an independent cohort (n=97), with an accruing prospective cohort (n=160). Model predictive performance was assessed using the area under the curve (AUC). Results: Models were developed and validated using sequential addition of omics features to relevant clinical factors. Baseline clinical data alone achieved an AUC of 68%. Clinical plus three-tier TMB (<10, 10-20, >20 mut/mb) achieved an AUC of 78%. Clinical data plus GEP achieved 79% AUC, inclusive of tumor inflammation, antigen presentation and T-cell related signatures. Clinical and MIF spatial pathology achieved an 82% AUC including the distances between T-cells, CD16+ cells and melanoma cells as features in the model. Finally, the combination of TMB and GEP achieved an 83% AUC. Prospective validation of the models is awaiting follow-up milestones. Conclusion: Multi-omic models using pre-treatment tissue and clinicopathology can significantly improve the accuracy of predicting patient outcomes to ICI treatments compared to baseline clinical data alone. Several models may be required based on different omic combinations to account for the reality of biopsy suitability and assay failures. These findings prove personalized precision treatment of patients with immunotherapies is possible in the clinical setting and such approaches should become routine care. Citation Format: Tuba N. Gide, Nurudeen A. Adegoke, Yizhe Mao, Monica Lennox, Saurab Raj, Camelia Quek, Ismael A. Vergara, Nigel Maher, Alison Potter, Robyn P. Saw, John F. Thompson, Andrew J. Spillane, Kerwin F. Shannon, Matteo S. Carlino, Maria Gonzalez, Serigne N. Lo, Alexander M. Menzies, Inês Pires da Silva, Richard A. Scolyer, Georgina V. Long, James S. Wilmott. Predictive biomarker models of immunotherapy response in patients with metastatic melanoma: genomic, transcriptomic, and immune profiles from the Personalised Immunotherapy Program (PIP). [abstract]. In: Proceedings of the American Association for Cancer Research Annual Meeting 2023; Part 1 (Regular and Invited Abstracts); 2023 Apr 14-19; Orlando, FL. Philadelphia (PA): AACR; Cancer Res 2023;83(7_Suppl):Abstract nr 5701.
更多查看译文
关键词
metastatic melanoma,personalised immunotherapy program,immunotherapy response,predictive biomarker models,immune profiles
AI 理解论文
溯源树
样例
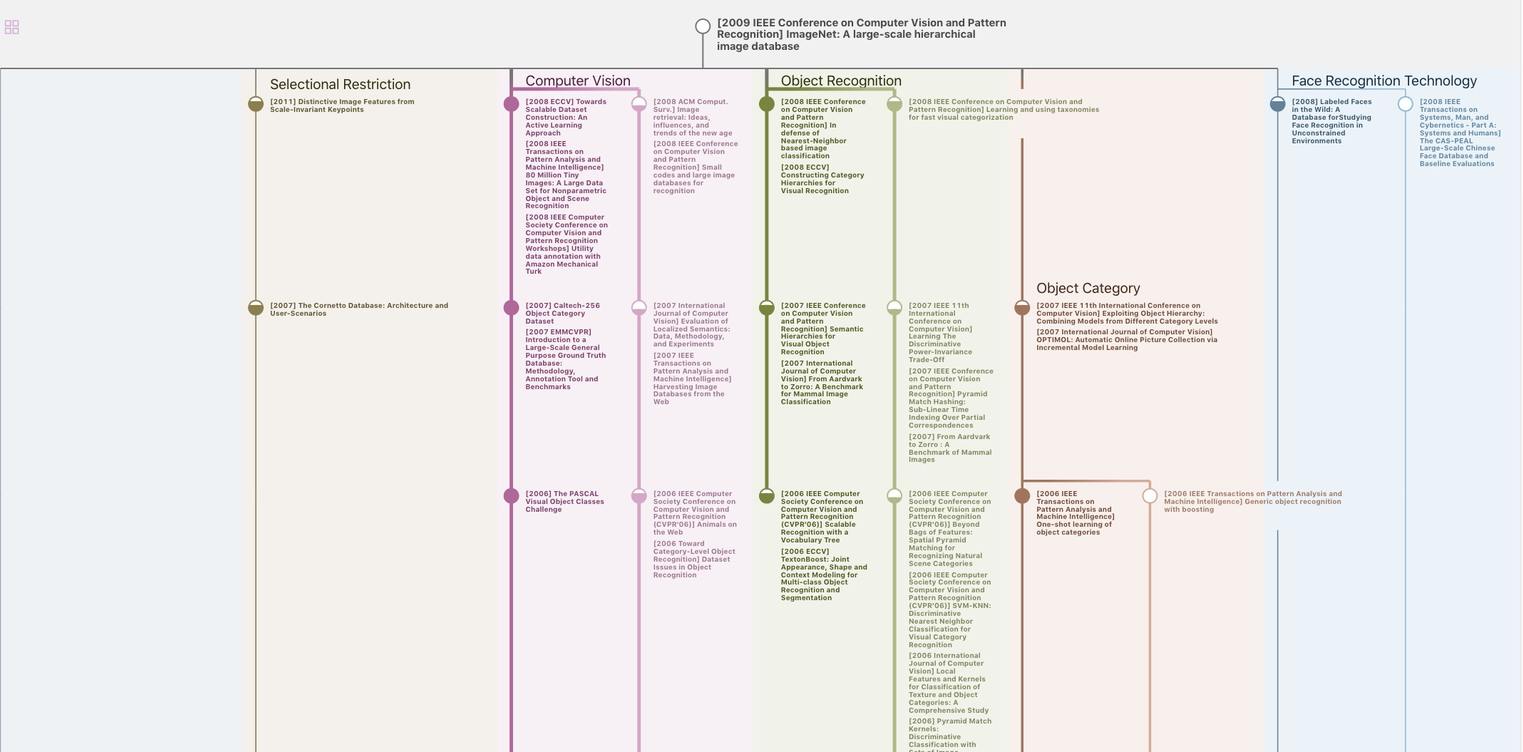
生成溯源树,研究论文发展脉络
Chat Paper
正在生成论文摘要