BRLMSESCT: Design of a Bioinspired Reinforcement Learning Model for Side-Effect Analysis of Spinal Cord Tumours
Research Square (Research Square)(2023)
摘要
Abstract Due to improper postures, and unhealthy lifestyle of millennials, there has been an exponential increase in spinal cord related diseases. These include Slip Discs, Spine Injuries, Tumours, etc. each of which has multiple side-effects on the human body. To analyze these conditions, a wide variety of image processing models are developed by researchers. But most of these models do not analyze side-effects of spinal cord tumours on other body parts, due to which their applicability is limited when used for clinical trials. The main novelty of this work is to analyze side effects resulting due to spinal cord tumours, and to perform this task a novel Bioinspired Reinforcement Learning Model for Side-Effect Analysis of Spinal Cord Tumours is discussed in this text. The proposed model initially uses a Recurrent Neural Network (RNN) based on combination of Long-Short-Term Memory (LSTM) & Gated Recurrent Unit (GRU) for extraction of highly dense image features. These features allow the model to estimate tumour positions in Computer Tomography (CT) scans. The extracted features are classified via the RNN Model, which assists in high accuracy classification & localization of spinal cord tumours. These classification & localization results are linked with blood reports to estimate side-effects on kidney, lungs, heart activity and vitamin levels. To perform this correlation, a Grey Wolf Optimization (GWO) Model is used, which assists in linking tumour type, and size with blood report parameters. The GWO Model evaluates a fitness function, that fuses tumour levels with its side-effects on individual body parts. This fusion is done via analysis of temporal blood reports, which evaluates effects of different tumour types-and-sizes on individual body parameters. Due to a combination of GWO with LSTM & GRU based RNN, the model is capable of showcasing high accuracy of tumour classification, with better precision of correlation with side effects when compared with state-of-the-art models. It was observed that the proposed model was able to achieve 98.5% accuracy for tumour classification, 96.4% correlation precision with kidney diseases, 95.8% correlation precision with lung diseases, 96.2% correlation precision with heart diseases, and 91.5% correlation precision with vitamin deficiencies. Due to such a high performance, the model is capable of deployment for real-time clinical applications.
更多查看译文
关键词
bioinspired reinforcement learning model,spinal cord tumours,spinal cord,learning model,side-effect
AI 理解论文
溯源树
样例
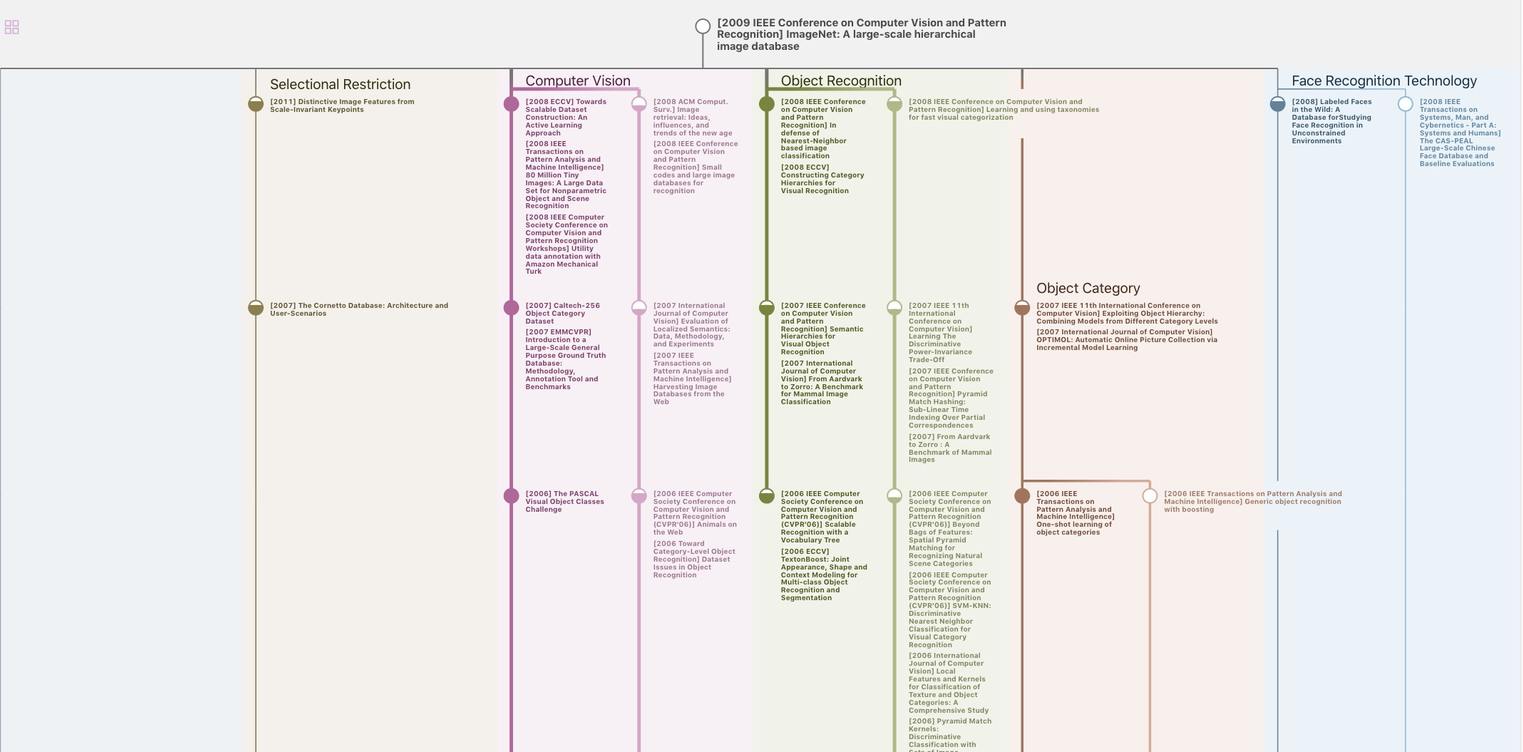
生成溯源树,研究论文发展脉络
Chat Paper
正在生成论文摘要