Assessment of Diagnostic Performance of Dermatologists Cooperating With a Convolutional Neural Network in a Prospective Clinical Study
JAMA Dermatology(2023)
摘要
Importance Studies suggest that convolutional neural networks (CNNs) perform equally to trained dermatologists in skin lesion classification tasks. Despite the approval of the first neural networks for clinical use, prospective studies demonstrating benefits of human with machine cooperation are lacking. Objective To assess whether dermatologists benefit from cooperation with a market-approved CNN in classifying melanocytic lesions. Design, Setting, and Participants In this prospective diagnostic 2-center study, dermatologists performed skin cancer screenings using naked-eye examination and dermoscopy. Dermatologists graded suspect melanocytic lesions by the probability of malignancy (range 0-1, threshold for malignancy ≥0.5) and indicated management decisions (no action, follow-up, excision). Next, dermoscopic images of suspect lesions were assessed by a market-approved CNN, Moleanalyzer Pro (FotoFinder Systems). The CNN malignancy scores (range 0-1, threshold for malignancy ≥0.5) were transferred to dermatologists with the request to re-evaluate lesions and revise initial decisions in consideration of CNN results. Reference diagnoses were based on histopathologic examination in 125 (54.8%) lesions or, in the case of nonexcised lesions, on clinical follow-up data and expert consensus. Data were collected from October 2020 to October 2021. Main Outcomes and Measures Primary outcome measures were diagnostic sensitivity and specificity of dermatologists alone and dermatologists cooperating with the CNN. Accuracy and receiver operator characteristic area under the curve (ROC AUC) were considered as additional measures. Results A total of 22 dermatologists detected 228 suspect melanocytic lesions (190 nevi, 38 melanomas) in 188 patients (mean [range] age, 53.4 [19-91] years; 97 [51.6%] male patients). Diagnostic sensitivity and specificity significantly improved when dermatologists additionally integrated CNN results into decision-making (mean sensitivity from 84.2% [95% CI, 69.6%-92.6%] to 100.0% [95% CI, 90.8%-100.0%]; P = .03; mean specificity from 72.1% [95% CI, 65.3%-78.0%] to 83.7% [95% CI, 77.8%-88.3%]; P < .001; mean accuracy from 74.1% [95% CI, 68.1%-79.4%] to 86.4% [95% CI, 81.3%-90.3%]; P < .001; and mean ROC AUC from 0.895 [95% CI, 0.836-0.954] to 0.968 [95% CI, 0.948-0.988]; P = .005). In addition, the CNN alone achieved a comparable sensitivity, higher specificity, and higher diagnostic accuracy compared with dermatologists alone in classifying melanocytic lesions. Moreover, unnecessary excisions of benign nevi were reduced by 19.2%, from 104 (54.7%) of 190 benign nevi to 84 nevi when dermatologists cooperated with the CNN ( P < .001). Most lesions were examined by dermatologists with 2 to 5 years (96, 42.1%) or less than 2 years of experience (78, 34.2%); others (54, 23.7%) were evaluated by dermatologists with more than 5 years of experience. Dermatologists with less dermoscopy experience cooperating with the CNN had the most diagnostic improvement compared with more experienced dermatologists. Conclusions and Relevance In this prospective diagnostic study, these findings suggest that dermatologists may improve their performance when they cooperate with the market-approved CNN and that a broader application of this human with machine approach could be beneficial for dermatologists and patients.
更多查看译文
关键词
dermatologists,convolutional neural network,diagnostic performance,neural network,clinical study
AI 理解论文
溯源树
样例
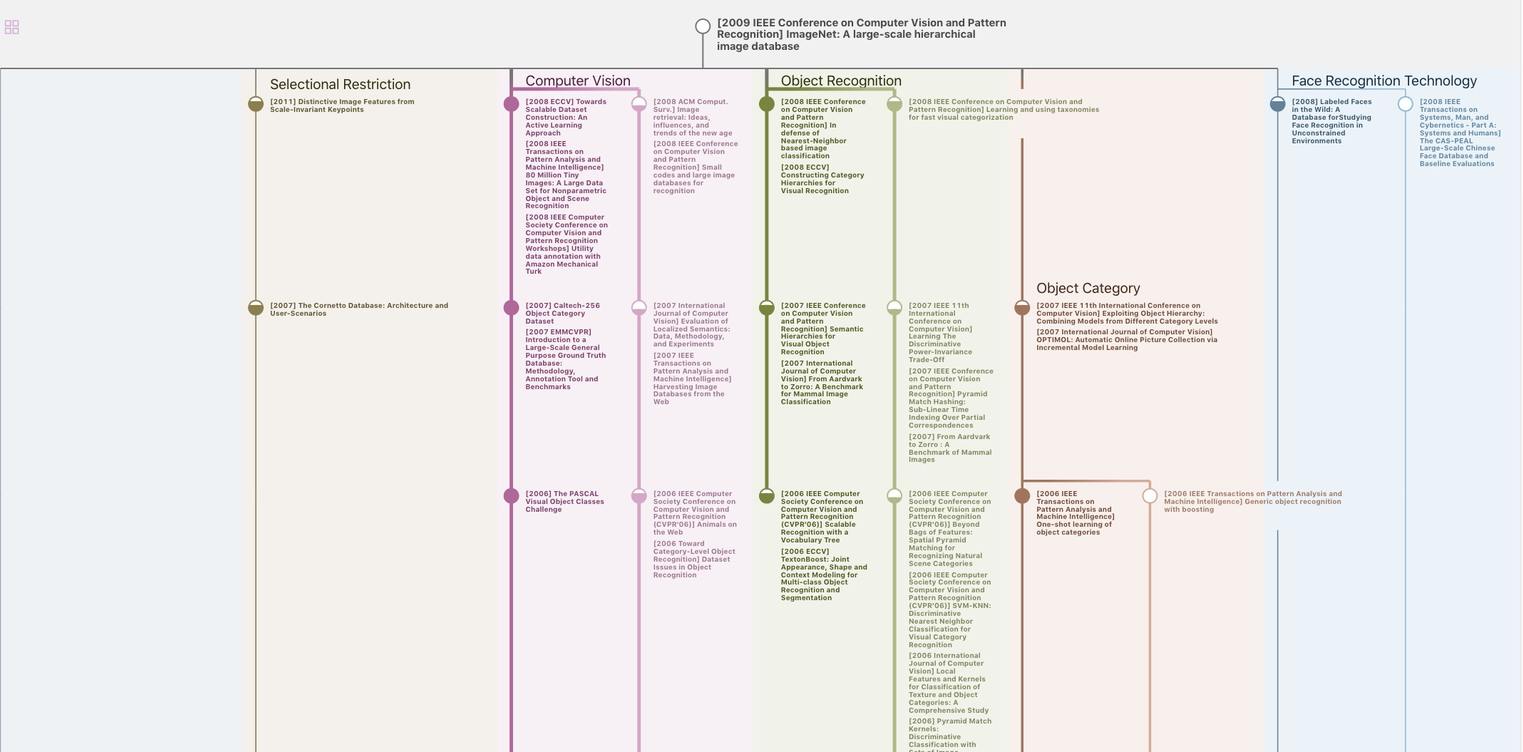
生成溯源树,研究论文发展脉络
Chat Paper
正在生成论文摘要