Data-Driven Discovery of Anomaly-Sensitive Parameters from Uvula Wake Flows Using Wavelet Analyses and Poincar Maps
Acoustics(2023)
摘要
This study presents a data-driven approach to identifying anomaly-sensitive parameters through a multiscale, multifaceted analysis of simulated respiratory flows. The anomalies under consideration include a pharyngeal model with three levels of constriction (M1, M2, M3) and a flapping uvula with two types of kinematics (K1, K2). Direct numerical simulations (DNS) were implemented to solve the wake flows induced by a flapping uvula; instantaneous vortex images, as well as pressures and velocities at seven probes, were recorded for twelve cycles. Principal component analysis (PCA), wavelet-based multifractal spectrum and scalogram, and Poincare mapping were implemented to identify anomaly-sensitive parameters. The PCA results demonstrated a reasonable periodicity of instantaneous vortex images in the leading vector space and revealed distinct patterns between models with varying uvula kinematics (K1, K2). At higher PCA ranks, the periodicity gradually decays, eventually transitioning to a random pattern. The multifractal spectra and scalograms of pressures in the pharynx (P6, P7) show high sensitivity to uvula kinematics, with the pitching mode (K2) having a wider spectrum and a left-skewed peak than the heaving mode (K1). Conversely, the Poincare maps of velocities and pressures in the pharynx (Vel6, Vel7, P6, P7) exhibit high sensitivity to pharyngeal constriction levels (M1-M3), but not to uvula kinematics. The parameter sensitivity to anomaly also differs with the probe site; thus, synergizing measurements from multiple probes with properly extracted anomaly-sensitive parameters holds the potential to localize the source of snoring and estimate the collapsibility of the pharynx.
更多查看译文
关键词
uvula kinematics,pharynx collapse,principal component analysis,wavelet analysis,multifractal spectrum,scalogram,Poincare map
AI 理解论文
溯源树
样例
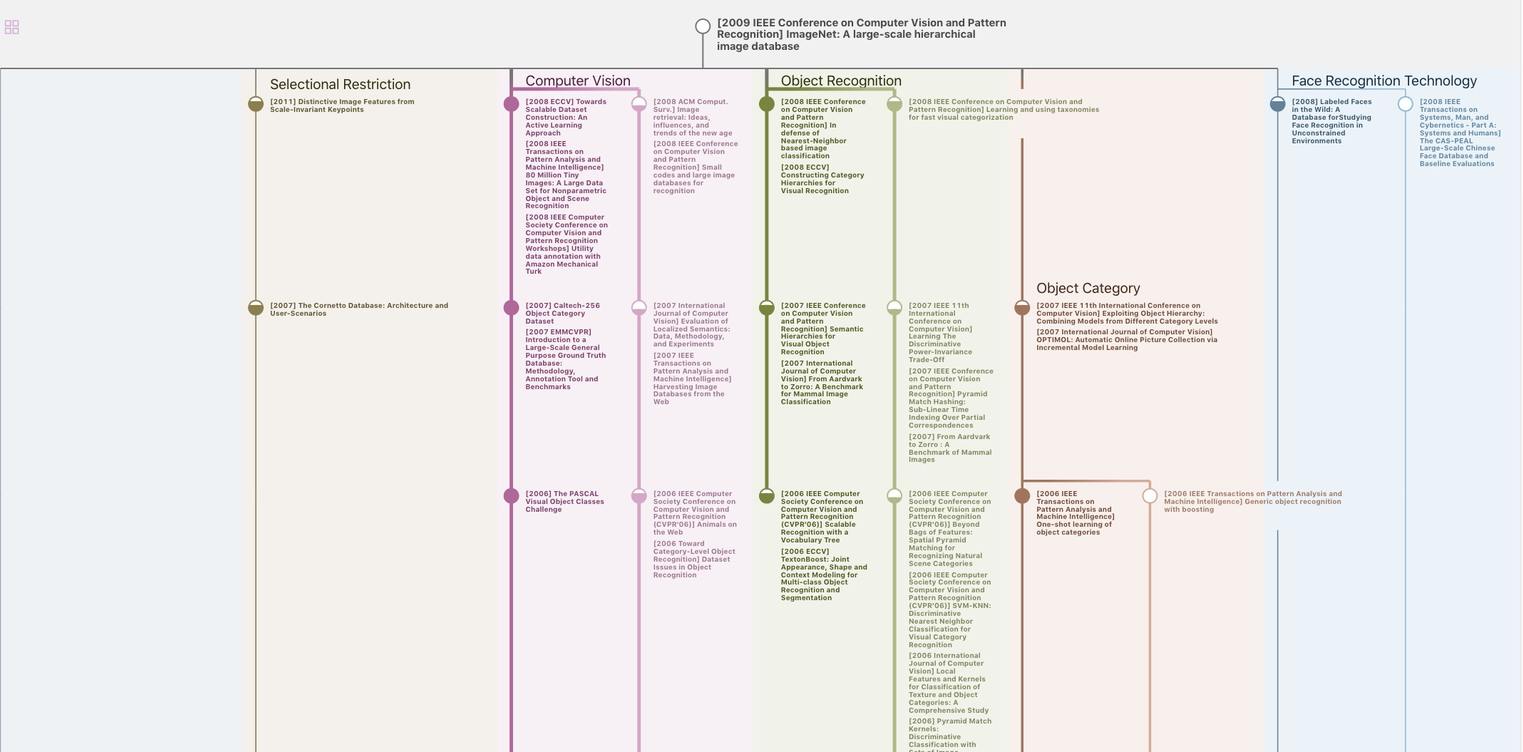
生成溯源树,研究论文发展脉络
Chat Paper
正在生成论文摘要