Resource Management for MEC Assisted Multi-Layer Federated Learning Framework.
IEEE Trans. Wirel. Commun.(2024)
摘要
In this paper, a mobile edge computing (MEC) assisted multi-layer architecture is proposed to support the implementation of federated learning in Internet of Things (IoT) networks. In this architecture, when performing a federated learning based task, data samples can be partially offloaded to MEC servers and cloud server rather than only processing the task at the IoT devices. After collecting local model parameters from devices and MEC servers, cloud server makes an aggregation and broadcasts it back to all devices. An optimization problem is presented to minimize the total federated training latency by jointly optimizing decisions on data offloading ratio, computation resource allocation and bandwidth allocation. To solve the formulated NP hard problem, the optimization problem is converted into quadratically constrained quadratic program (QCQP) and an efficient algorithm is proposed based on semidefinite relaxation (SDR) method. Furthermore, the scenario with the constraint of indivisible tasks in devices is considered and an applicable algorithm is proposed to get effective offloading decisions. Simulation results show that the proposed solutions can get effective resource allocation strategy and the proposed multi-layer federated learning architecture outperforms the conventional federated learning scheme in terms of the learning latency performance.
更多查看译文
关键词
Federated learning,mobile edge computing,cloud radio access network,resource allocation,SDR method
AI 理解论文
溯源树
样例
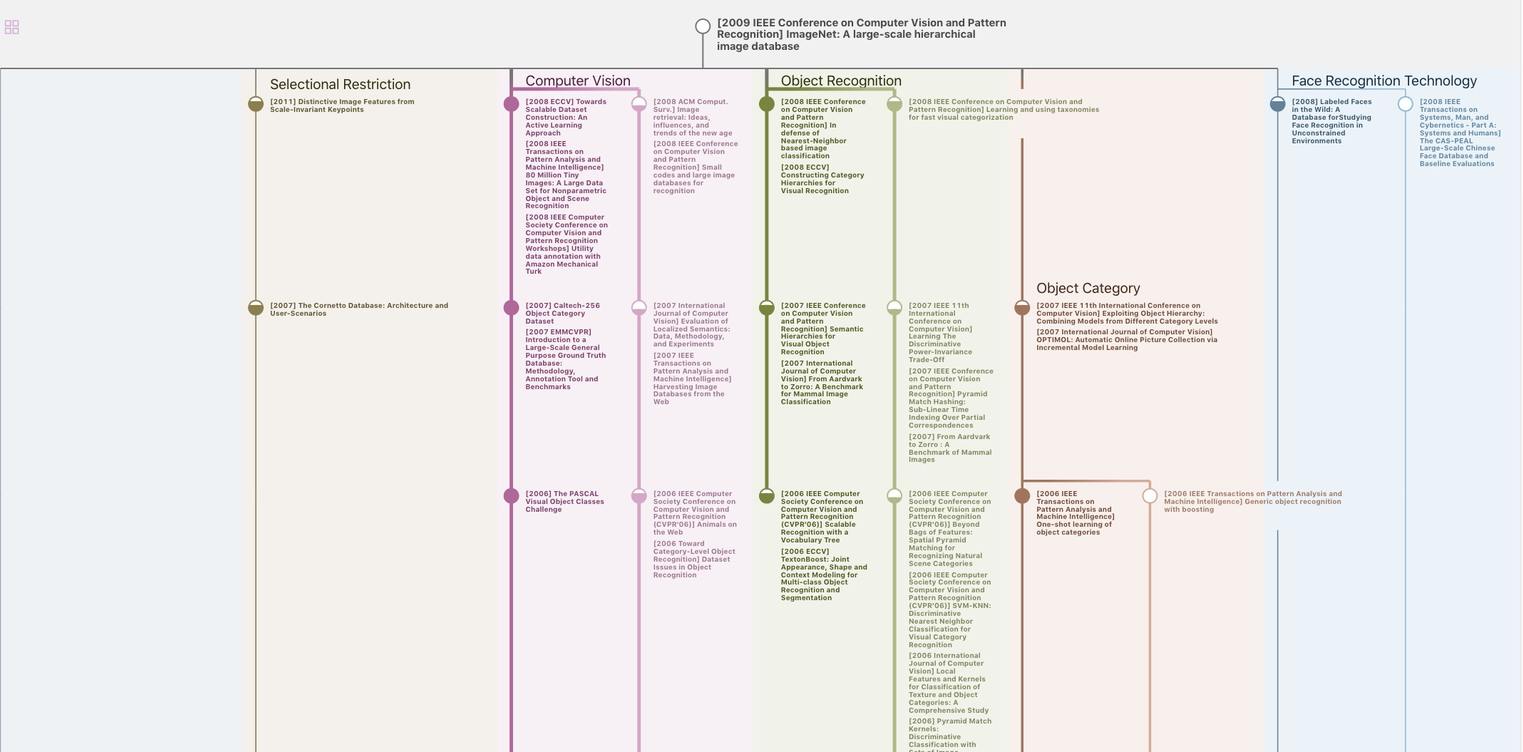
生成溯源树,研究论文发展脉络
Chat Paper
正在生成论文摘要