Towards a Causal Decision-Making Framework for Recommender Systems
ACM Transactions on Recommender Systems(2023)
摘要
Abstract. Causality is gaining more and more attention in the machine learning community and consequently also in recommender systems research. The limitations of learning offline from observed data are widely recognized, however, applying debiasing strategies like Inverse Propensity Weighting does not always solve the problem of making wrong estimates. This concept paper contributes a summary of debiasing strategies in recommender systems and the design of several toy examples demonstrating the limits of these commonly applied approaches. Therefore, we propose to map the causality frameworks of potential outcomes and structural causal models onto the recommender systems domain in order to foster future research and development. For instance, applying causal discovery strategies on offline data to learn the causal graph in order to compute counterfactuals or improve debiasing strategies.
更多查看译文
关键词
recommender systems,decision-making
AI 理解论文
溯源树
样例
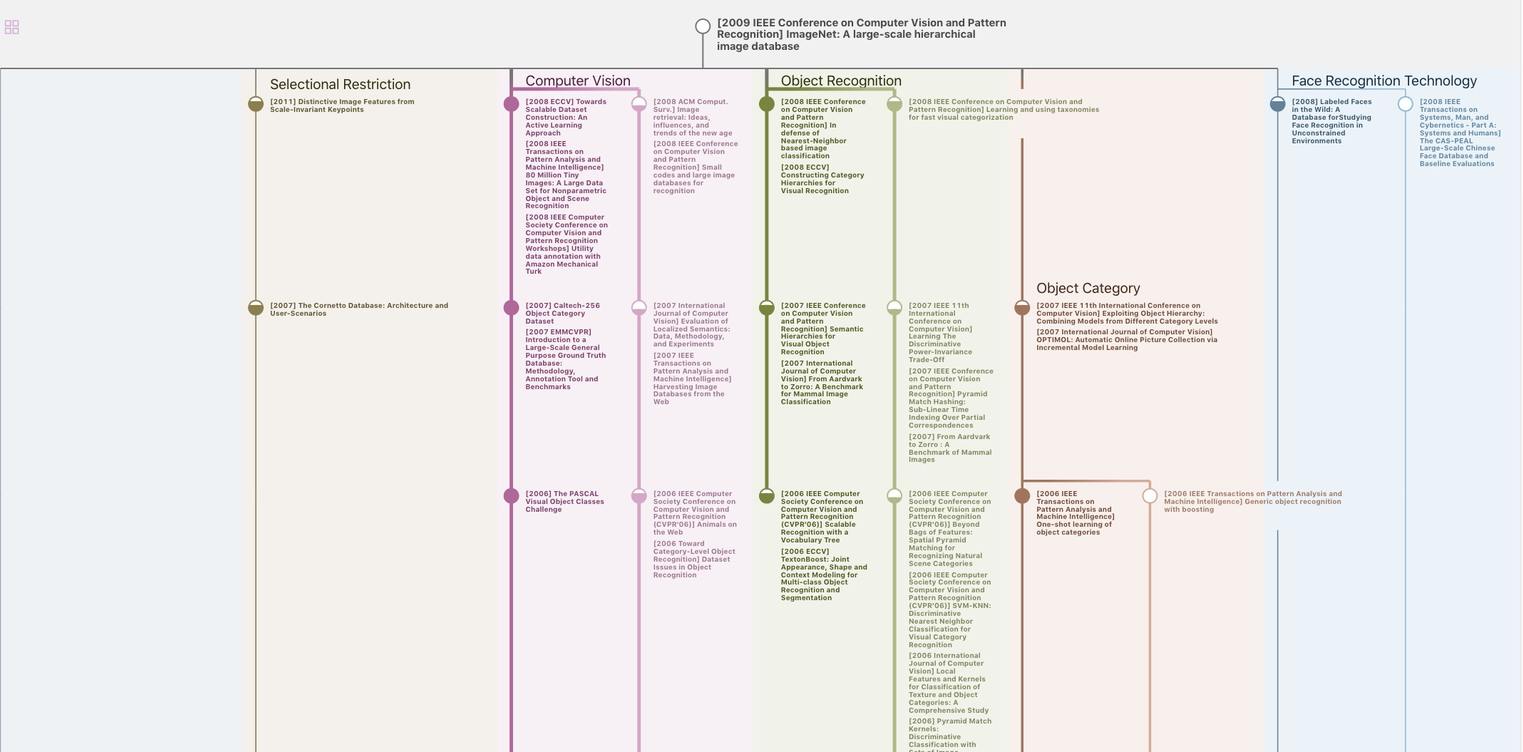
生成溯源树,研究论文发展脉络
Chat Paper
正在生成论文摘要