Learning Gradient Fields for Scalable and Generalizable Irregular Packing
arXiv (Cornell University)(2023)
摘要
The packing problem, also known as cutting or nesting, has diverse applications in logistics, manufacturing, layout design, and atlas generation. It involves arranging irregularly shaped pieces to minimize waste while avoiding overlap. Recent advances in machine learning, particularly reinforcement learning, have shown promise in addressing the packing problem. In this work, we delve deeper into a novel machine learning-based approach that formulates the packing problem as conditional generative modeling. To tackle the challenges of irregular packing, including object validity constraints and collision avoidance, our method employs the score-based diffusion model to learn a series of gradient fields. These gradient fields encode the correlations between constraint satisfaction and the spatial relationships of polygons, learned from teacher examples. During the testing phase, packing solutions are generated using a coarse-to-fine refinement mechanism guided by the learned gradient fields. To enhance packing feasibility and optimality, we introduce two key architectural designs: multi-scale feature extraction and coarse-to-fine relation extraction. We conduct experiments on two typical industrial packing domains, considering translations only. Empirically, our approach demonstrates spatial utilization rates comparable to, or even surpassing, those achieved by the teacher algorithm responsible for training data generation. Additionally, it exhibits some level of generalization to shape variations. We are hopeful that this method could pave the way for new possibilities in solving the packing problem.
更多查看译文
关键词
gradient fields,packing,generalizable irregular
AI 理解论文
溯源树
样例
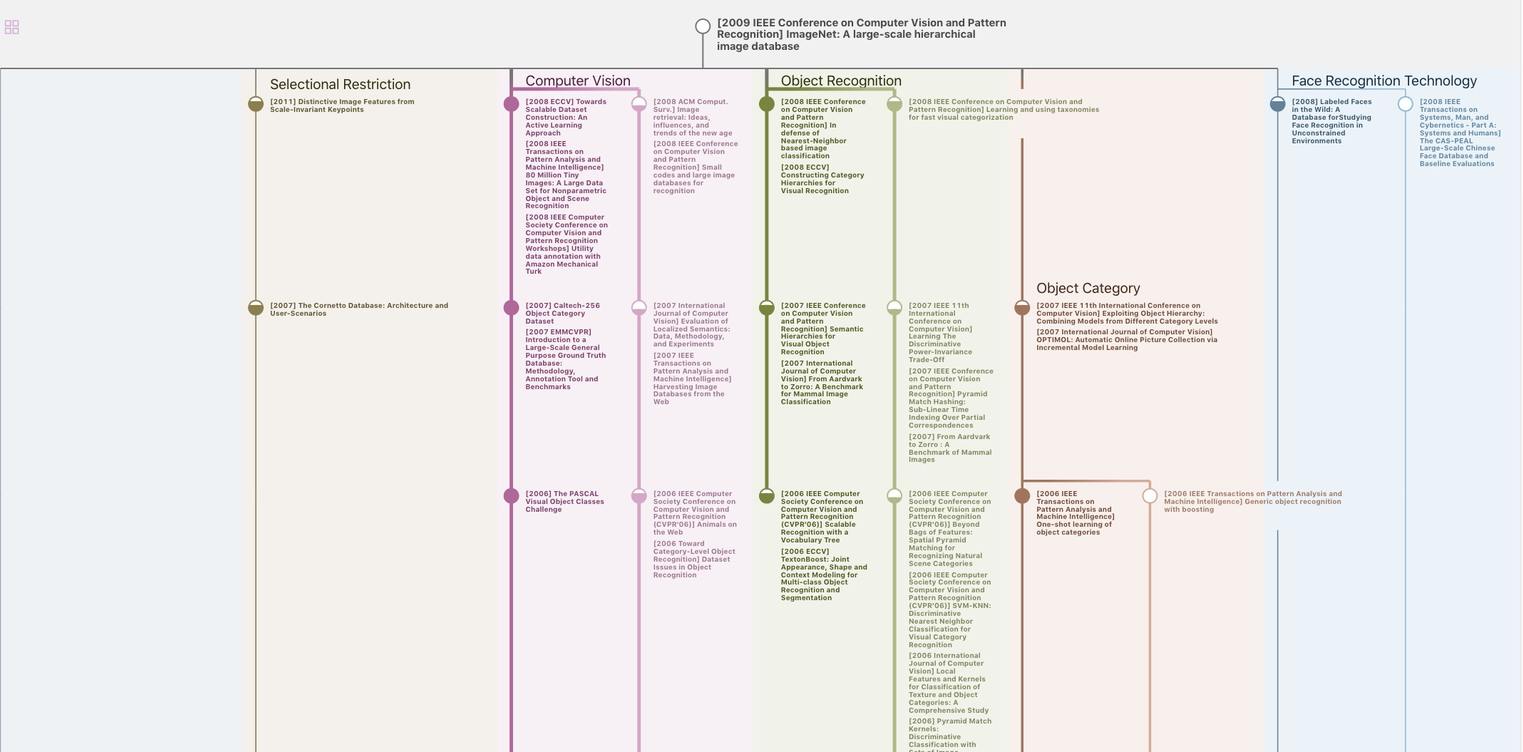
生成溯源树,研究论文发展脉络
Chat Paper
正在生成论文摘要