Machine learning accelerated study for predicting the lattice constant and substitution energy of metal doped titanium dioxide
CERAMICS INTERNATIONAL(2024)
摘要
Currently, titanium dioxide (TiO2) has been extensively studied for its wide applications in many fields, and metal doping is regarded as one of the important methods for modifying TiO2 to improve its performance. Previous explorations on metal doped TiO2 mainly relied on density functional theory (DFT) calculations or chemical experiments, which required lots of time, computation resource, and costs. In this study, we validated the potential of combining machine learning (ML) methods with DFT calculations to improve the efficiency of developing metal doped TiO2 materials. The doped oxide systems comprising 22 different metal elements were investigated, and the lattice constant and substitution energy were calculated via the generalized gradient approximation approach to form the dataset for ML. It is found that through reasonable feature selection and ML modeling validation, the gradient boosting decision tree model performs exceptionally well in predicting the lattice constant and substitution energy. This study provides an effective strategy for developing doped oxide systems based on ML method.
更多查看译文
关键词
Metal doped titanium dioxide,Machine learning,Density functional theory,Lattice constant,Substitution energy
AI 理解论文
溯源树
样例
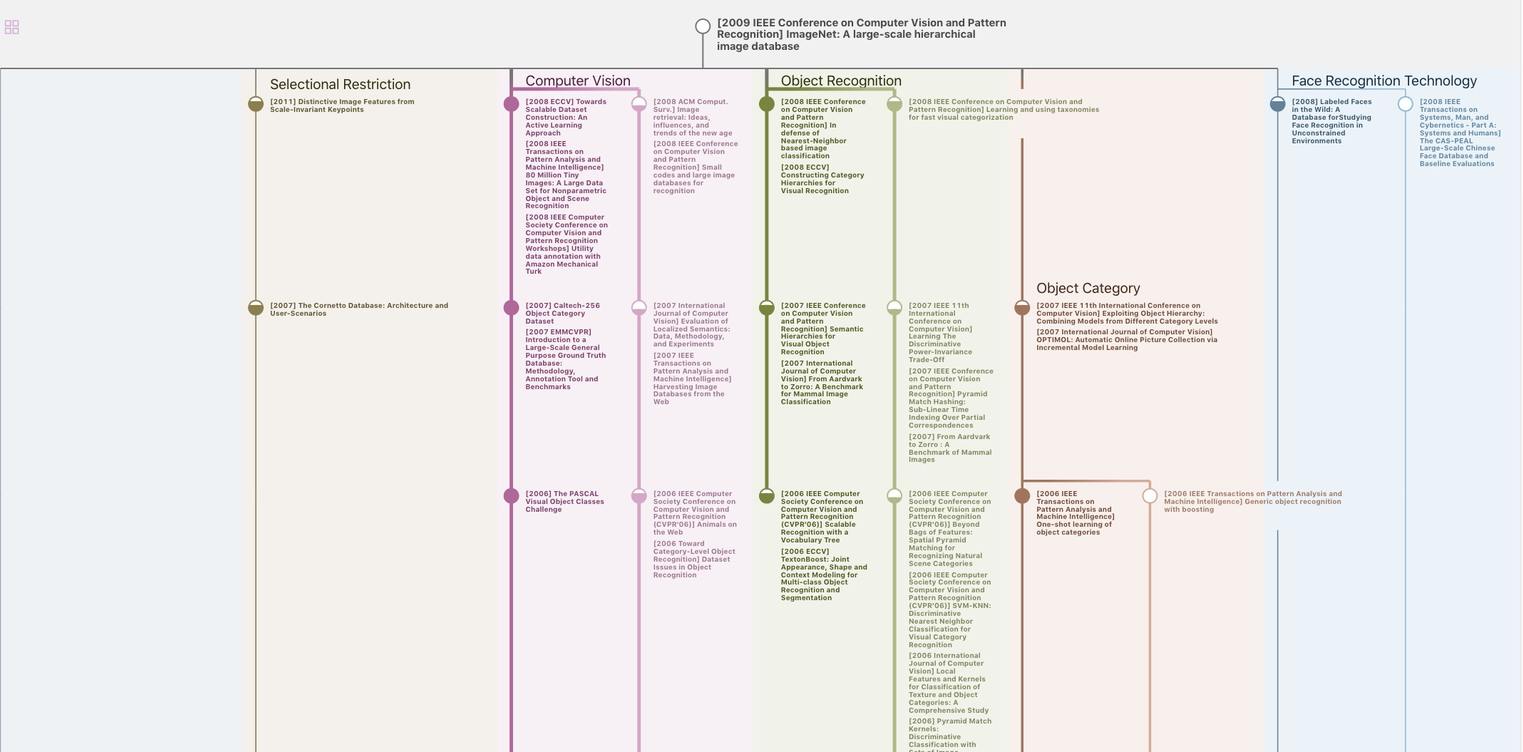
生成溯源树,研究论文发展脉络
Chat Paper
正在生成论文摘要