Adaptive Optimal Consensus Control of Multiagent Systems With Unknown Dynamics and Disturbances via Reinforcement Learning
IEEE transactions on artificial intelligence(2023)
摘要
An adaptive optimal consensus control design technique is presented for uncertain multiagent systems with prescribed performance guarantees using reinforcement learning (RL) algorithm. Firstly, an adaptive neural network identifier is employed to learn the knowledge of uncertain system dynamics and a disturbance observer is developed to compensate for time-varying disturbances. Secondly, a critic-network learning structure is established to obtain the approximate solution of Hamilton-Jacobi-Bellman (HJB) equations of multiagent systems. Then, an experience replay method is applied to update the critic network weights without requiring persistence of excitation condition. Thirdly, RL-based optimized consensus controllers are developed such that (i) the cost function is minimized, (ii) transient and steady-state performances of consensus error systems are guaranteed, and (iii) uniform ultimate boundedness of the closed-loop systems is achieved. Finally, application to consensus control of unmanned surface vehicles with uncertain hydrodynamic dampings is given to demonstrate the effectiveness of the optimal control design technique.
更多查看译文
关键词
Reinforcement learning (RL),neural networks (NNs),prescribed performance,distributed optimal consensus control,unmanned surface vehicles (USVs)
AI 理解论文
溯源树
样例
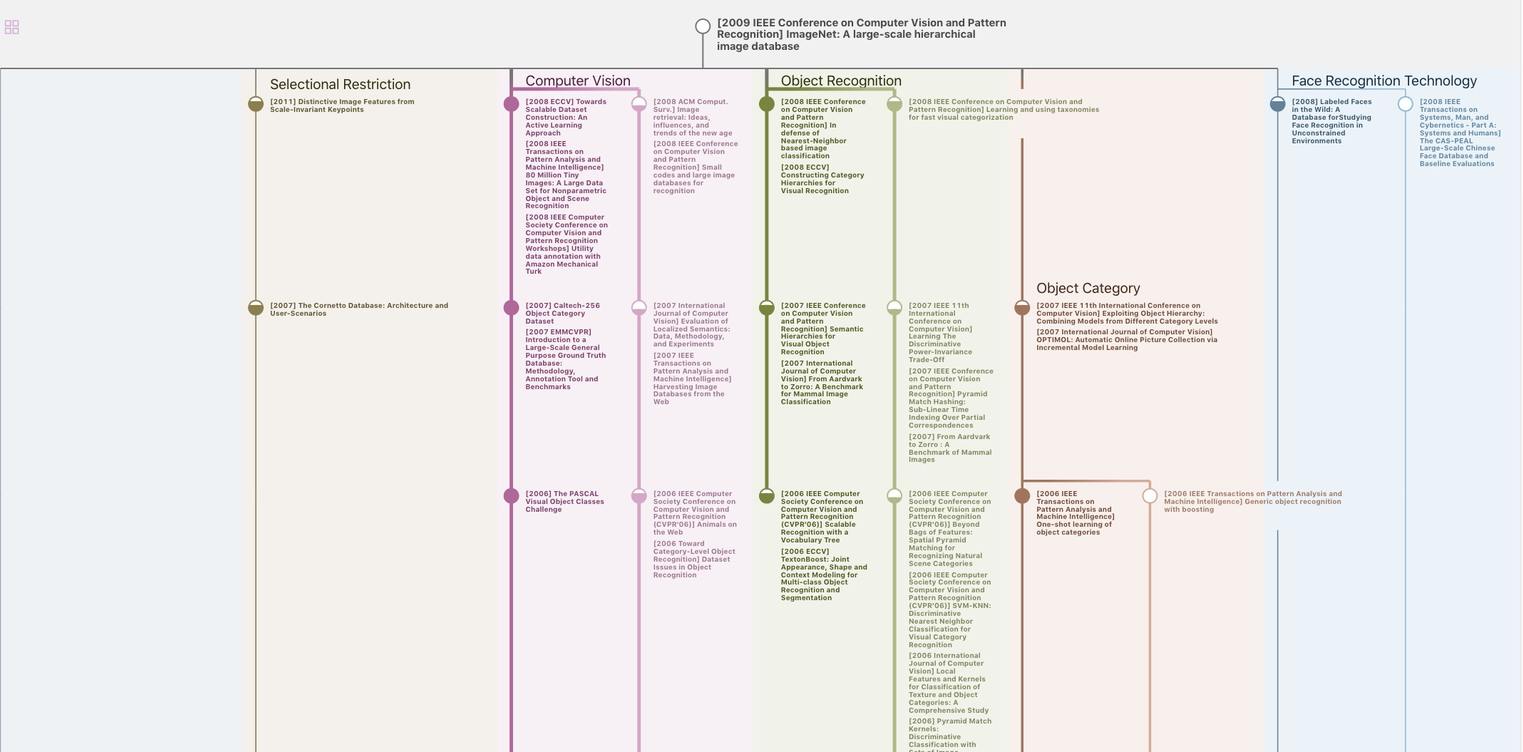
生成溯源树,研究论文发展脉络
Chat Paper
正在生成论文摘要