Federated Submodular Maximization With Differential Privacy
IEEE INTERNET OF THINGS JOURNAL(2024)
摘要
Submodular maximization is a fundamental problem in many Internet of Things applications, such as sensor placement, resource allocation, and mobile crowdsourcing. Despite being intensively studied over the last two decades, the problem of submodular maximization has not yet been considered in an emerging federated computation setting. In this article, we first comprehensively study federated submodular maximization, where a set of clients aims to cooperate in finding a set of items to maximize a monotone submodular function under the orchestration of a central server while providing strong privacy guarantees for their sensitive data. We consider the problem in a client-level differential privacy (DP) setting: the server is not necessarily trusted and the clients should perturb their results locally before sending them to the server. Specifically, we propose a novel approximation algorithm for federated submodular maximization by incorporating client-level DP mechanisms and decomposed function evaluations into the greedy algorithm, along with two heuristics to further reduce the privacy budget, computational cost, and communication overhead. Finally, we perform extensive experiments to demonstrate the effectiveness and efficiency of our proposed algorithms.
更多查看译文
关键词
Approximation algorithm,differential privacy (DP),federated computation,submodular maximization
AI 理解论文
溯源树
样例
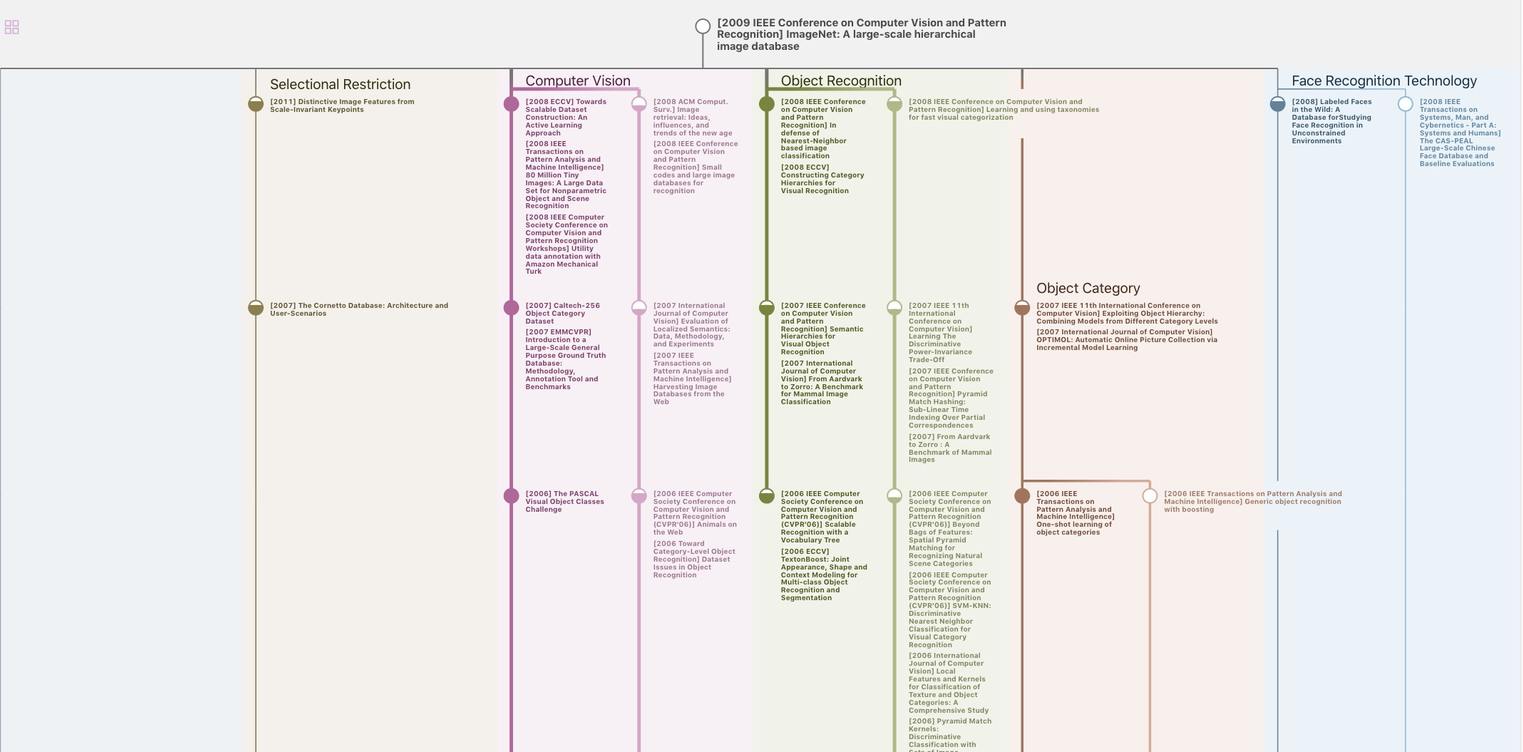
生成溯源树,研究论文发展脉络
Chat Paper
正在生成论文摘要