Physics-informed W-Net GAN for the direct stochastic inversion of fullstack seismic data into facies models
Scientific Reports(2024)
摘要
Predicting the subsurface spatial distribution of geological facies from fullstack geophysical data is a main step in the geo-modeling workflow for energy exploration and environmental tasks and requires solving an inverse problem. Generative adversarial networks (GANs) have shown great potential for geologically accurate probabilistic inverse modeling, but existing methods require multiple sequential steps and do not account for the spatial uncertainty of facies-dependent continuous properties, linking the facies to the observed geophysical data. This can lead to biased predictions of facies distributions and inaccurate quantification of the associated uncertainty. To overcome these limitations, we propose a GAN able to learn the physics-based mapping between facies and seismic domains, while accounting for the spatial uncertainty of such facies-dependent properties. During its adversarial training, the network reads the observed geophysical data, providing solutions to the inverse problems directly in a single step. The method is demonstrated on 2-D examples, using both synthetic and real data from the Norne field (Norwegian North Sea). The results show that the trained GAN can model facies patterns matching the spatial continuity patterns observed in the training images, fitting the observed geophysical data, and with a variability proportional to the spatial uncertainty of the facies-dependent properties.
更多查看译文
关键词
fullstack seismic data,direct stochastic inversion,physics-informed,w-net
AI 理解论文
溯源树
样例
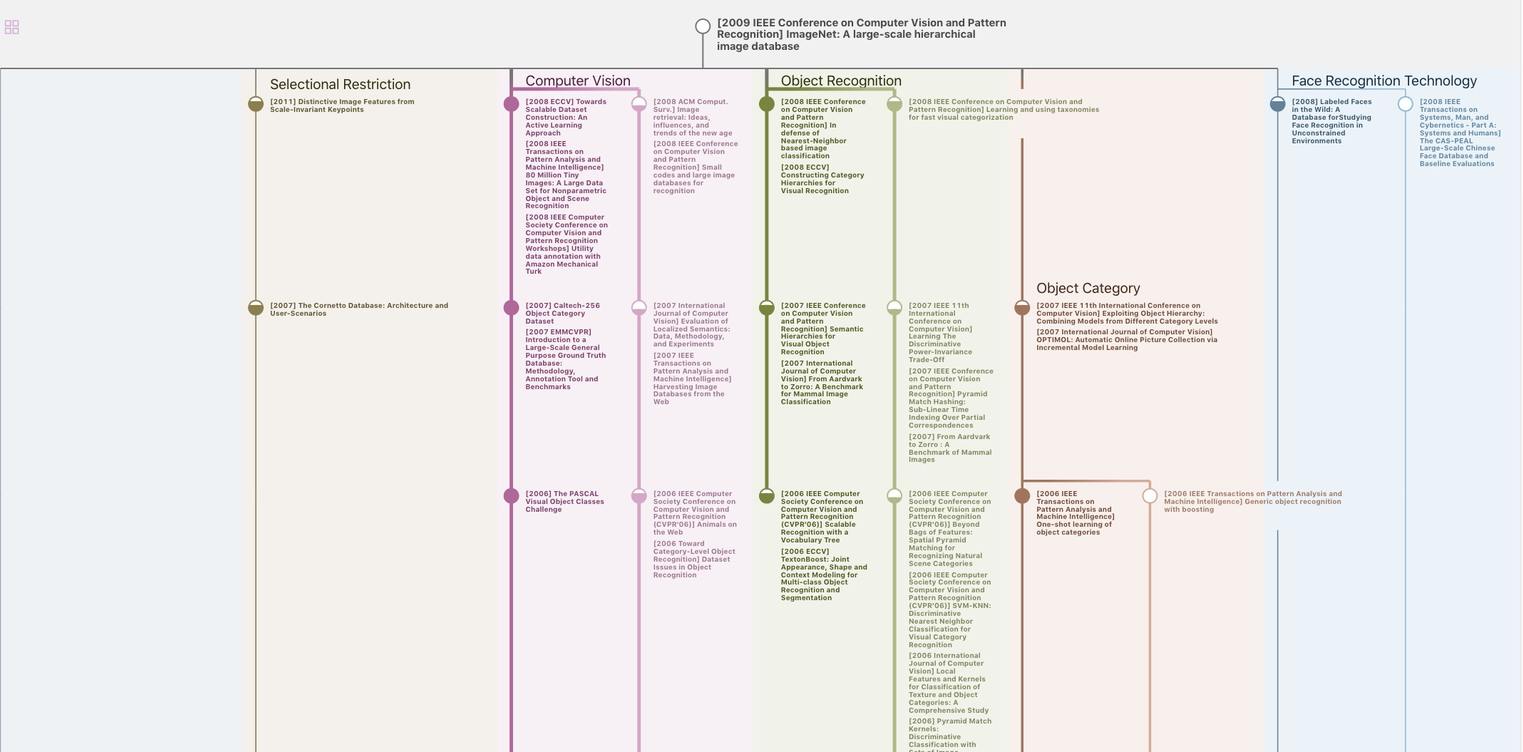
生成溯源树,研究论文发展脉络
Chat Paper
正在生成论文摘要