Domain Complementary Adaptation by Leveraging Diversity and Discriminability From Multiple Sources
IEEE TRANSACTIONS ON MULTIMEDIA(2024)
摘要
Due to the lack of labeled data in many real-world applications, unsupervised domain adaptation has attracted a great deal of attention in the machine learning community through its use of labeled data from source domains. However, how to make full use of the discriminative information from different sources remains a challenge due to various domain gaps. In this article, we propose a domain complementary adaptation method by leveraging the diversity between sources and the discriminability of each source with contrastive learning. In the proposed method, we adopt several branch networks, denoted as domain branch networks, to learn different views of discriminative domain-invariant features from each source. Moreover, an ensemble classification network trained with domain-invariant features from all domain branch networks is adopted to guide the domain branch networks in providing diverse knowledge. We design a domain mutual contrastive loss by forcing the domain branch networks to be different from one another and be consistent with the ensemble classification network to learn diverse domain-invariant features. To further improve the discriminability of domain branch networks, a domain structure-oriented contrastive loss is proposed to learn the discriminative intrinsic neighborhood structure across each source and target domain. Extensive experiments on the Office-31, Office-Home and DomainNet datasets show that the proposed method outperforms state-of-the-art methods.
更多查看译文
关键词
Knowledge engineering,Feature extraction,Prototypes,Loss measurement,Faces,Adaptation models,Task analysis,Unsupervised domain adaptation,diversity,contrastive learning,complementary learning
AI 理解论文
溯源树
样例
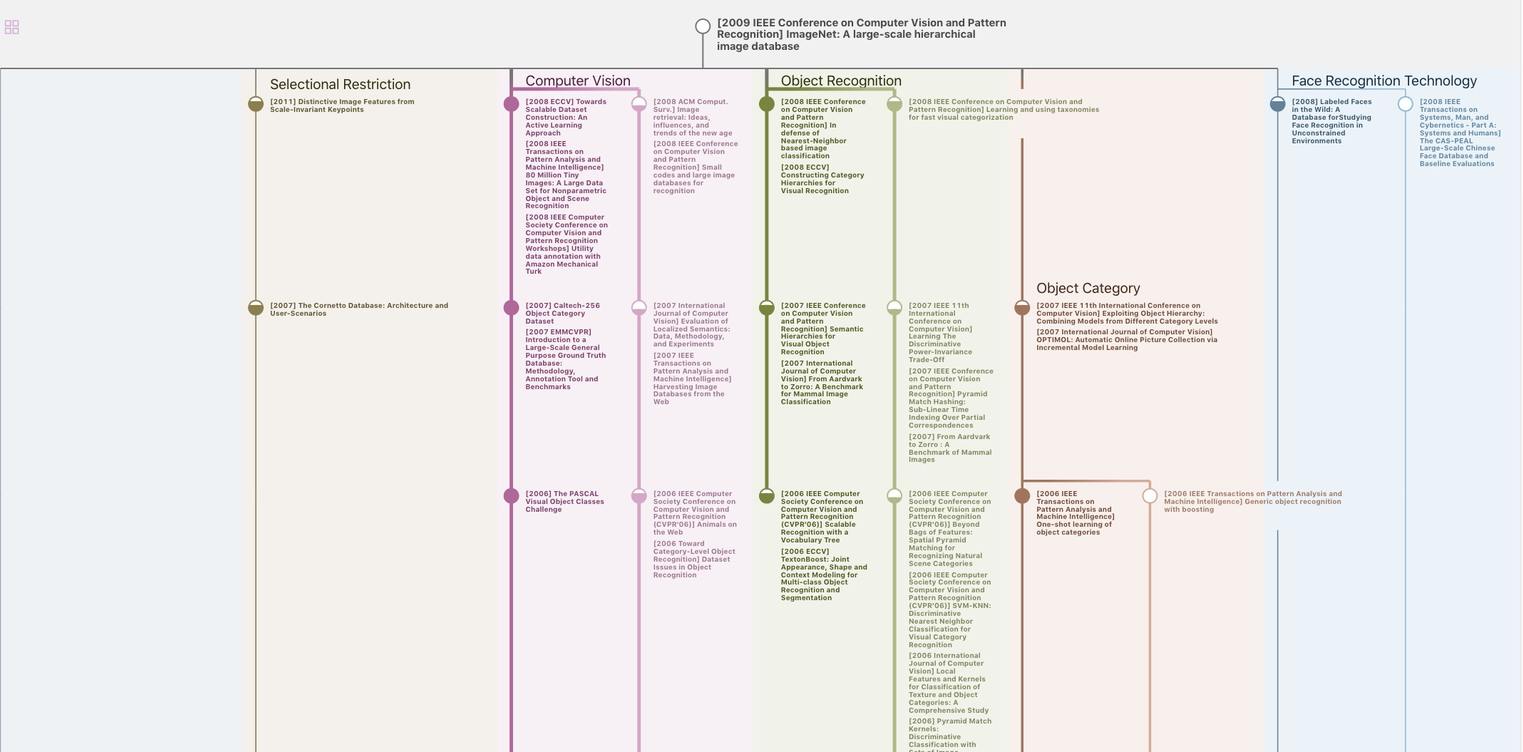
生成溯源树,研究论文发展脉络
Chat Paper
正在生成论文摘要