Generative Quantum Machine Learning via Denoising Diffusion Probabilistic Models.
arXiv (Cornell University)(2024)
摘要
Deep generative models are key-enabling technology to computer vision, text generation, and large language models. Denoising diffusion probabilistic models (DDPMs) have recently gained much attention due to their ability to generate diverse and high-quality samples in many computer vision tasks, as well as to incorporate flexible model architectures and a relatively simple training scheme. Quantum generative models, empowered by entanglement and superposition, have brought new insight to learning classical and quantum data. Inspired by the classical counterpart, we propose the quantum denoising diffusion probabilistic model (QuDDPM) to enable efficiently trainable generative learning of quantum data. QuDDPM adopts sufficient layers of circuits to guarantee expressivity, while it introduces multiple intermediate training tasks as interpolation between the target distribution and noise to avoid barren plateau and guarantee efficient training. We provide bounds on the learning error and demonstrate QuDDPM's capability in learning correlated quantum noise model, quantum many-body phases, and topological structure of quantum data. The results provide a paradigm for versatile and efficient quantum generative learning.
更多查看译文
关键词
generative quantum machine,probabilistic models,diffusion
AI 理解论文
溯源树
样例
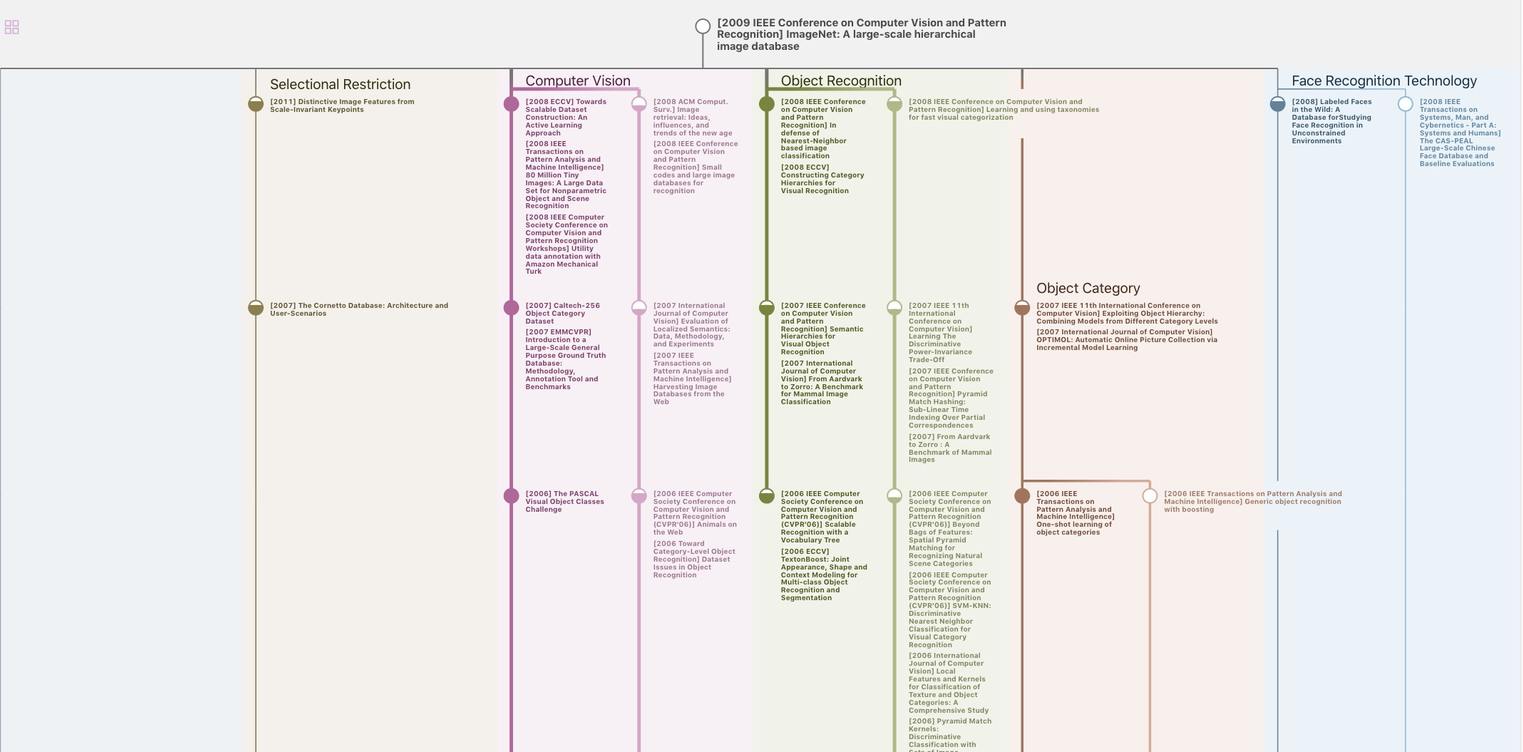
生成溯源树,研究论文发展脉络
Chat Paper
正在生成论文摘要