FP3O: Enabling Proximal Policy Optimization in Multi-Agent Cooperation with Parameter-Sharing Versatility
arXiv (Cornell University)(2023)
摘要
Existing multi-agent PPO algorithms lack compatibility with different types of parameter sharing when extending the theoretical guarantee of PPO to cooperative multi-agent reinforcement learning (MARL). In this paper, we propose a novel and versatile multi-agent PPO algorithm for cooperative MARL to overcome this limitation. Our approach is achieved upon the proposed full-pipeline paradigm, which establishes multiple parallel optimization pipelines by employing various equivalent decompositions of the advantage function. This procedure successfully formulates the interconnections among agents in a more general manner, i.e., the interconnections among pipelines, making it compatible with diverse types of parameter sharing. We provide a solid theoretical foundation for policy improvement and subsequently develop a practical algorithm called Full-Pipeline PPO (FP3O) by several approximations. Empirical evaluations on Multi-Agent MuJoCo and StarCraftII tasks demonstrate that FP3O outperforms other strong baselines and exhibits remarkable versatility across various parameter-sharing configurations.
更多查看译文
关键词
proximal policy optimization,cooperation
AI 理解论文
溯源树
样例
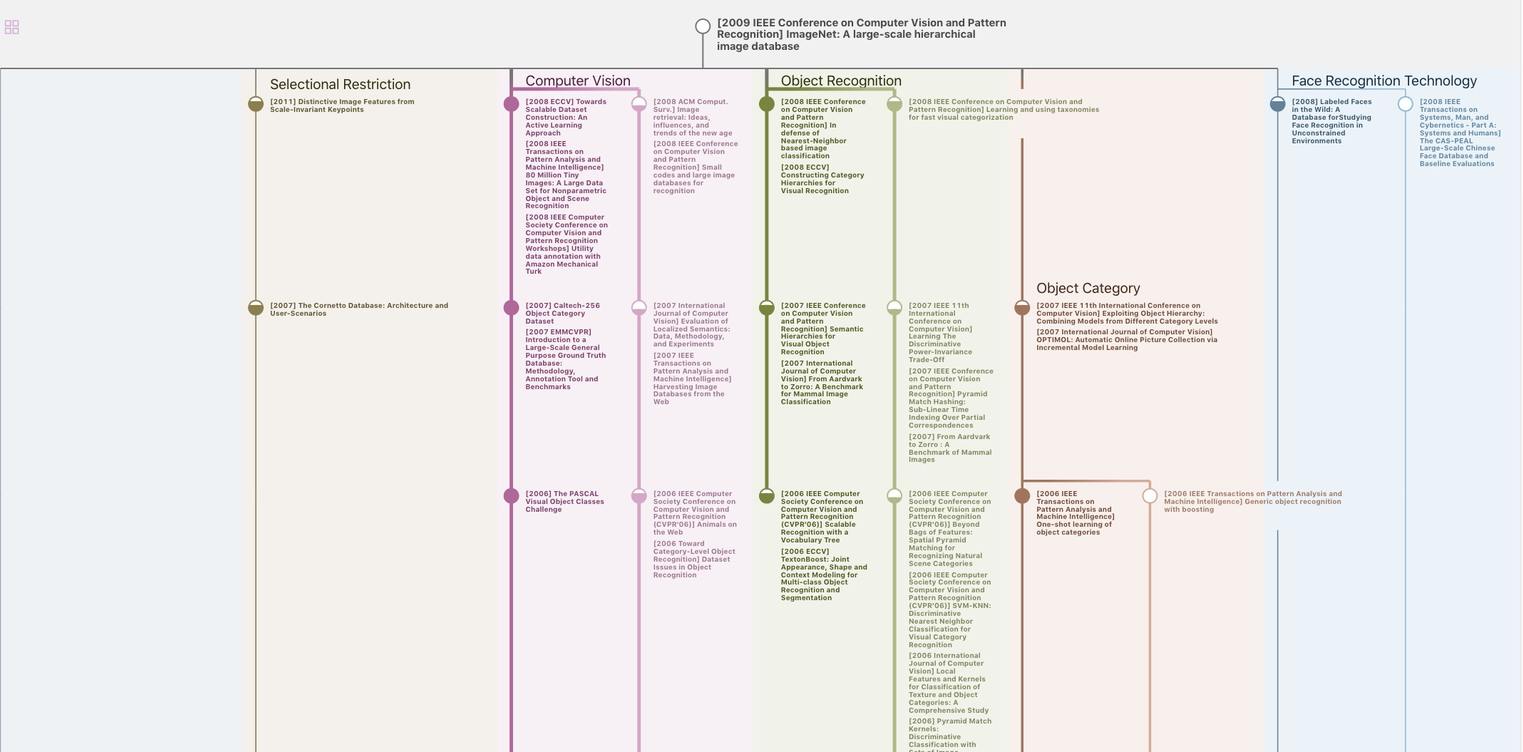
生成溯源树,研究论文发展脉络
Chat Paper
正在生成论文摘要