Clinico-Genomics Model to Predict Survival in Non-Metastatic Breast Cancer: Comparing the Performance of Machine Learning Models
International Journal of Radiation Oncology Biology Physics(2023)
摘要
Breast cancer is a heterogenous spectrum of disease with variable outcome. This heterogeneity of outcome could be driven by underlying heterogeneity in the genotypes. There remains an unmet need of defining prognosis based on underlying genetic heterogeneity. The primary objective of this study was to build a combined clinic-genomics model to predict overall survival (OS) in patients with non-metastatic breast cancer.The Molecular Taxonomy of Breast Cancer International Consortium (METABRIC) database is a combined Canada-UK Project which contains information on 31 demographic, clinical and treatment characteristics in addition to m-RNA level z-score for 331 genes for 1904 breast cancer patients. We excluded patients with metaplastic breast cancer (n = 1) or unknown histologic subtype (n = 13), and those with missing information on breast surgery (n = 22) and cellularity (n = 54). Demographic characteristics included age of the patient and menopausal status while clinical characteristics included tumor stage, number of positive lymph nodes, pathologic grade, cellularity, receptor status for estrogen and progesterone receptors, and HER2neu gene amplification status. Treatment included type of breast cancer surgery, chemotherapy, hormone therapy, and radiation therapy. The data was split randomly at an 80:20 ratio into training and testing dataset. Subsequently, two cross-validated supervised machine learning algorithms were applied to build the prognostic model - elastic net logistic regression and random forest classifier. We compared the performance of the models in the testing dataset using area under receiver operating curve (AUC). All analysis were done using sparklyr - an R interface to Apache SparkTM.Overall, 1814 patients were included in the analysis. The AUC for elastic net logistic regression model and random forest classifier was 0.74, and 0.73, respectively. The sensitivity and specificity of the elastic net logistic regression model was 0.84 and 0.45, respectively while those for the random forest classifier was 0.83 and 0.51, respectively. STAT5A, CASP8, HSD17b11, and CDKN2c were common to the list of important genes predicting OS in both models while age, lymph node count, Nottingham Prognostic Index (NPI), and type of breast surgery were common to the list of clinical factors predicting OS in both models.This study shows that a multivariable prognostic model with combination of clinical and demographic parameters with Z-score expression data can predict for overall survival.
更多查看译文
关键词
predict survival,breast cancer,machine learning models,clinico-genomics,non-metastatic
AI 理解论文
溯源树
样例
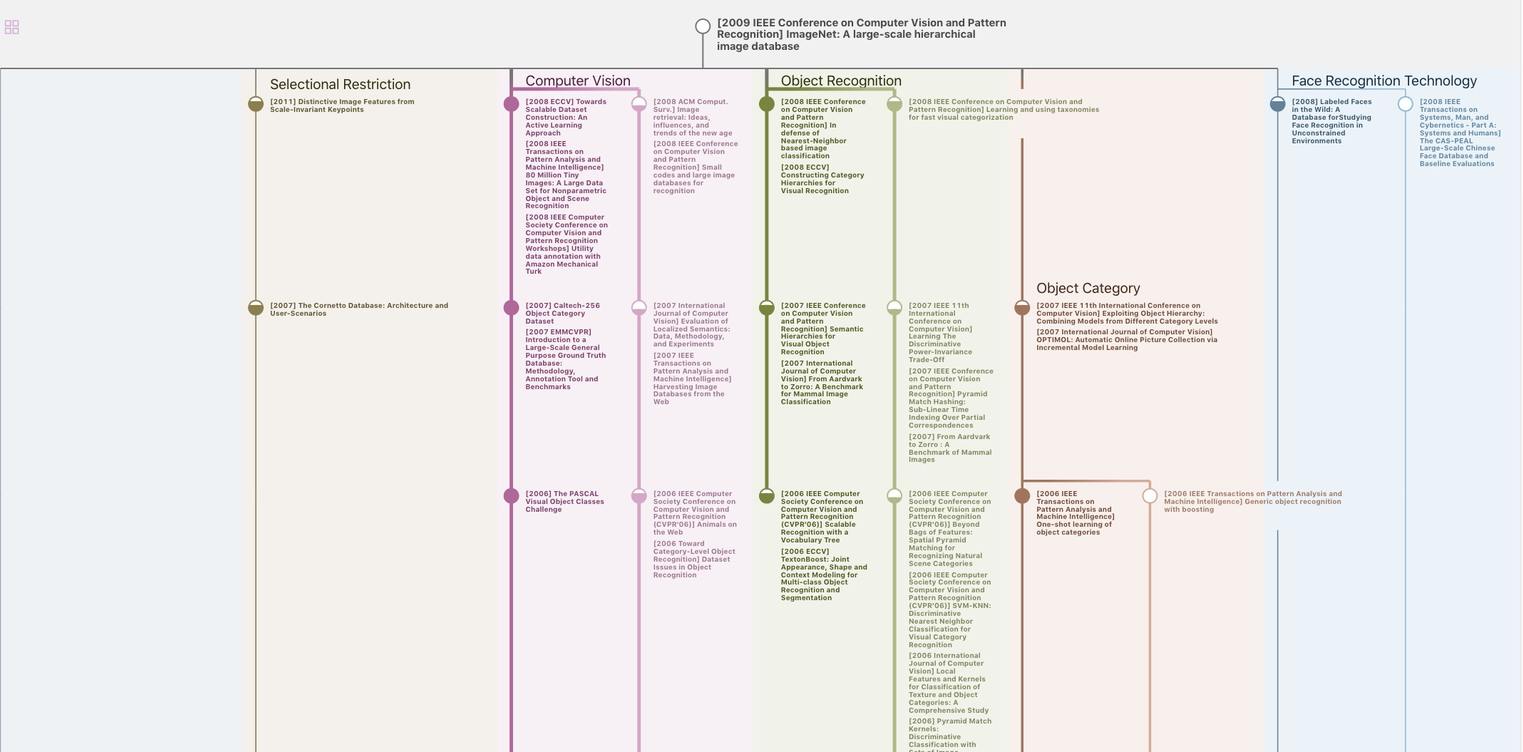
生成溯源树,研究论文发展脉络
Chat Paper
正在生成论文摘要