A Machine Learning Framework Integrating Electronic Health Records for Survival Prognostication in Cervix Cancer
International Journal of Radiation Oncology Biology Physics(2023)
摘要
Recent advances in Machine learning (ML) have led to promising clinical applications in oncology, such as improved detection on imaging, predicting emergency department visits, and predicting survival. The hypothesis of this study is a machine learning model using features extracted from electronic health records can be created to improve individual prognostication in patients with cervix cancer managed with radiotherapy in a binary higher and lower risk group model.This study used a single-institution retrospective dataset. Electronic records of patients treated for cervix cancer with definitive radiotherapy from 2003-2014 were queried. Features were selected for model building were based on relevant clinical variables and ease of abstraction from records: age, race, smoking status, parity, self-reported unintentional weight loss, histology, revised 2018 Fédération Internationale de Gynécologie et d'Obstétrique (FIGO) staging, tumor size, number of enlarged lymph nodes, involved node location, presence of metastases, radiotherapy duration, and concurrent chemotherapy. The dataset was split into training and testing cohorts, with multivariable Cox regression with Lasso regularization performed to predict hazard ratios (HR), and internal 5-fold cross-validation (training set) to determine the regularization parameter. For each patient, a partial HR using all variables was predicted. A cut-off value maximally splitting the training dataset into higher and lower risk cohorts was calculated in the training dataset using Kaplan-Meier estimates and log-rank tests, and this predicted cut-off value was evaluated in the test dataset.A total of 226 patients were included in study, with a median follow-up of 55.1 months; 226 patients in training cohort, and 57 in testing cohort. Feature concordance indices of 0.74 and 0.75 were obtained in the testing and training datasets, respectively, with minimal over-fitting. The three variables that contributed most to the model (with Log HR, 95% CI, p-value) were FIGO stage (0.38, 0.1-0.66, p = 0.01), presence of metastases (0.26, -0.02-0.53, p = 0.07), and unintentional weight loss (-0.34, -0.54-0.14, p<0.01), and FIGO IIB maximally divided the cohort into higher and lower risk stage groupings. A partial HR of 1.15 threshold using all features predicted the largest survival separation between higher and lower risk patients, more so than stage alone (p = 1.18 × 10-3 separated by stage alone vs p = 8.97 × 10-7 separated by partial hazard).A machine learning approach was able to improve prognostication of survival in cervix cancer using additional features from electronic health records. Future work should explore prognostication using large scale datasets and pre-treatment variables for potential incorporation into patient discussions and shared decision making.
更多查看译文
关键词
cervix cancer,survival prognostication,machine learning
AI 理解论文
溯源树
样例
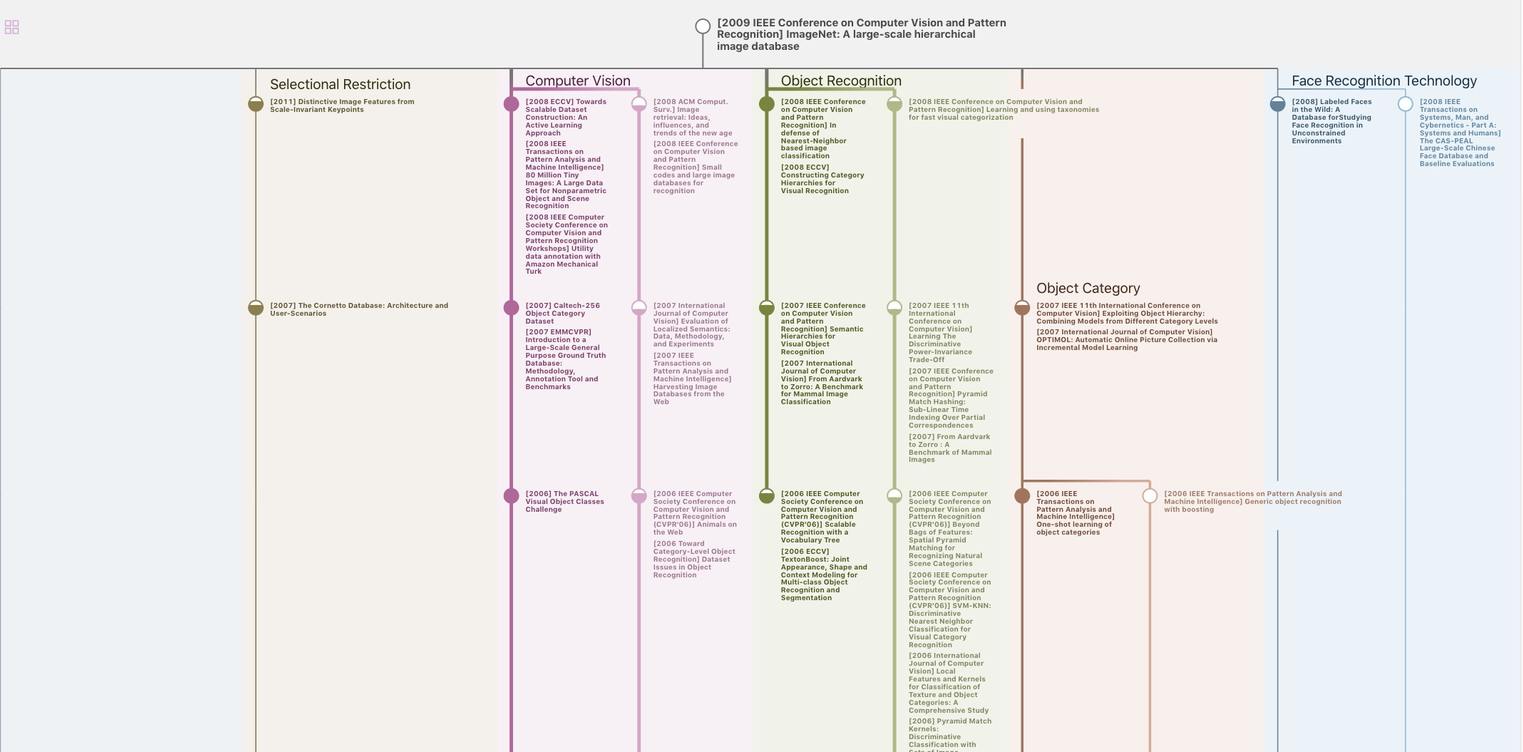
生成溯源树,研究论文发展脉络
Chat Paper
正在生成论文摘要