Lifelike Agility and Play on Quadrupedal Robots using Reinforcement Learning and Generative Pre-trained Models
Research Square (Research Square)(2023)
摘要
Summarizing knowledge from animals and human beings inspires robotic innovations. In this work, we propose a framework for driving legged robots act like real animals with lifelike agility and strategy in complex environments. Inspired by large pre-trained models witnessed with impressive performance in language and image understanding, we introduce the power of advanced deep generative models to produce motor control signals stimulating legged robots to act like real animals. Unlike conventional controllers and end-to-end RL methods that are task-specific, we propose to pre-train generative models over animal motion datasets to preserve expressive knowledge of animal behavior. The pre-trained model holds sufficient primitive-level knowledge yet is environment-agnostic. It is then reused for a successive stage of learning to align with the environments by traversing a number of challenging obstacles that are rarely considered in previous approaches, including creeping through narrow spaces, jumping over hurdles, freerunning over scattered blocks, etc. Finally, a task-specific controller is trained to solve complex downstream tasks by reusing the knowledge from previous stages. Enriching the knowledge regarding each stage does not affect the usage of other levels of knowledge. This flexible framework offers the possibility of continual knowledge accumulation at different levels. We successfully apply the trained multi-level controllers to the MAX robot, a quadrupedal robot developed in-house, to mimic animals, traverse complex obstacles, and play in a designed challenging multi-agent Chase Tag Game, where lifelike agility and strategy emerge on the robots. The present research pushes the frontier of robot control with new insights on reusing multi-level pre-trained knowledge and solving highly complex downstream tasks in the real world.
更多查看译文
关键词
quadrupedal robots,reinforcement
AI 理解论文
溯源树
样例
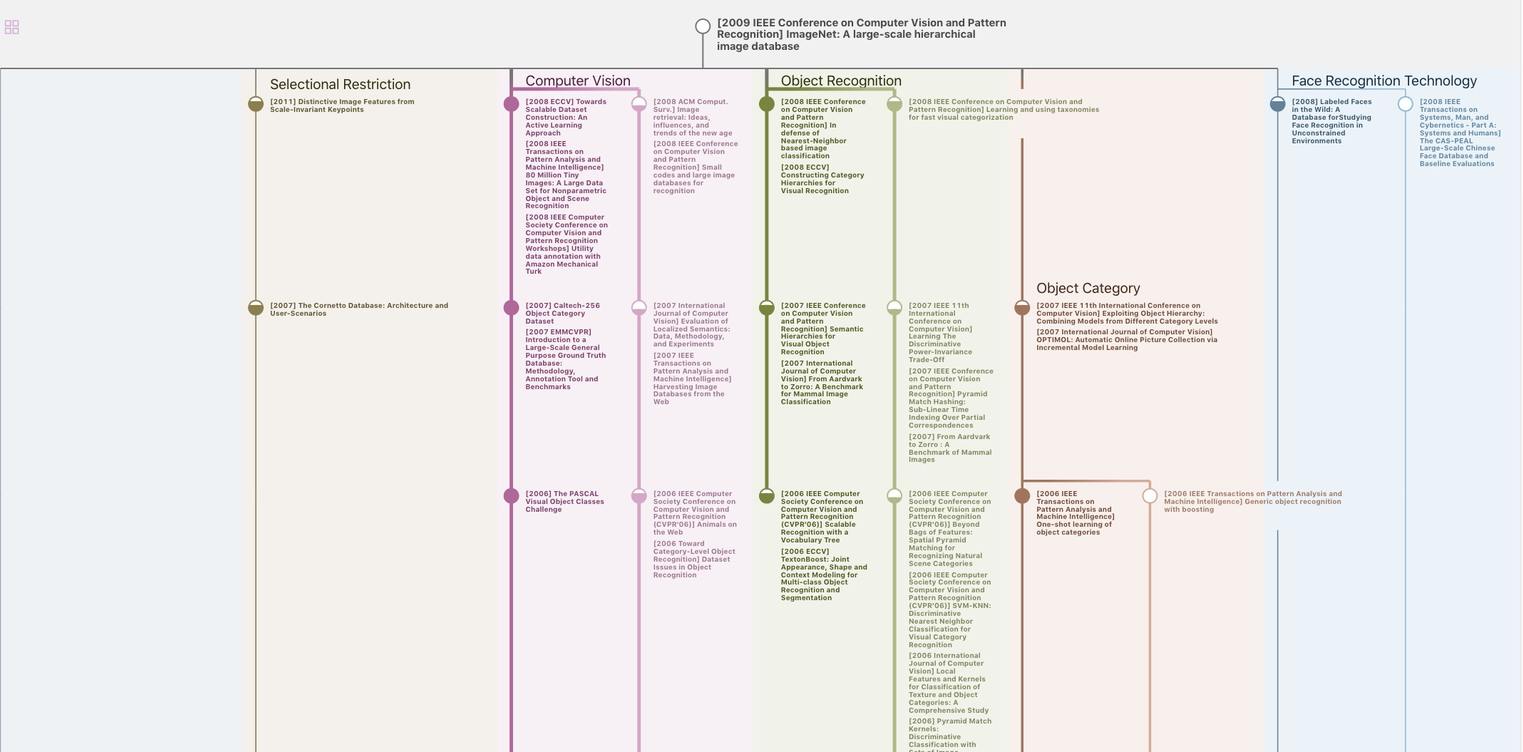
生成溯源树,研究论文发展脉络
Chat Paper
正在生成论文摘要