Improving the Expected Goal Value in Football Using Multilayer Perceptron Networks
Communications in computer and information science(2023)
摘要
The development of big data and machine learning is having a special boost in the field of innovation in sports. Football is the most popular sport in Europe with millions of players and billions of euros invested. Currently, machine learning applications in football are focused on video analysis to events detection (tracking of players, statistics matches, scouting, ...), injuries evaluation and prediction, among others. The xG metric (Expected Goals) determines the probability that a shot will result in a goal and is often displayed on screen during the most important football matches in the world. In this paper we present a new model to obtain more accurate predictions of the probability of a shot becoming a goal. The model is based on a multi-layer perceptron neural network (MLP), which allows the interaction of different variables and improves the model’s performance. Our proposal includes an evaluation of the quality and a comparison with the xG provided by statsbomb, one of the most important football data providers of the world. The results show that our model outperforms the quality of the expectations provided by statsbomb. Specifically, it is clearly better in their capability to detect actual positive cases (goals).
更多查看译文
关键词
goal value,football,networks
AI 理解论文
溯源树
样例
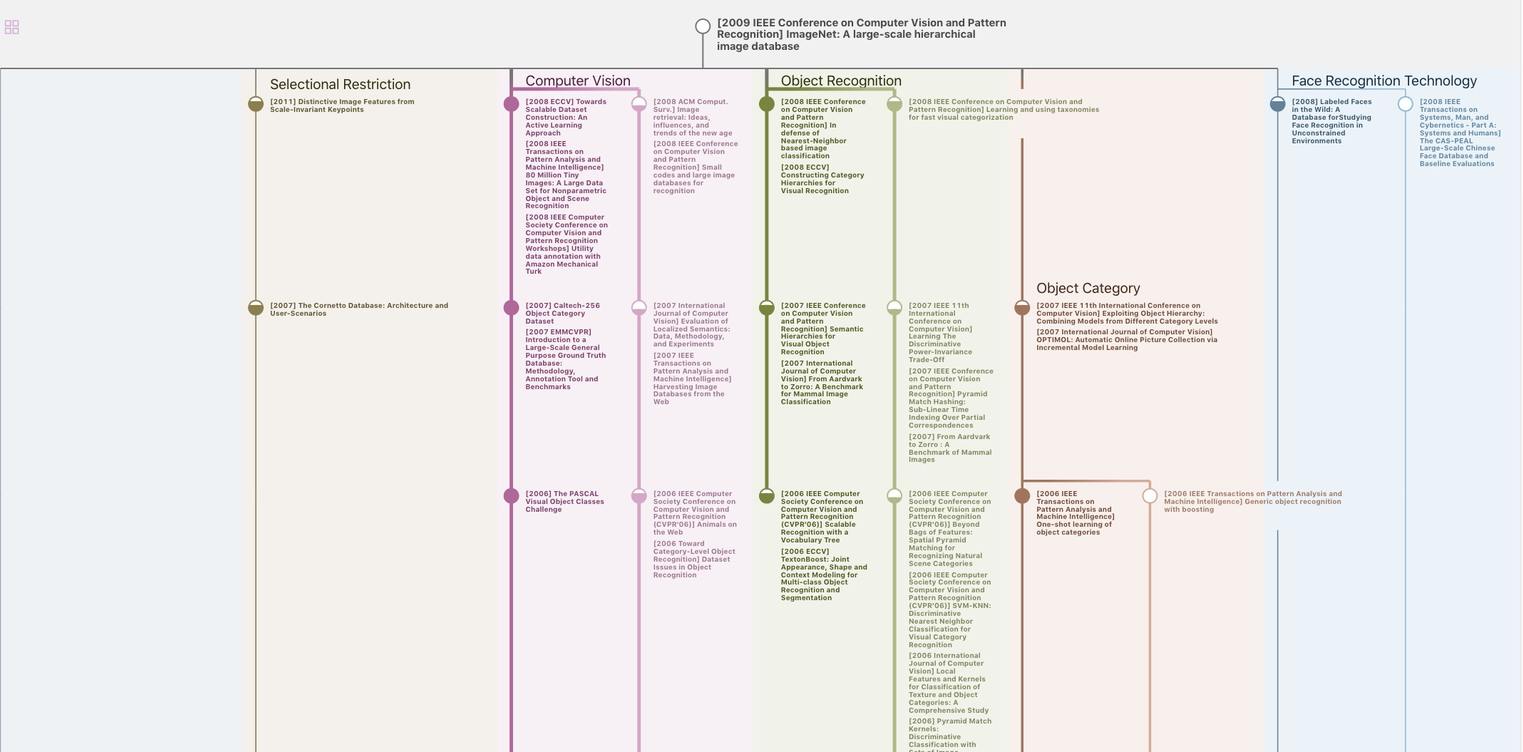
生成溯源树,研究论文发展脉络
Chat Paper
正在生成论文摘要