Variable-Length Differential Evolution for Numerical and Discrete Association Rule Mining
IEEE ACCESS(2024)
摘要
This paper proposes a variable-length Differential Evolution for Association Rule Mining. The proposed algorithm includes a novel representation of individuals, which can encode both numerical and discrete attributes in their original or absolute complement of the original intervals. The fitness function used is comprised of a weighted sum of Support and Confidence Association Rule Mining metrics. The proposed algorithm was tested on fourteen publicly available, and commonly used datasets from the UC Irvine Machine Learning Repository. It is also compared to the nature inspired algorithms taken from the NiaARM framework, providing superior results. The implementation of the proposed algorithm follows the principles of Green Artificial Intelligence, where a smaller computational load is required for obtaining promising results, and thus lowering the carbon footprint.
更多查看译文
关键词
Data mining,Genetic algorithms,Artificial intelligence,Statistics,Sociology,Measurement,Indexes,Association rule mining,differential evolution,data mining,variable-length solution representation,green AI
AI 理解论文
溯源树
样例
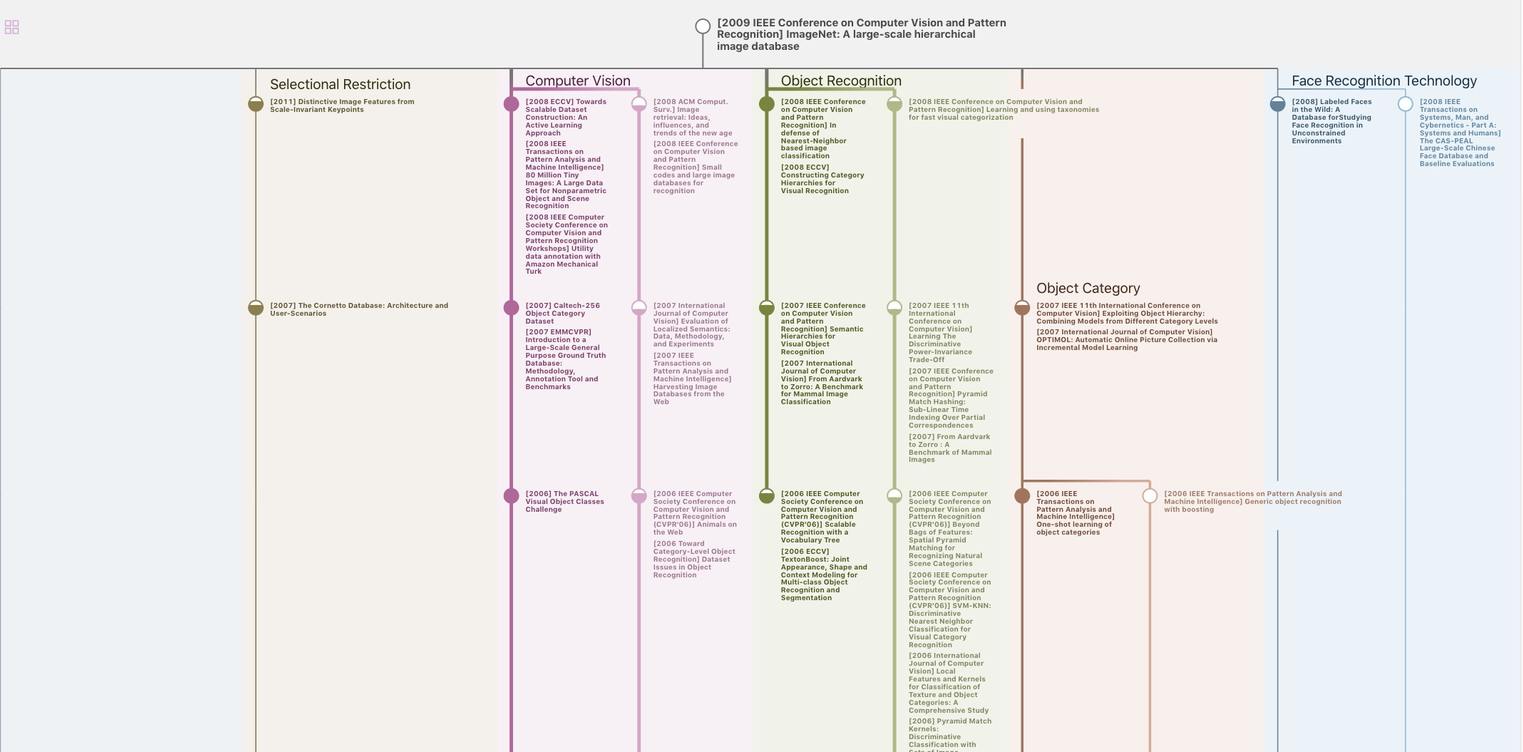
生成溯源树,研究论文发展脉络
Chat Paper
正在生成论文摘要