Root and community inference on latent network growth processes using noisy attachment models
Journal of The Royal Statistical Society Series B-statistical Methodology(2023)
摘要
Abstract Many existing statistical models for networks overlook the fact that most real-world networks are formed through a growth process. To address this, we introduce the PAPER (Preferential Attachment Plus Erdős-Rényi) model for random networks, where we let a random network G be the union of a preferential attachment (PA) tree T and additional Erdős-Rényi) (ER) random edges. The PA tree component captures the underlying growth/recruitment process of a network where vertices and edges are added sequentially, while the ER component can be regarded as random noise. Given only a single snapshot of the final network G, we study the problem of constructing confidence sets for the early history, in particular the root node, of the unobserved growth process; the root node can be patient zero in a disease infection network or the source of fake news in a social media network. We propose an inference algorithm based on Gibbs sampling that scales to networks with millions of nodes and provide theoretical analysis showing that the expected size of the confidence set is small so long as the noise level of the ER edges is not too large. We also propose variations of the model in which multiple growth processes occur simultaneously, reecting the growth of multiple communities, and we use these models to provide a new approach to community detection.
更多查看译文
关键词
latent network growth processes,community inference,noisy attachment models
AI 理解论文
溯源树
样例
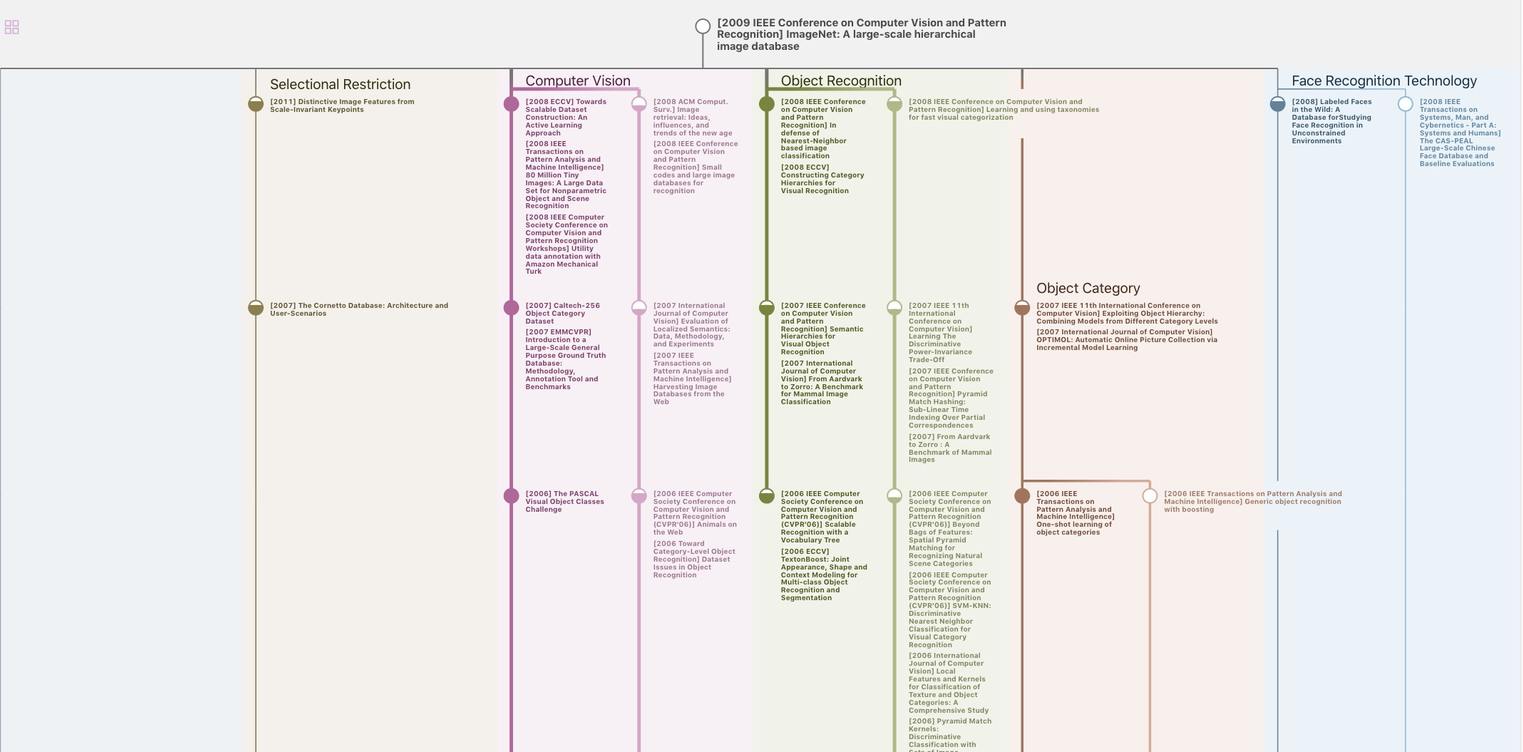
生成溯源树,研究论文发展脉络
Chat Paper
正在生成论文摘要