Selective Multi-Source Domain Adaptation Network for Cross-Subject Motor Imagery Discrimination
IEEE Transactions on Cognitive and Developmental Systems(2023)
摘要
Discriminating motor imagery with electroencephalogram (EEG)-based brain-computer interface (BCI) poses a challenge as it involves an extensive data acquisition phase that demands a substantial amount of effort from the user. To address this issue, one approach is to use unsupervised domain adaptation, where classification models are constructed using data from multiple subjects, and only the unlabeled data from the target user is used for model calibration. However, since brain patterns from motor imagery vary between individuals, the reliability of each subject must be considered when multiple subjects are used to build the classification model. Thus in this paper, we propose Selective-MDA that performs domain adaptation on each source subject and selectively limits influences based on their domain discrepancies. To evaluate our approach, we assess our results with two public datasets, BCI Competition IV IIa and the Autocalibration and Recurrent Adaptation datasets. We further investigate the effect of source selection by comparing the discrimination performance when different numbers of source domains are selected based on discrepancy measures. Our results demonstrate that Selective-MDA not only integrates multi-source domain adaptation to cross-subject motor imagery discrimination but also highlights the impact of source domain selection when using data from multiple subjects for model training.
更多查看译文
关键词
brain-computer interface,unsupervised domain adaptation,motor imagery,electroencephalography,neural decoding
AI 理解论文
溯源树
样例
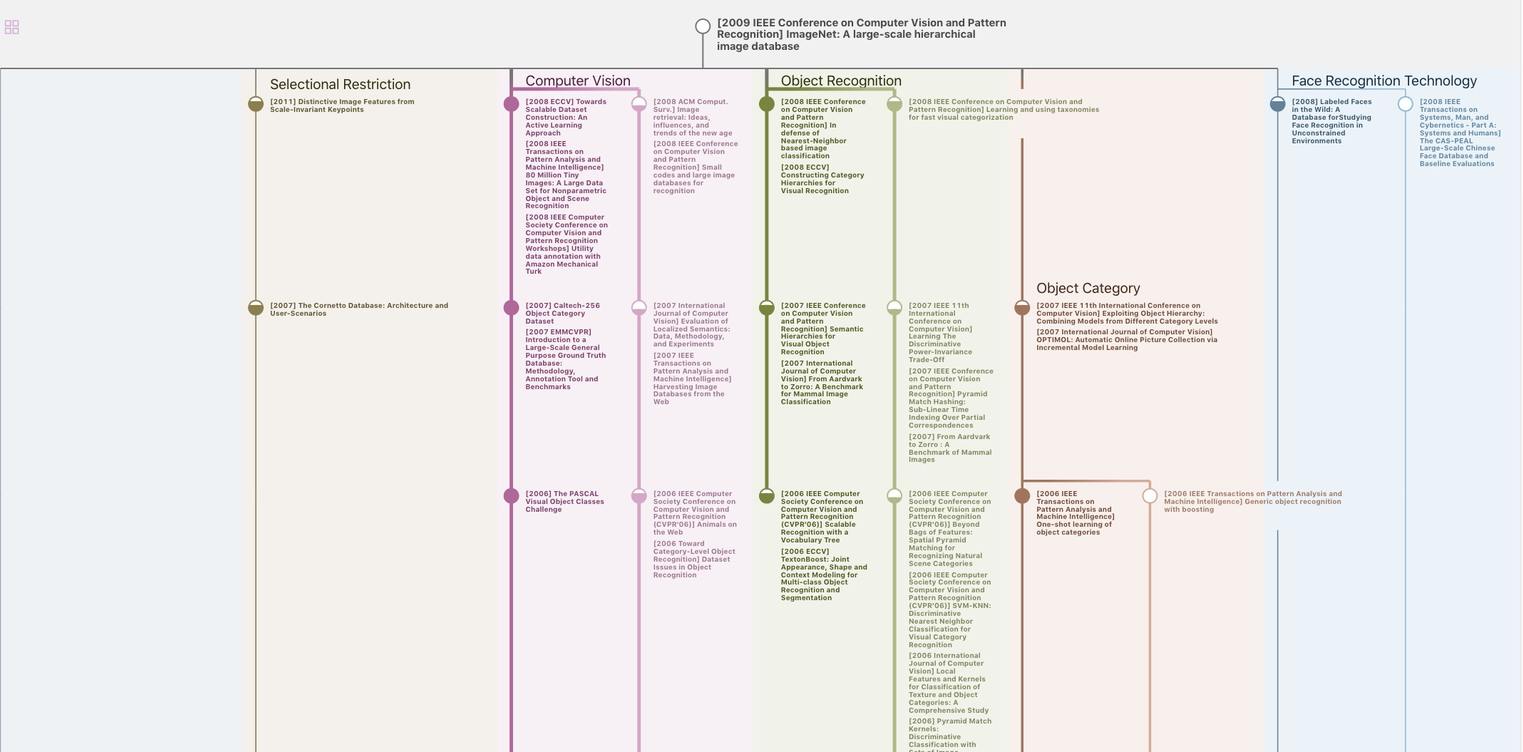
生成溯源树,研究论文发展脉络
Chat Paper
正在生成论文摘要