Bilateral Knowledge Interaction Network for Referring Image Segmentation.
IEEE Trans. Multim.(2024)
摘要
Referring image segmentation aims to segment objects that are described by natural language expressions. Although remarkable advancements have been made to align natural language expressions with visual representations for better performance, the interaction between image-level and text-level information is still not formulated properly. Most of the previous works focus on building correlations between vision and language, ignoring the variety of objects. The target objects with unique appearances may not be correctly located or completely segmented. In this paper, we propose a novel Bilateral Knowledge Interaction Network, termed BKINet, which reformulates the image-text interaction in a bilateral manner to adapt concrete knowledge of the target object in the image. BKINet contains two key components: a knowledge learning module (KLM) and a knowledge applying module (KAM). In the KLM, the abstract knowledge from text features is replenished with concrete knowledge from visual features to adapt to the target objects in the input images, which generates the knowledge interaction kernels (KI kernels) containing abundant referring information. With the referring information of KI kernels, the KAM is designed to highlight the most relevant visual features for predicting the accurate segmentation mask. Extensive experiments on three widely-used datasets,
i.e.
RefCOCO, RefCOCO+, and G-ref, demonstrate the superiority of BKINet over the state-of-the-art. Our code is released at
https://github.com/dhding/BKINet.
更多查看译文
关键词
referring image segmentation,vision-language
AI 理解论文
溯源树
样例
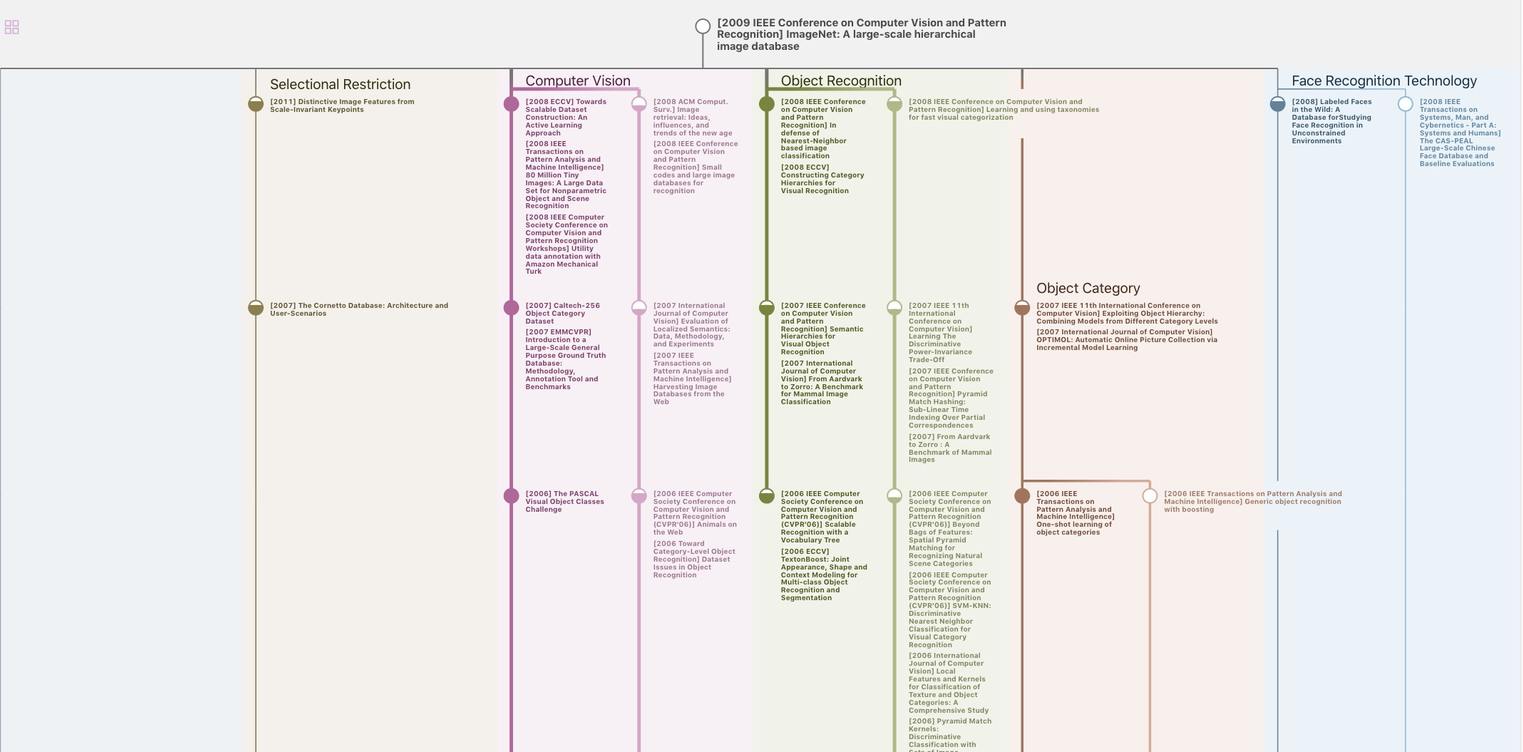
生成溯源树,研究论文发展脉络
Chat Paper
正在生成论文摘要