Machine learning technique unraveled subspecies-specific ionomic variation with the preferential mineral enrichment in rice
Cereal Chemistry(2023)
摘要
Background and ObjectivesTo enrich micronutrients in rice through breeding and to identify biofortified donor lines, screening a large diversity panel of major subspecies of rice accessions for their ionomes is necessary.FindingsInductively coupled plasma optical emission spectroscopy was deployed to profile grain ionomes from 1100 rice accessions. Classification models derived for multidimensional ionomics data using a random forest model, support vector machine, and artificial neural network predicted three distinct groups that differ in ionomic levels with higher prediction accuracy (76.8%-85.1%). While class A showed inferior lines in mineral content, class B and C represent superior lines with enriched multiple minerals. The identified contrasting lines from the modeling classifications were milled and profiled for minerals and toxic elements using inductively coupled plasma mass spectrometry and revealed donors with an elevated mineral content even higher than the Zn-biofortified rice lines.ConclusionOverall, the result of this study provided new insights into ionomic variation among subspecies with a preferential enrichment of minerals in temperate japonica and admixed populations coincided with increased grain protein content, while in indica median mineral content is low while selected for increased amylose content.Significance and NoveltyArtificial intelligence models were developed to predict grains enriched with multiple minerals.
更多查看译文
关键词
grain quality,ionome,machine learning,micronutrients,modeling,rice diversity
AI 理解论文
溯源树
样例
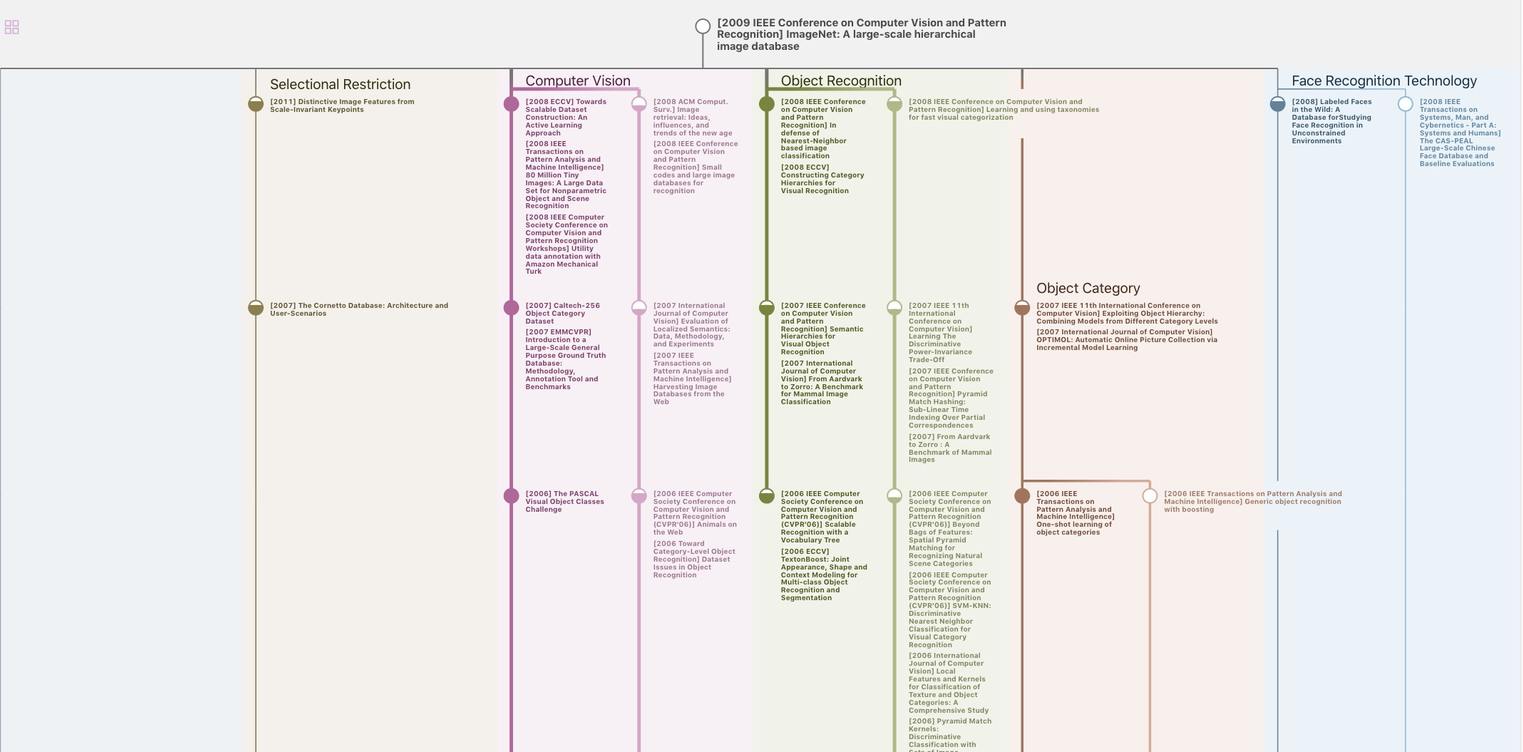
生成溯源树,研究论文发展脉络
Chat Paper
正在生成论文摘要