Deep reinforcement learning optimized double exponentially weighted moving average controller for chemical mechanical polishing processes
CHEMICAL ENGINEERING RESEARCH & DESIGN(2023)
摘要
This study investigates a deep reinforcement learning (DRL)-assisted double exponentially weighted moving average (dEWMA) controller for run-to-run (RtR) control in the semiconductor manufacturing process. We focus on implementing parameter adaption of dEWMA controllers to achieve disturbance compensation and target tracking. Owing to the powerful adaptive decision-making capability of the DRL, the weight adjustment of dEWMA controller is formulated as a Markov decision process. Specifically, the DRL behaves as an assisted controller to derive appropriate weights that facilitate dEWMA to perform highly accurate disturbance estimation, whereas the standard dEWMA works as a baseline controller to provide suitable recipes for the manufacturing process. Consequently, a composite control strategy integrating DRL and dEWMA is developed. In addition, a twin-delayed deep deterministic policy gradient algorithm is employed to adjust the weights of dEWMA online. The effectiveness of the proposed scheme is validated in a chemical mechanical polishing process. Several disturbance rejection scenarios verify the benefits of the suggested approach.(c) 2023 Institution of Chemical Engineers. Published by Elsevier Ltd. All rights reserved.
更多查看译文
关键词
Deep reinforcement learning,Run-to-run control,Double exponentially weighted,moving average,Chemical mechanical polishing,Parameter optimization
AI 理解论文
溯源树
样例
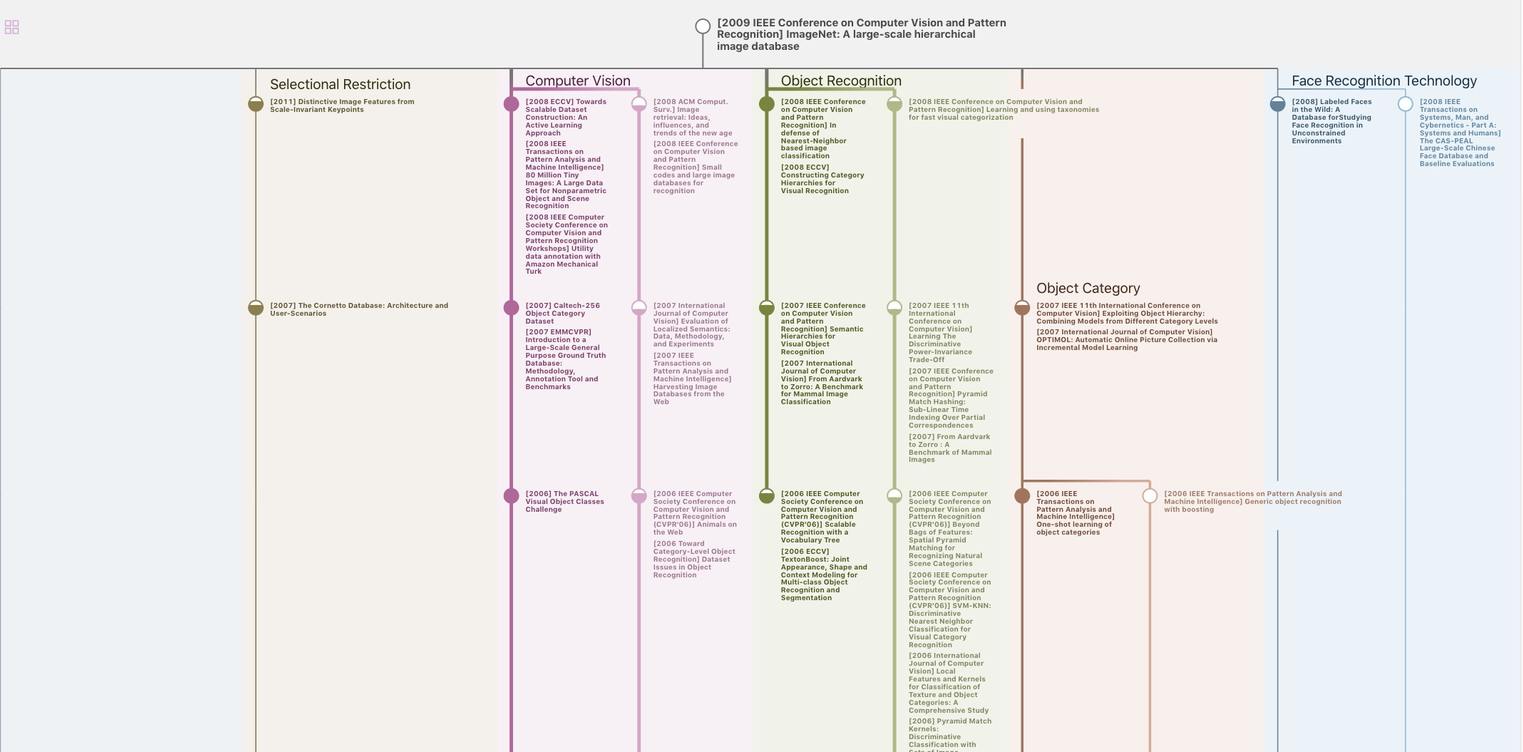
生成溯源树,研究论文发展脉络
Chat Paper
正在生成论文摘要