Bagging and Boosting Fine-Tuning for Ensemble Learning.
IEEE Trans. Artif. Intell.(2024)
摘要
Ensemble learning aggregates outputs from multiple base learners for better performance. Bootstrap aggregating (Bagging) and boosting are two popular such approaches. They are suitable for integrating unstable base learners with large variance and weak base learners with large bias, respectively, but not base learners with small variance and/or bias, e.g., support vector machine, regularized logistic regression and ridge regression. This paper proposes two novel ensemble-learning-based fine-tuning approaches, boosting fine-tuning (BF) and Bagging and boosting fine-tuning (BBF), to fine-tune learners with small variance and/or bias for better performance. BF embeds boosting in a single hidden layer neural network. In each iteration, BF first uses Newton's method to generate a temporary training set, and then trains a boosting learner on it. BBF combines BF and Bagging. It first uses bootstrap to obtain multiple replicas of the training set, and then trains a BF learner on each replica. Extensive experiments on 46 real world datasets demonstrated that BBF is flexible, robust and effective, and can fine-tune many popular classifiers to achieve better generalization performance.
更多查看译文
关键词
Bagging,boosting,broad learning system,en-semble learning,fine-tuning
AI 理解论文
溯源树
样例
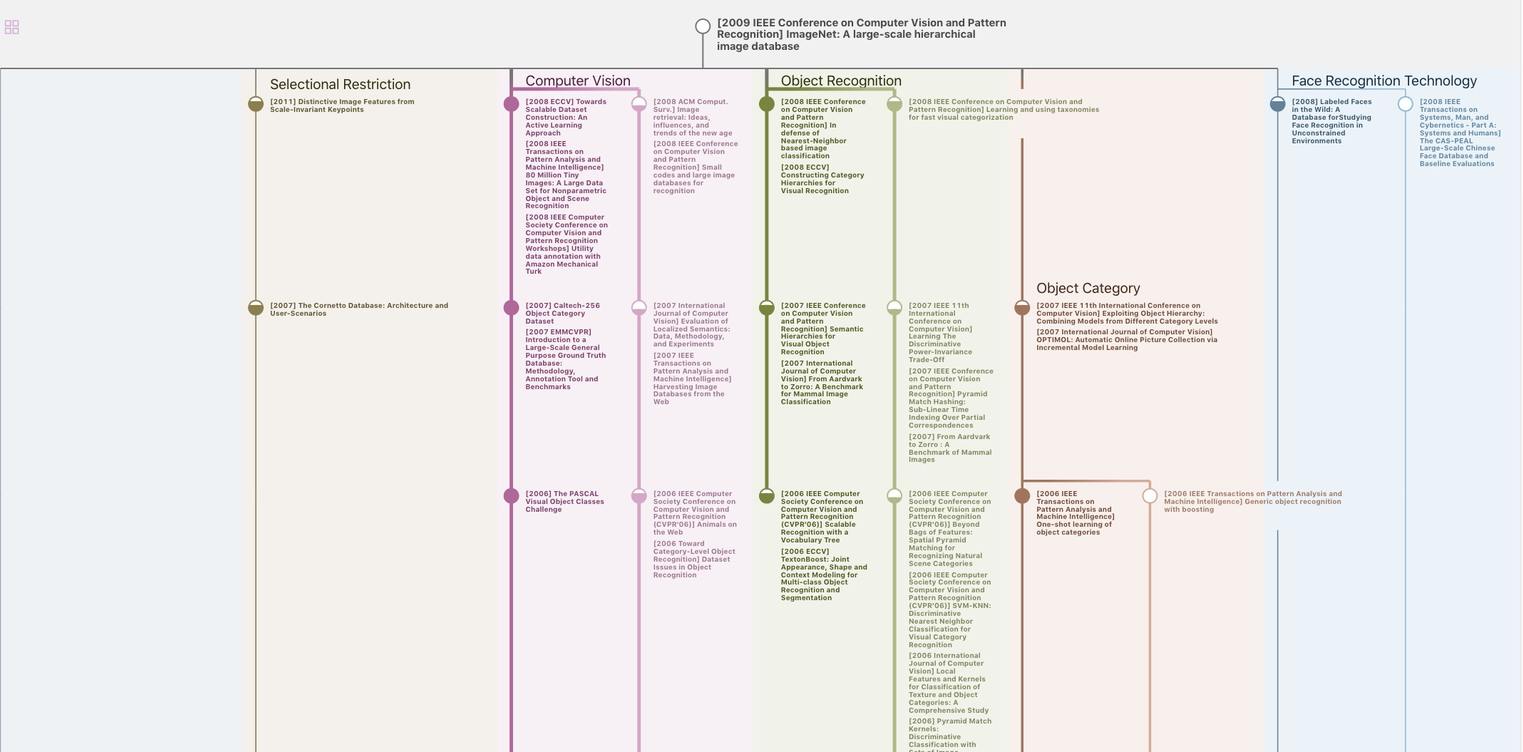
生成溯源树,研究论文发展脉络
Chat Paper
正在生成论文摘要