Textual Enhanced Adaptive Meta-Fusion for Few-Shot Visual Recognition.
IEEE Trans. Multim.(2024)
摘要
Few-shot learning (FSL) is a challenging task that aims to train a classifier to recognize novel categories, where only a few annotated examples are available in each category. Recently, many FSL approaches have been proposed based on the meta-learning paradigm, which attempts to learn transferable knowledge from similar tasks by designing a meta-learner. However, most of these approaches only exploit the information from visual modality and do not utilize ones from additional modalities (
e.g.
, textual description). Since the labeled examples in FSL are limited, increasing the information on the examples is a probable solution to improve the classification performance. This motivates us to propose a novel meta-learning method, termed textual enhanced adaptive meta-fusion FSL (TAMF-FSL), which leverages both the visual information from the visual image and semantic information from language supervision. Specifically, TAMF-FSL exploits the semantic information of textual description to improve the visual-based models. We first employ a text encoder to learn the semantic features of each visual category, and then design a modality alignment module and meta-fusion module to align and fuse the visual and semantic features for final prediction. Extensive experiments show that the proposed method outperforms many recent or competitive FSL counterparts on two popular datasets.
更多查看译文
关键词
Few-shot visual recognition,semantic information,multimodal fusion
AI 理解论文
溯源树
样例
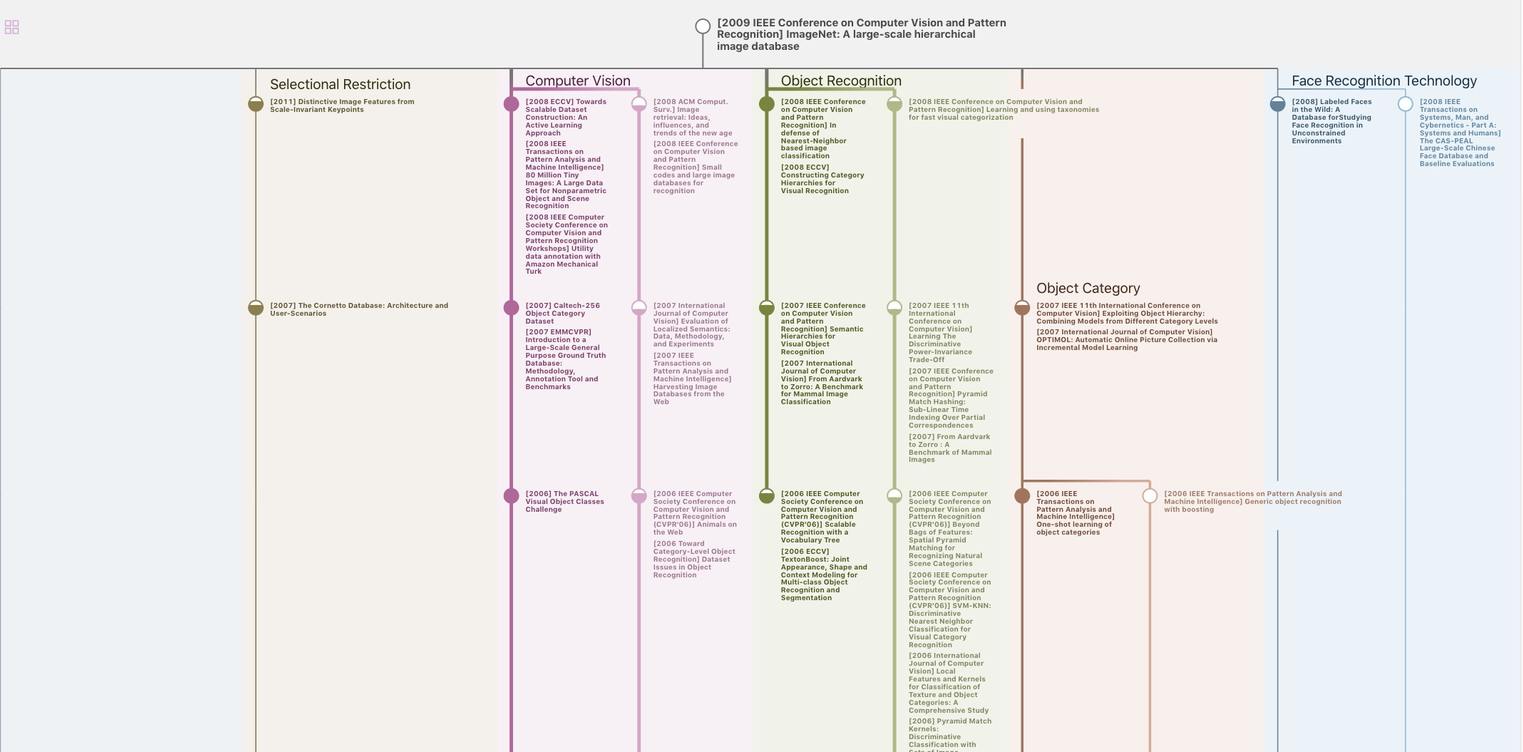
生成溯源树,研究论文发展脉络
Chat Paper
正在生成论文摘要