DeepDefense: A Steganalysis-Based Backdoor Detecting and Mitigating Protocol in Deep Neural Networks for AI Security
Security and Communication Networks(2023)
摘要
Backdoor attacks have been recognized as a major AI security threat in deep neural networks (DNNs) recently. The attackers inject backdoors into DNNs during the model training such as federated learning. The infected model behaves normally on the clean samples in AI applications while the backdoors are only activated by the predefined triggers and resulted in the specified results. Most of the existing defensing approaches assume that the trigger settings on different poisoned samples are visible and identical just like a white square in the corner of the image. Besides, the sample-specific triggers are always invisible and difficult to detect in DNNs, which also becomes a great challenge against the existing defensing protocols. In this paper, to address the above problems, we propose a backdoor detecting and mitigating protocol based on a wider separate-then-reunion network (WISERNet) equipped with a cryptographic deep steganalyzer for color images, which detects the backdoors hiding behind the poisoned samples even if the embedding algorithm is unknown and further feeds the poisoned samples into the infected model for backdoor unlearning and mitigation. The experimental results show that our work performs better in the backdoor defensing effect compared to state-of-the-art backdoor defensing methods such as fine-pruning and ABL against three typical backdoor attacks. Our protocol reduces the attack success rate close to 0% on the test data and slightly decreases the classification accuracy on the clean samples within 3%.
更多查看译文
关键词
ai security,backdoor detecting,deepdefense neural networks,steganalysis-based
AI 理解论文
溯源树
样例
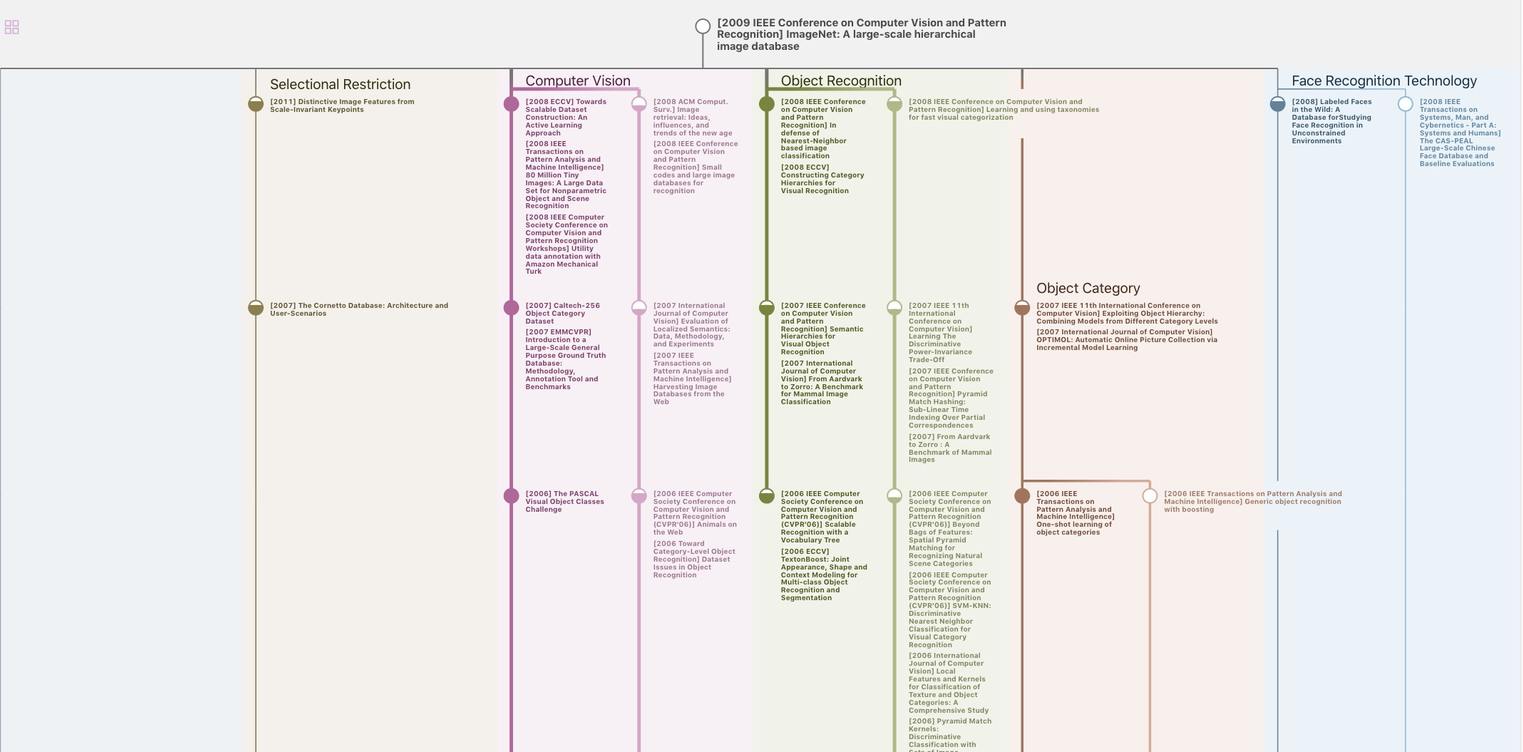
生成溯源树,研究论文发展脉络
Chat Paper
正在生成论文摘要