Tuning Bayesian optimization for materials synthesis: simulating two- and three-dimensional cases
Science and Technology of Advanced Materials: Methods(2023)
摘要
Compared to the optimization of a 1D synthesis parameter in materials synthesis, the optimization of multi-dimensional synthesis parameters is challenging for researchers. Bayesian optimization (BO) has shown high performance in optimizing high-dimensional synthesis parameters when appropriate hyperparameters are adopted. However, hyperparameter tuning for the kernel and acquisition functions used in BO is yet to be fully discussed by material researchers. In this study, we simulated materials synthesis under 2D and 3D synthesis conditions using artificial model functions with different process windows to investigate the effects of hyperparameters. The assumed parameters were temperature, oxygen partial pressure, and the sputtering power for thin-film deposition. Our findings indicate that estimating the process window and the range of physical property change based on the experience and knowledge of the materials researcher is crucial for tuning the hyperparameters of the kernel function. The simulations for high-dimensional search spaces case also indicate that the number of trials for optimization of synthesis conditions might reach several hundred or more. Therefore, the dimensionality and range of the search space must be limited based on the number of practical experiments, which is crucial for applying Bayesian optimization to materials synthesis. Our results facilitate fully automated and autonomous materials synthesis using BO and robotics for materials exploration in a high-dimensional search space.
更多查看译文
关键词
bayesian optimization,materials synthesis,three-dimensional
AI 理解论文
溯源树
样例
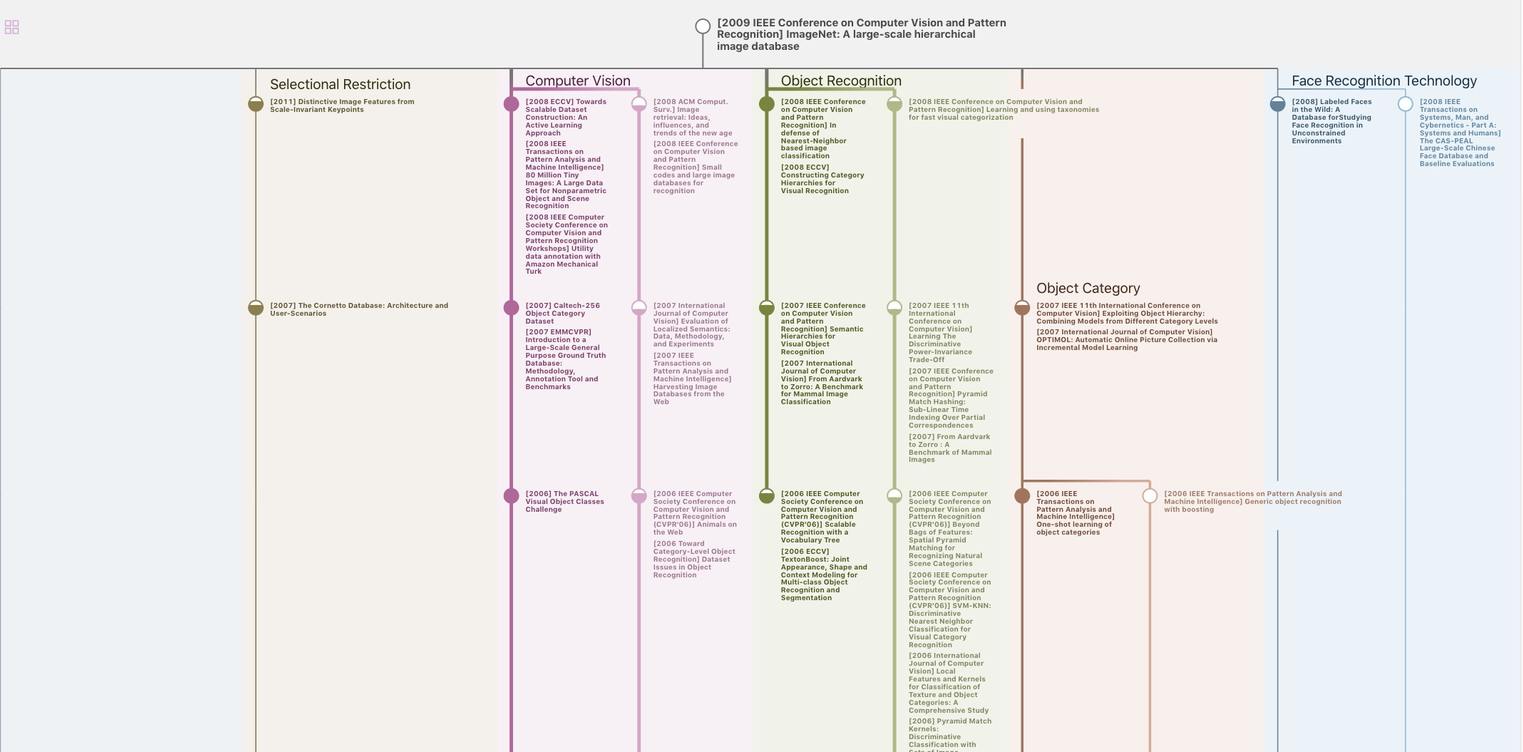
生成溯源树,研究论文发展脉络
Chat Paper
正在生成论文摘要