Re-Benchmarking Pool-Based Active Learning for Binary Classification
arXiv (Cornell University)(2023)
摘要
Active learning is a paradigm that significantly enhances the performance of machine learning models when acquiring labeled data is expensive. While several benchmarks exist for evaluating active learning strategies, their findings exhibit some misalignment. This discrepancy motivates us to develop a transparent and reproducible benchmark for the community. Our efforts result in an open-sourced implementation (https://github.com/ariapoy/active-learning-benchmark) that is reliable and extensible for future research. By conducting thorough re-benchmarking experiments, we have not only rectified misconfigurations in existing benchmark but also shed light on the under-explored issue of model compatibility, which directly causes the observed discrepancy. Resolving the discrepancy reassures that the uncertainty sampling strategy of active learning remains an effective and preferred choice for most datasets. Our experience highlights the importance of dedicating research efforts towards re-benchmarking existing benchmarks to produce more credible results and gain deeper insights.
更多查看译文
关键词
active learning,classification,re-benchmarking,pool-based
AI 理解论文
溯源树
样例
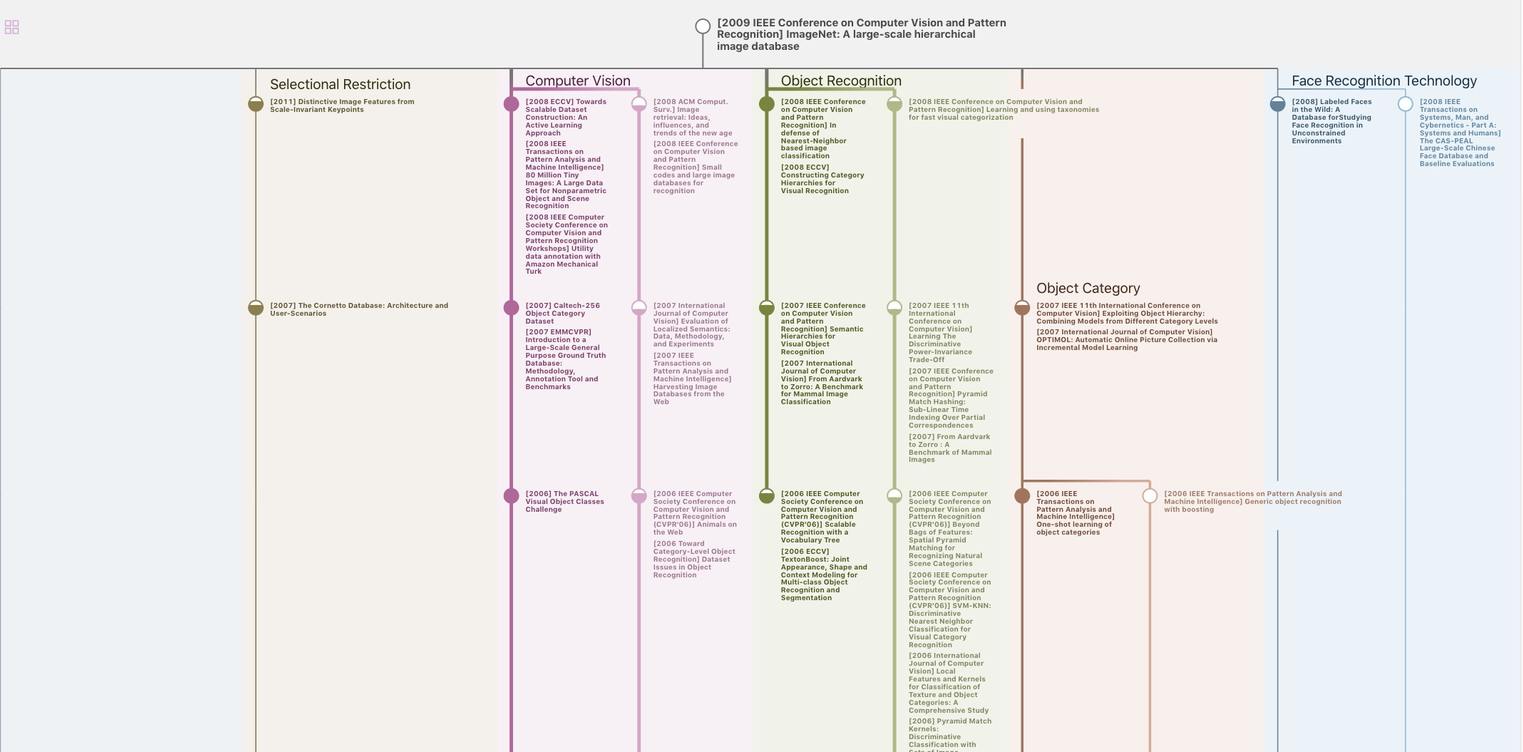
生成溯源树,研究论文发展脉络
Chat Paper
正在生成论文摘要