Learning Proposal-Aware Re-Ranking for Weakly-Supervised Temporal Action Localization
IEEE TRANSACTIONS ON CIRCUITS AND SYSTEMS FOR VIDEO TECHNOLOGY(2024)
摘要
Weakly-supervised temporal action localization (WTAL) aims to localize and classify action instances in untrimmed videos with only video-level labels available. Despite the remarkable success of existing methods, whose generated proposals are commonly far more than the ground-truth action instances, it still makes sense to improve the ranking accuracy of the generated proposals since users in real-world scenarios usually prioritize the action proposals with the highest confidence scores. The inaccuracy of the proposal ranking mainly comes from two aspects: For one thing, the traditional proposal generation manner entirely relies on snippet-level perception, resulting in a significant yet unnoticed gap with the target of proposal-level localization. For another, existing methods commonly employ a hand-crafted proposal generation manner, a post-process that does not participate in model optimization. To address the above issues, we propose an end-to-end trained two-stage method, termed as Learning Proposal-aware Re-ranking (LPR) for WTAL. In the first stage, we design a proposal-aware feature learning module to inject the proposal-aware contextual information into each snippet, and then the enhanced features are utilized for predicting initial proposals. Furthermore, to perform effective and efficient proposal re-ranking, in the second stage, we contrast the proposals attached with high confidence scores with our constructed multi-scale foreground/background prototypes for further optimization. Evaluated by both the vanilla and Top- $k$ mAP metrics, results of extensive experiments on two popular benchmarks demonstrate the effectiveness of our proposed method.
更多查看译文
关键词
Proposals,Feature extraction,Location awareness,Videos,Measurement,Task analysis,Optimization,weakly-supervised temporal action localization,Proposal-aware reranking
AI 理解论文
溯源树
样例
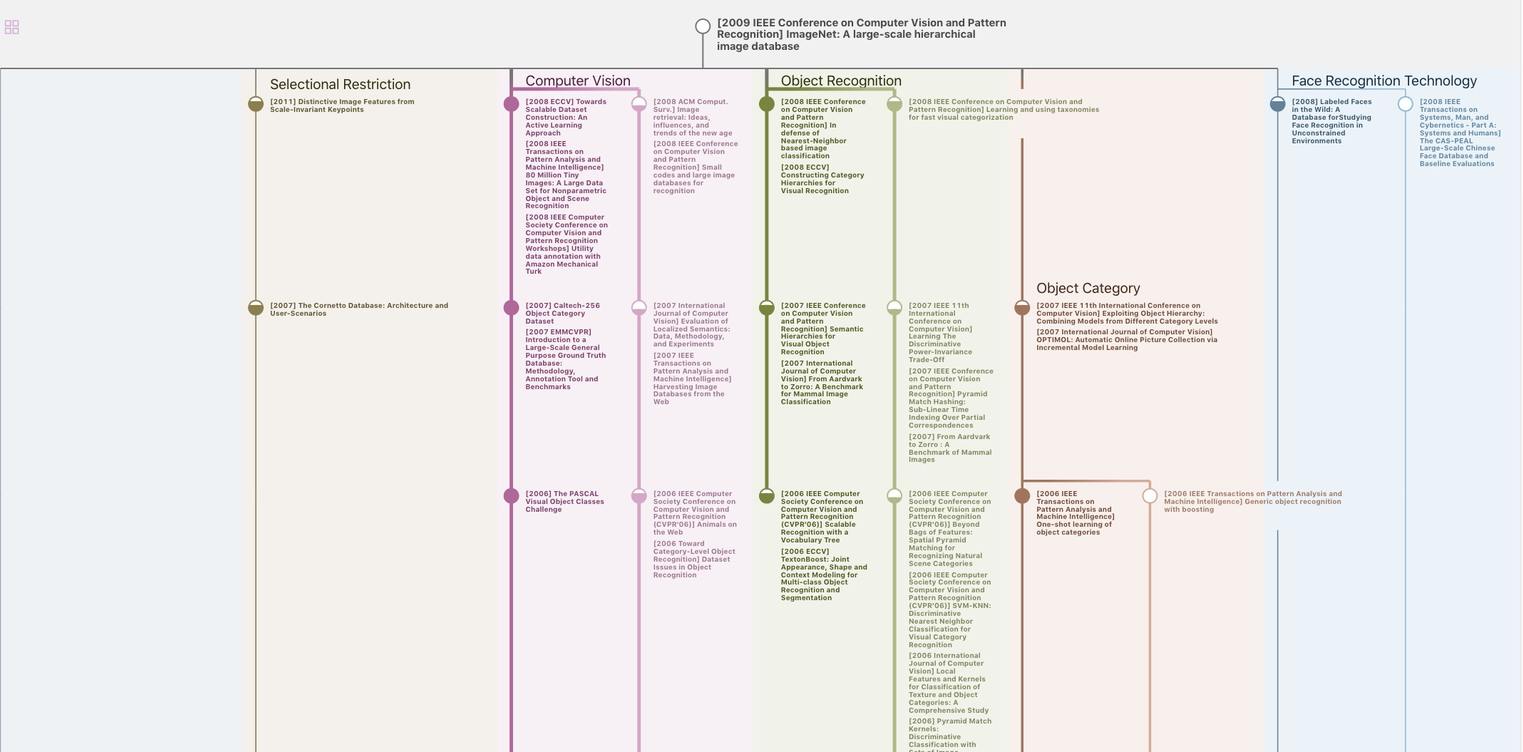
生成溯源树,研究论文发展脉络
Chat Paper
正在生成论文摘要