M3KE: A Massive Multi-Level Multi-Subject Knowledge Evaluation Benchmark for Chinese Large Language Models
arXiv (Cornell University)(2023)
摘要
Large language models have recently made tremendous progress in a variety of aspects, e.g., cross-task generalization, instruction following. Comprehensively evaluating the capability of large language models in multiple tasks is of great importance. In this paper, we propose M3KE, a Massive Multi-Level Multi-Subject Knowledge Evaluation benchmark, which is developed to measure knowledge acquired by Chinese large language models by testing their multitask accuracy in zero- and few-shot settings. We have collected 20,477 questions from 71 tasks. Our selection covers all major levels of Chinese education system, ranging from the primary school to college, as well as a wide variety of subjects, including humanities, history, politics, law, education, psychology, science, technology, art and religion. All questions are multiple-choice questions with four options, hence guaranteeing a standardized and unified assessment process. We've assessed a number of state-of-the-art open-source Chinese large language models on the proposed benchmark. The size of these models varies from 335M to 130B parameters. Experiment results demonstrate that they perform significantly worse than GPT-3.5 that reaches an accuracy of ~ 48% on M3KE. The dataset is available at https://github.com/tjunlp-lab/M3KE.
更多查看译文
关键词
chinese large language models,knowledge,multi-level,multi-subject
AI 理解论文
溯源树
样例
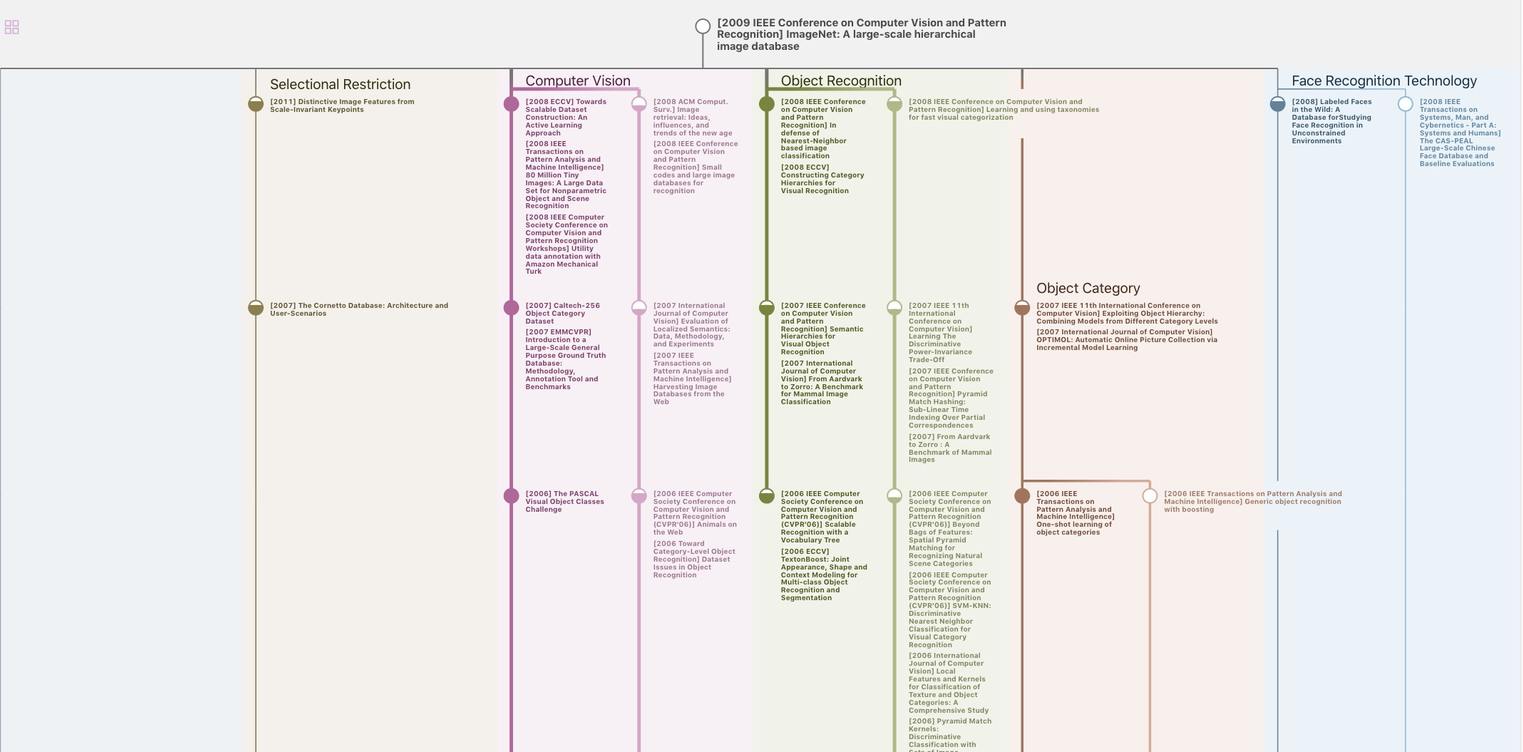
生成溯源树,研究论文发展脉络
Chat Paper
正在生成论文摘要