Tackling bias in the data for breast cancer prediction using machine learning-based decision support
Biostatistics & Epidemiology(2023)
摘要
In this study, a machine learning (ML)-based decision support approach was developed to identify breast cancer likelihood in patients, based on their background and physiological data. Two ML models, Naïve Bayes and Logistic Regression were used to evaluate the Breast Cancer Surveillance Consortium dataset that had about 9:1 ratio of non-cancer cases (‘Class 0’) to cancer cases (‘Class 1’). We manually built both balanced and unbalanced training datasets and a non-overlapping testing dataset using a stratified sampling method. For each model, we partitioned the prediction results on testing set into two groups, the ‘Agree’ group included cases where balanced and unbalanced ML predictions agreed, and the remaining cases come under ‘Disagree’ group. Sensitivity and Positive Predictive Value were used as the prediction performance measures. For Naïve Bayes, the sensitivity of Class 1 in regular versus ‘Agree’ group increased from 0.687 to 0.936 and for Logistic Regression, it increased from 0.358 to 0.8306. This indicates the ‘Agree’ group predictions were more accurate and could be labeled as high-confidence ML predictions. The ‘Agree’ group consisted of 89% cases in the testing set, so the improved prediction performance was applicable for a large portion of the testing dataset.
更多查看译文
关键词
breast cancer prediction,breast cancer,bias,learning-based
AI 理解论文
溯源树
样例
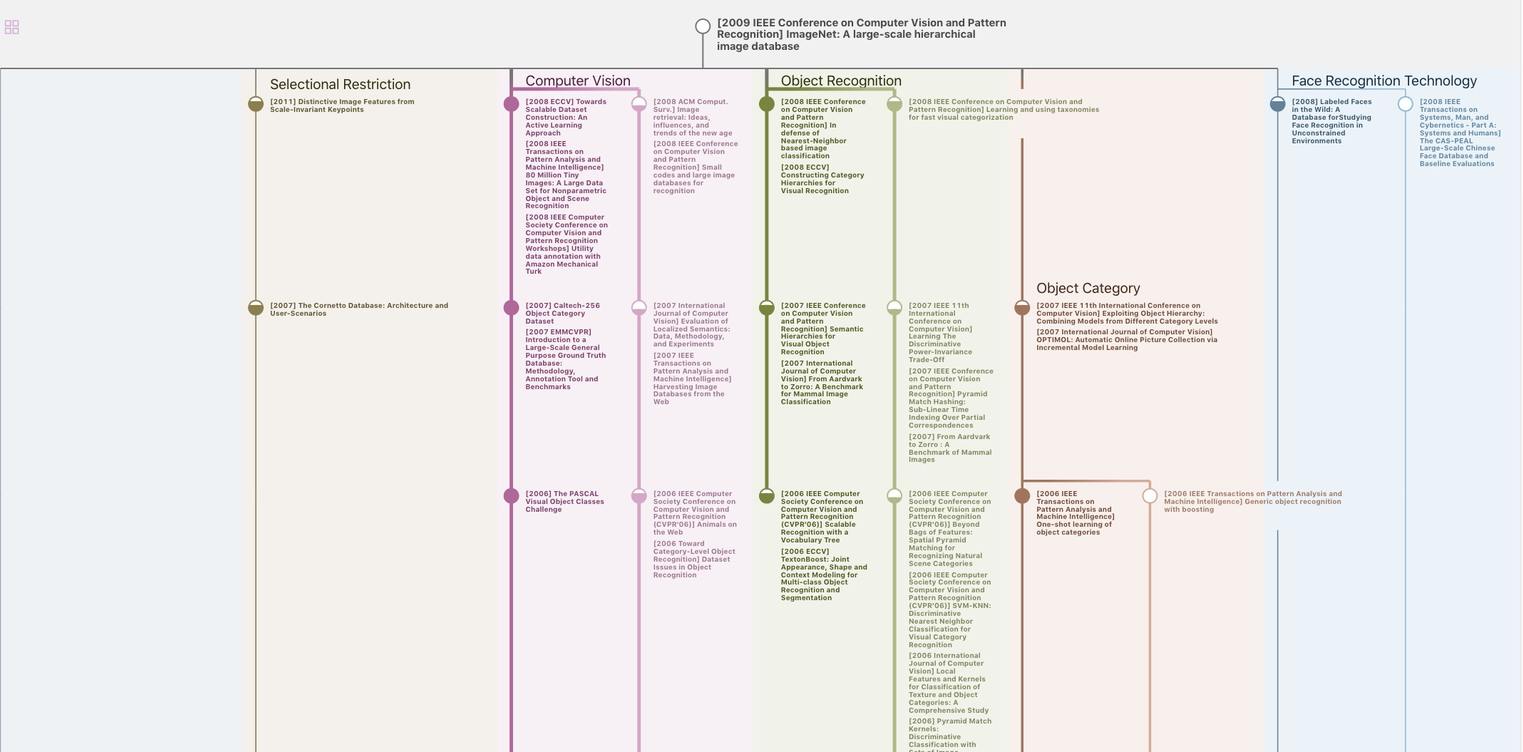
生成溯源树,研究论文发展脉络
Chat Paper
正在生成论文摘要