Measuring the Consistency and Diversity of 3D Face Generation
IEEE JOURNAL OF SELECTED TOPICS IN SIGNAL PROCESSING(2023)
摘要
3D-aware GANs have shown their impressive power on 3D controlling for synthesized portraits. While the plausible facial reality is achieved, the inherent 3D properties of the generated results have actually not been well analyzed. One of the reasons is that the wildly-used metrics, such as Inception Score (IS) or Frechet Inception Distance (FID), focus more on the perceptual features rather than explicit 3D clues. In this article, we propose two novel 3D metrics, which measure the face consistency and diversity on a 3D level, to compensate for IS or FID on GAN evaluation. With such metrics, the generated faces are systematically analyzed on a wide range of GANs, where we obtain reasonable but different conclusions from the wildly-used approaches. Inspired by the performance on the proposed metrics, we then discuss what is crucial for the GANs to get robust 3D properties, and what may bring uncertainty or inconsistency to the generating procedure. Finally, we propose two simple but efficient solutions to contribute to superior 3D synthesizing accuracy across different architectures, which further demonstrate the significance of the proposed metrics.
更多查看译文
关键词
Three-dimensional displays,Measurement,Solid modeling,Reliability,Image reconstruction,Face recognition,Generative adversarial networks,3D face,generative adversarial networks (GANs),GAN evaluation
AI 理解论文
溯源树
样例
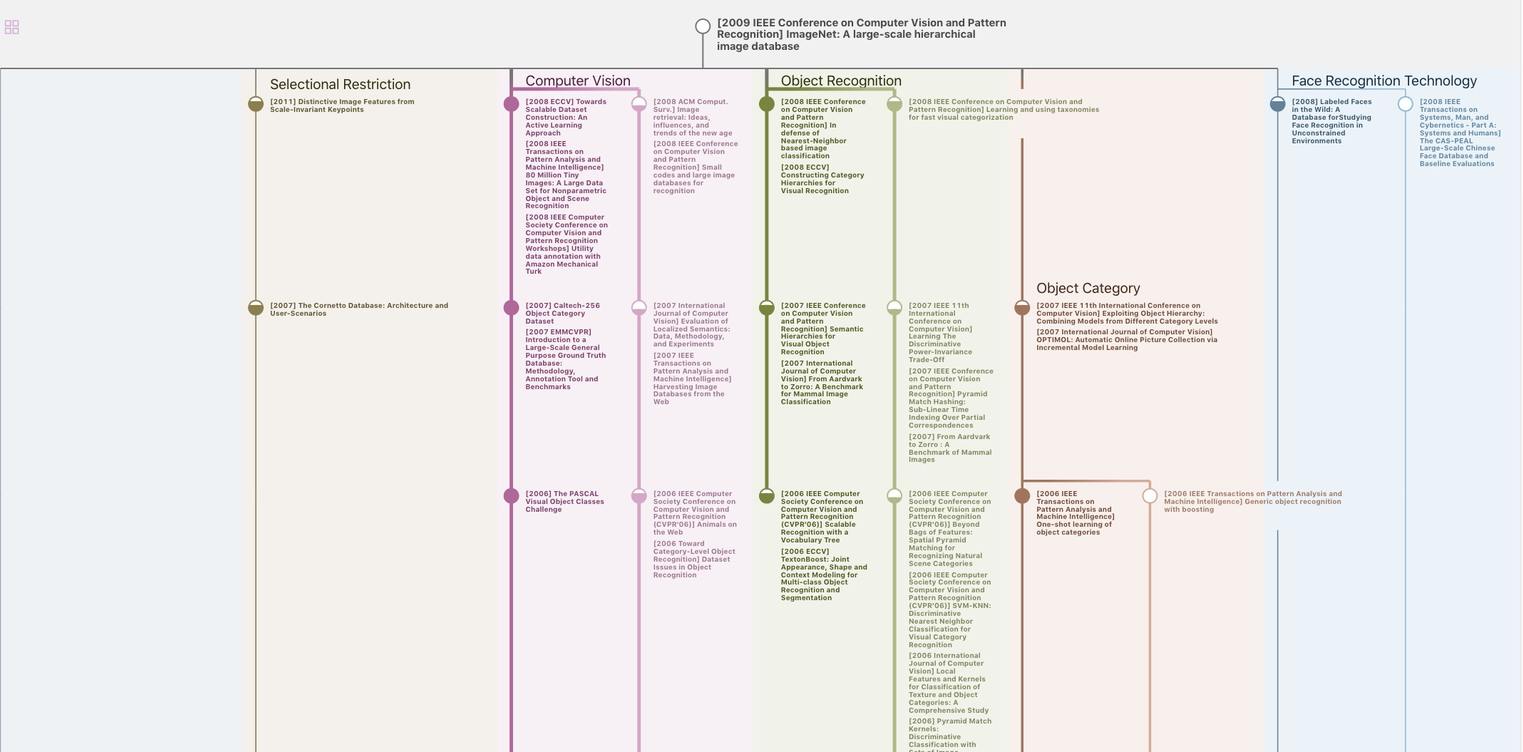
生成溯源树,研究论文发展脉络
Chat Paper
正在生成论文摘要