Importance Biased Traffic Scene Segmentation in Diverse Weather Conditions.
IEEE Trans. Intell. Veh.(2024)
摘要
Robust semantic segmentation under adverse weather conditions is an open challenge in autonomous driving applications. The main difficulty comes from the uncontrollability of the outdoor environment and the combined effects of various factors such as rain and fog. An importance biased network (IBN) model is proposed to improve the generalization of segmentation models for both hazardous and normal weather. It can adapt its prediction ability according to the severity of the weather, and gives high priority to the importance classes (such as road, person and car) when the conditions are too severe to maintain the accuracy of all classes. Importance biased module uses multi-branch decoder to divide the segmentation task into simplified sub-problems, and this divide-and-conquer strategy improves the robustness of the model especially under the severe hazardous conditions; class crosstalk module explicitly represents the sematic-level correlations between the classes, which enhances the information sharing among sub-problems. Comprehensive experiments on public datasets demonstrate the effectiveness of the method. Especially for severe weather conditions, 5∼6% accuracy improvements have been obtained for the important classes on both synthetic and real data.
更多查看译文
关键词
Sematic segmentation,Autonomous driving,Adverse weather,Importance biased network
AI 理解论文
溯源树
样例
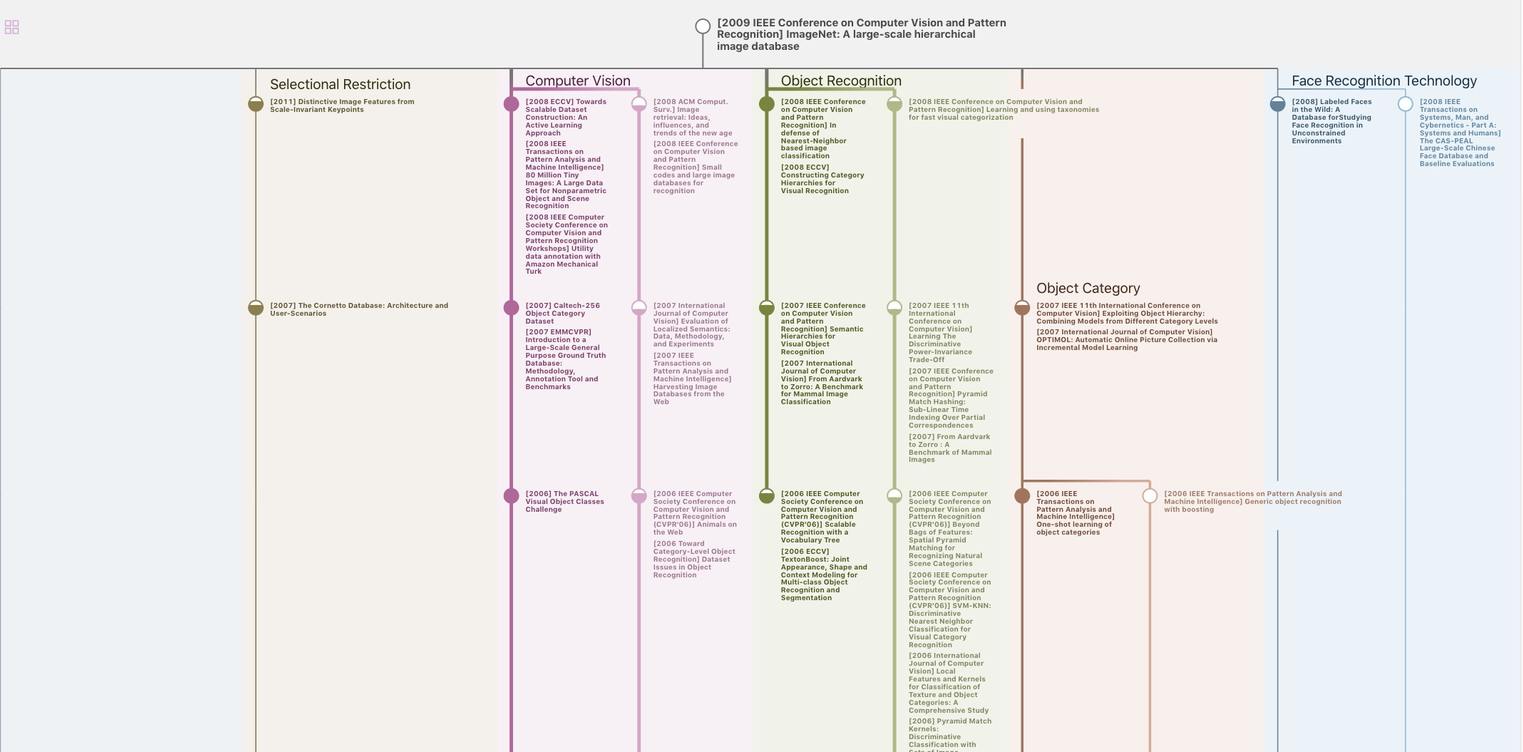
生成溯源树,研究论文发展脉络
Chat Paper
正在生成论文摘要