Efficient data-centric pest images identification method based on Mahalanobis entropy for intelligent agriculture
JOURNAL OF ELECTRONIC IMAGING(2023)
摘要
The frequent outbreak of crop pests is one of the main factors affecting crop yield. The accurate identification of pests is significant for effective pest control, which is the basis for the safe growth of crops and is also an important guarantee for high crop quality and crop yield. Computer vision based on supervised deep learning enables intelligent identification of pests, whose success is still inseparable from a large amount of labeled data, causing a large number of resource consumption due to data labeling. Therefore, an in-depth study on maximizing the value of data is essential when lacking labeled data. A new data evaluation method based on Mahalanobis distance and entropy is proposed to address the problem of lacking labeled data in intelligent pest identification. This method enables filtering high-value data, thus achieving effective pest identification performance with a small data volume. The experiment is conducted on a dataset we collected called PD-20, which shows the proposed method achieves baseline accuracy of 100% using only about 60% of the original data. Moreover, the proposed method can save at least 10% of the data volume compared with three comparison methods. To facilitate the deep integration of smart agriculture with artificial intelligence (AI), we designed an interactive framework of active learning for pest identification based on the proposed method, which lays the foundation for the application of AI in direction of agriculture.
更多查看译文
关键词
pest identification,supervised deep learning,active learning,smart agriculture,value of data
AI 理解论文
溯源树
样例
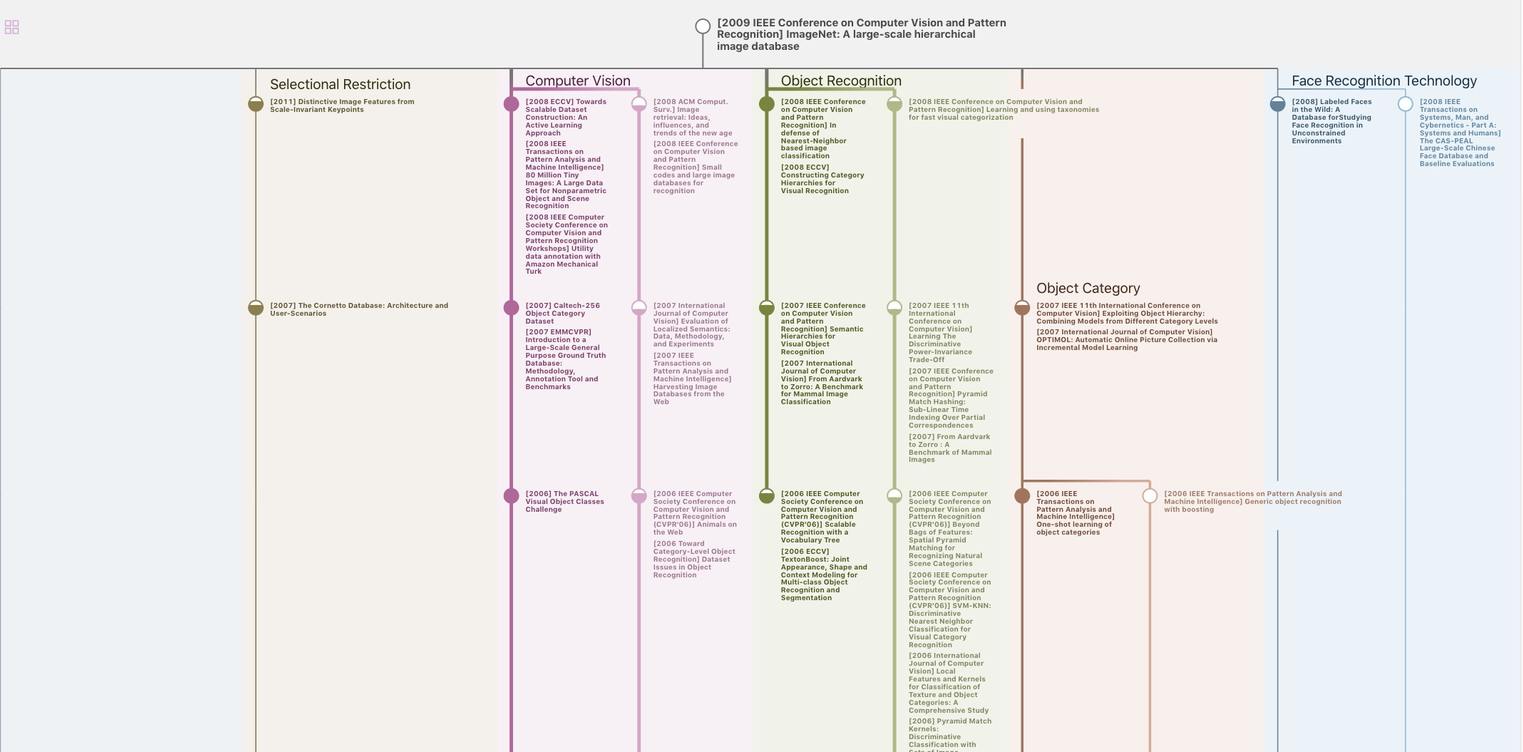
生成溯源树,研究论文发展脉络
Chat Paper
正在生成论文摘要