Decoding speech envelopes from electroencephalographic recordings: A comparison of regularized linear regression and long short-term memory deep neural network
Journal of the Acoustical Society of America(2023)
摘要
The speech envelope provides enough acoustic information to accurately recognize consonants and vowels (Shannon et al., 1995). The neural representation of speech envelopes is often assessed by reconstructing the envelopes from neural oscillations in the electroencephalogram (EEG) using linear decoders. One such approach is the multivariate temporal response function (mTRF), which achieves envelope reconstruction through regularized linear regression. Here, we compared the envelope reconstructions achieved by the mTRF and a non-linear alternative derived from a long-short term memory (LSTM) deep network. EEGs were collected from 15 native English speakers listening to an English audiobook (Reetzke et al., 2021). We trained a different decoder for each consonant and vowel in each listener. Reconstruction accuracy was measured as the Pearson coefficient (r) between observed and reconstructed envelopes. Preliminary results for the reconstruction of all vowels revealed that speech envelopes were moreaccurately reconstructed by the LSTM decoder (r: M = 0.247, SEM = 0.0024) than the mTRF (r: M = 0.074, SEM = 0.0025). Reconstruction accuracy was equally high and less variable across subjects for the LSTM approach. Additionally, high vowels showed lower decoding performance potentially due to their lower amplitude. These findings demonstrate the potential of non-linear approaches to investigating the neural representation of speech envelope cues.
更多查看译文
关键词
electroencephalographic recordings,speech envelopes,linear regression,neural network,short-term
AI 理解论文
溯源树
样例
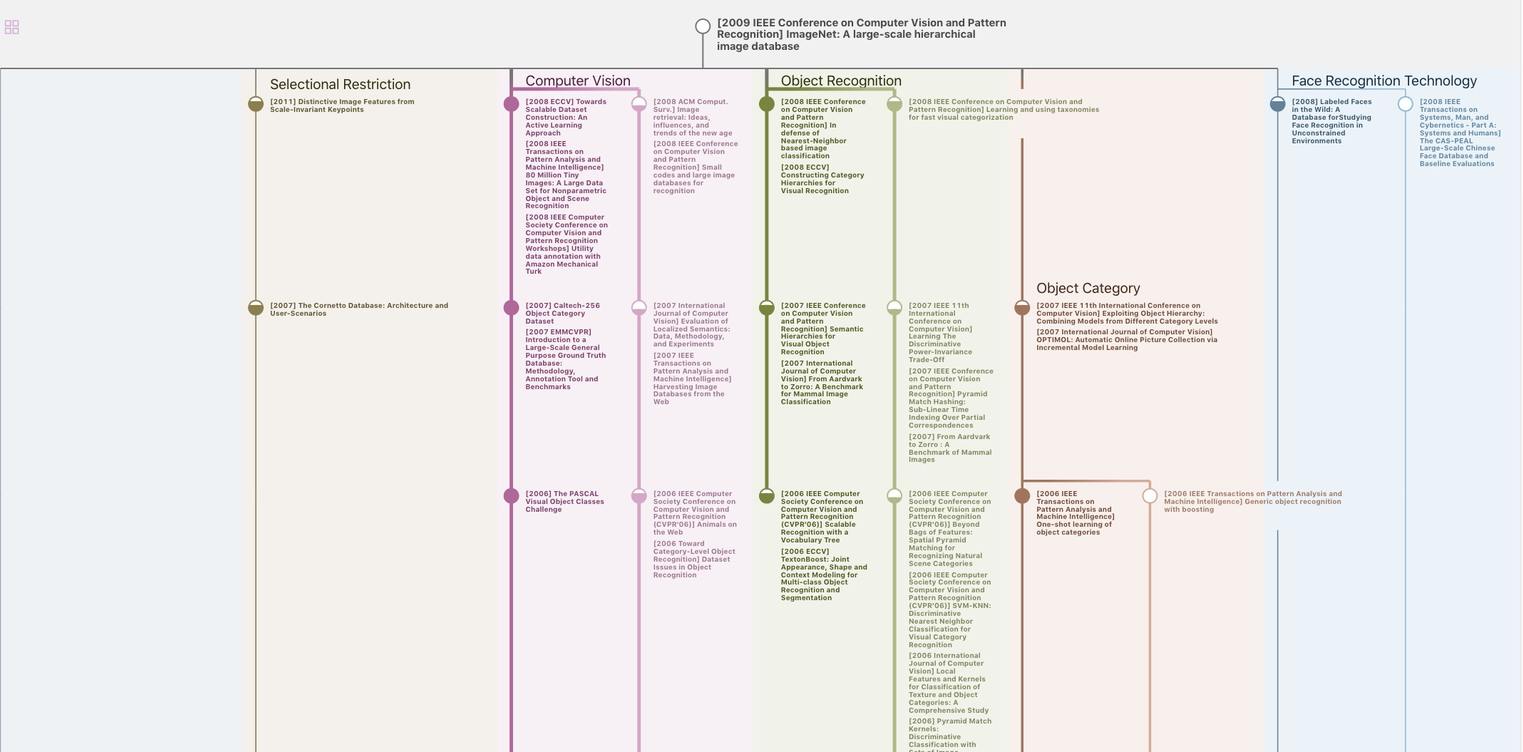
生成溯源树,研究论文发展脉络
Chat Paper
正在生成论文摘要