Aggregation of Disentanglement: Reconsidering Domain Variations in Domain Generalization
arXiv (Cornell University)(2023)
摘要
Domain Generalization (DG) is a fundamental challenge for machine learning models, which aims to improve model generalization on various domains. Previous methods focus on generating domain invariant features from various source domains. However, we argue that the domain variantions also contain useful information, ie, classification-aware information, for downstream tasks, which has been largely ignored. Different from learning domain invariant features from source domains, we decouple the input images into Domain Expert Features and noise. The proposed domain expert features lie in a learned latent space where the images in each domain can be classified independently, enabling the implicit use of classification-aware domain variations. Based on the analysis, we proposed a novel paradigm called Domain Disentanglement Network (DDN) to disentangle the domain expert features from the source domain images and aggregate the source domain expert features for representing the target test domain. We also propound a new contrastive learning method to guide the domain expert features to form a more balanced and separable feature space. Experiments on the widely-used benchmarks of PACS, VLCS, OfficeHome, DomainNet, and TerraIncognita demonstrate the competitive performance of our method compared to the recently proposed alternatives.
更多查看译文
关键词
domain variations,disentanglement,aggregation
AI 理解论文
溯源树
样例
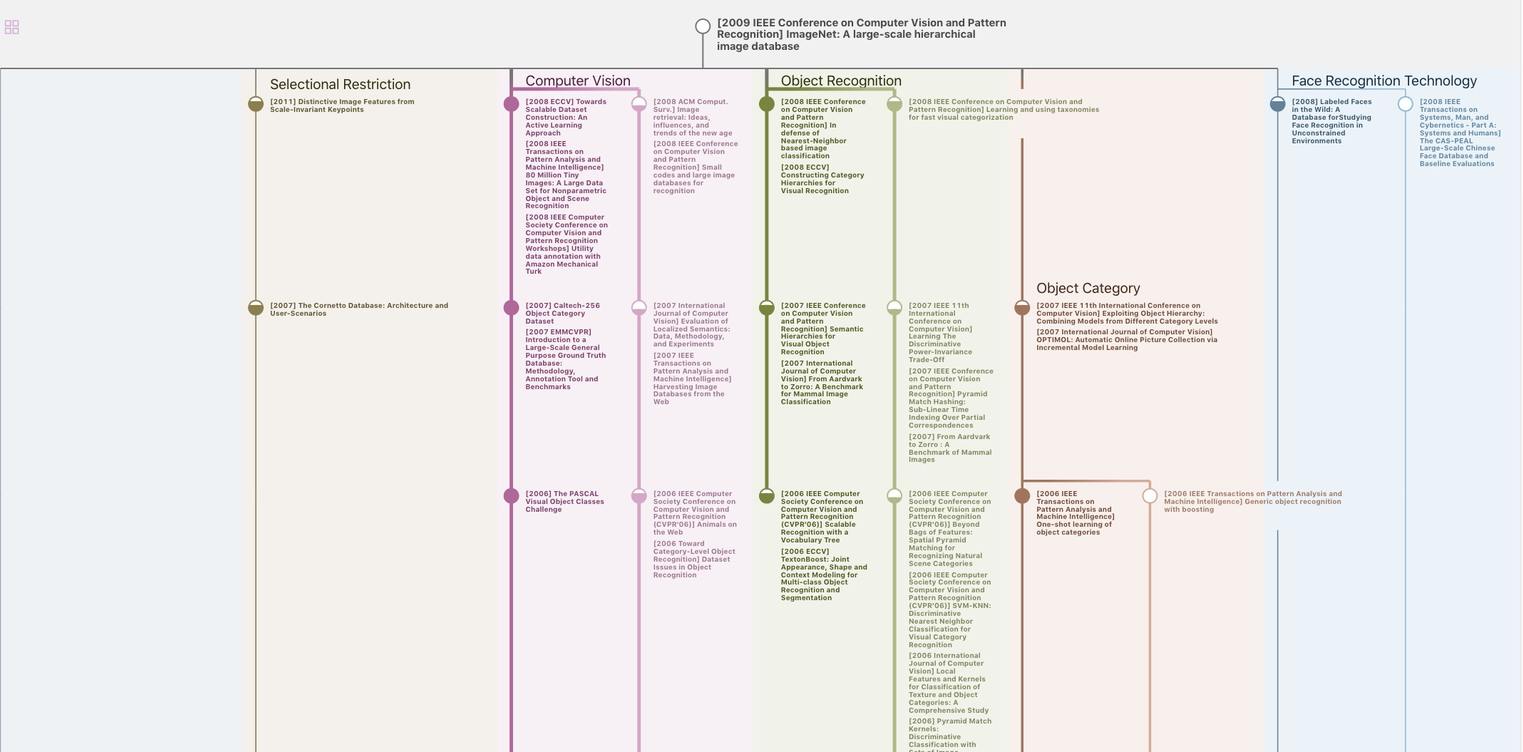
生成溯源树,研究论文发展脉络
Chat Paper
正在生成论文摘要