FedLEO: An Offloading-Assisted Decentralized Federated Learning Framework for Low Earth Orbit Satellite Networks
IEEE Transactions on Mobile Computing(2023)
摘要
Low Earth orbit (LEO) satellites enable complex Earth observation tasks (
e.g.,
remote sensing and cooperative monitoring) by leveraging large-scale satellite-generated Earth imageries and state-of-the-art machine learning (ML) techniques. However, due to restricted downlink bandwidth and spotty connectivity, it is infeasible for the satellites to transmit all the imageries to ground stations for ML model training. To address this issue, we use federated learning (FL) to mitigate the significant overhead of raw data transmission only by enabling model parameter exchange. Traditional FL requires a central server for model parameter aggregation, which is impractical for distributed LEO satellite constellation due to the difficulty of identifying a suitable central satellite. To tackle such challenge, we take the unique topological characteristics of the LEO satellite constellation to design a decentralized FL framework that enables efficient model aggregation in LEO satellite networks without a central server. The framework can avoid the reliability and communication bandwidth problems of the central server in centralized FL. To mitigate the straggler effect and address the statistical heterogeneity, we then propose a novel offloading framework for decentralized FL in LEO satellite networks to aid the collaboration among multiple satellites for resource sharing. Based on it, we derive a satellite-centric threshold-based offloading strategy and a system-wide greedy-based iterative offloading decision making algorithm, in order to achieve delay and accuracy optimization under the computation and communication power constraints. Theoretical analysis demonstrates that the proposed framework contributes to the high training performance of the global model. Extensive experiments based on realistic datasets show that the proposed framework can reduce the system delay by up to 41% on average and improve the global model accuracy by up to 9.39% compared with benchmark policies.
更多查看译文
关键词
Federated learning,low earth orbit satellite networks,statistical heterogeneity,straggler effect
AI 理解论文
溯源树
样例
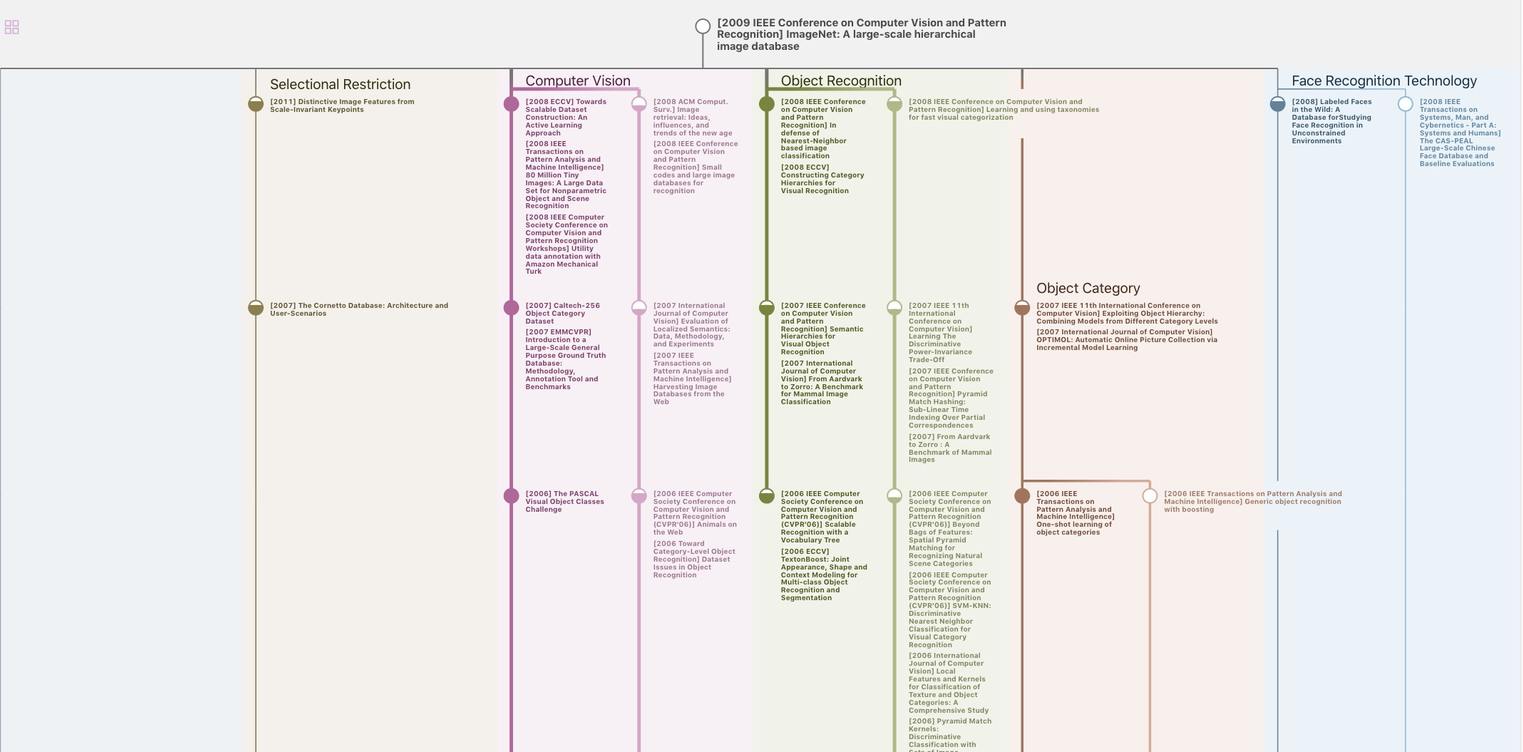
生成溯源树,研究论文发展脉络
Chat Paper
正在生成论文摘要