Online Preventive Control for Transmission Overload Relief Using Safe Reinforcement Learning With Enhanced Spatial-Temporal Awareness
IEEE TRANSACTIONS ON POWER SYSTEMS(2024)
摘要
The risk of transmission overload (TO) in power grids is increasing with the large-scale integration of intermittent renewable energy sources. An effective online preventive control schemes proves to be vital in safeguarding the security of power systems. In this paper, we formulate the online preventive control problem for TO alleviation as a constrained Markov decision process (CMDP), targeted to reduce the load rate of overloaded lines by implementing generation re-dispatch, transmission and busbar switching actions. The CMDP is solved with a state-of-the-art safe deep reinforcement learning method, based on the computationally efficient interior-point policy optimization (IPO), which facilitates desirable learning behavior towards constraint satisfaction and policy improvement simultaneously. The performance of IPO method is further improved with an enhanced perception of spatial-temporal correlations in power gird nodal and edge features, combining the strength of edge conditioned convolutional network and long short-term memory network, fostering more effective and robust preventive control policies to be devised. Case studies on a real-world system and a large-scale system validate the superior performance of the proposed method in TO alleviation, constraint handling, uncertainty adaptability and stability preservation, as well as its favorable computational performance, through benchmarking against both model-based and reinforcement learning-based baseline methods.
更多查看译文
关键词
Optimization,Substations,Generators,Switches,Uncertainty,Indexes,Voltage control,Constrained Markov decision process,online preventive control,power system spatial-temporal perception,safe deep reinforcement learning,transmission overload
AI 理解论文
溯源树
样例
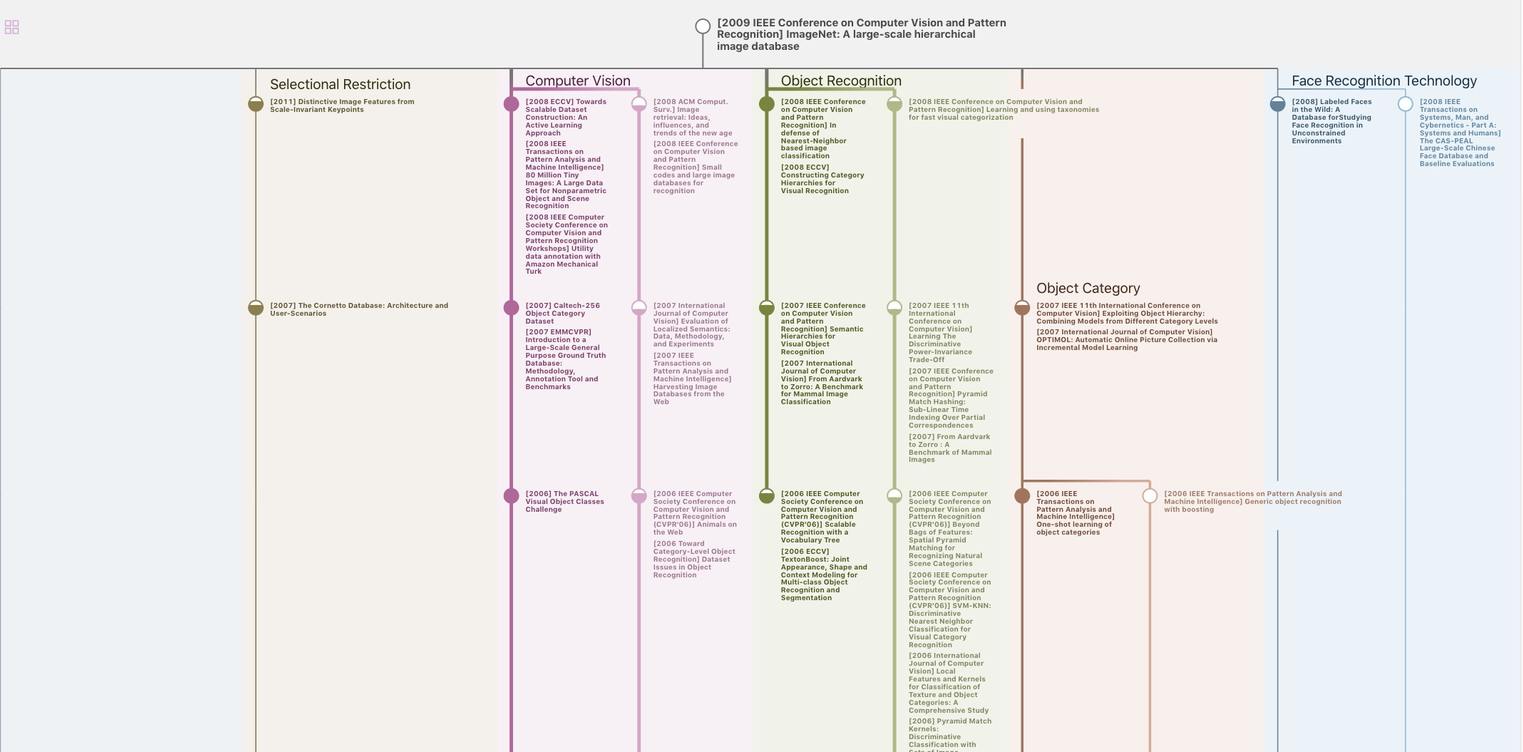
生成溯源树,研究论文发展脉络
Chat Paper
正在生成论文摘要