GIFAIR-FL: A Framework for Group and Individual Fairness in Federated Learning
INFORMS journal on data science(2023)
摘要
In this paper we propose \texttt{GIFAIR-FL}: a framework that imposes \textbf{G}roup and \textbf{I}ndividual \textbf{FAIR}ness to \textbf{F}ederated \textbf{L}earning settings. By adding a regularization term, our algorithm penalizes the spread in the loss of client groups to drive the optimizer to fair solutions. Our framework \texttt{GIFAIR-FL} can accommodate both global and personalized settings. Theoretically, we show convergence in non-convex and strongly convex settings. Our convergence guarantees hold for both $i.i.d.$ and non-$i.i.d.$ data. To demonstrate the empirical performance of our algorithm, we apply our method to image classification and text prediction tasks. Compared to existing algorithms, our method shows improved fairness results while retaining superior or similar prediction accuracy.
更多查看译文
关键词
individual fairness,group,learning
AI 理解论文
溯源树
样例
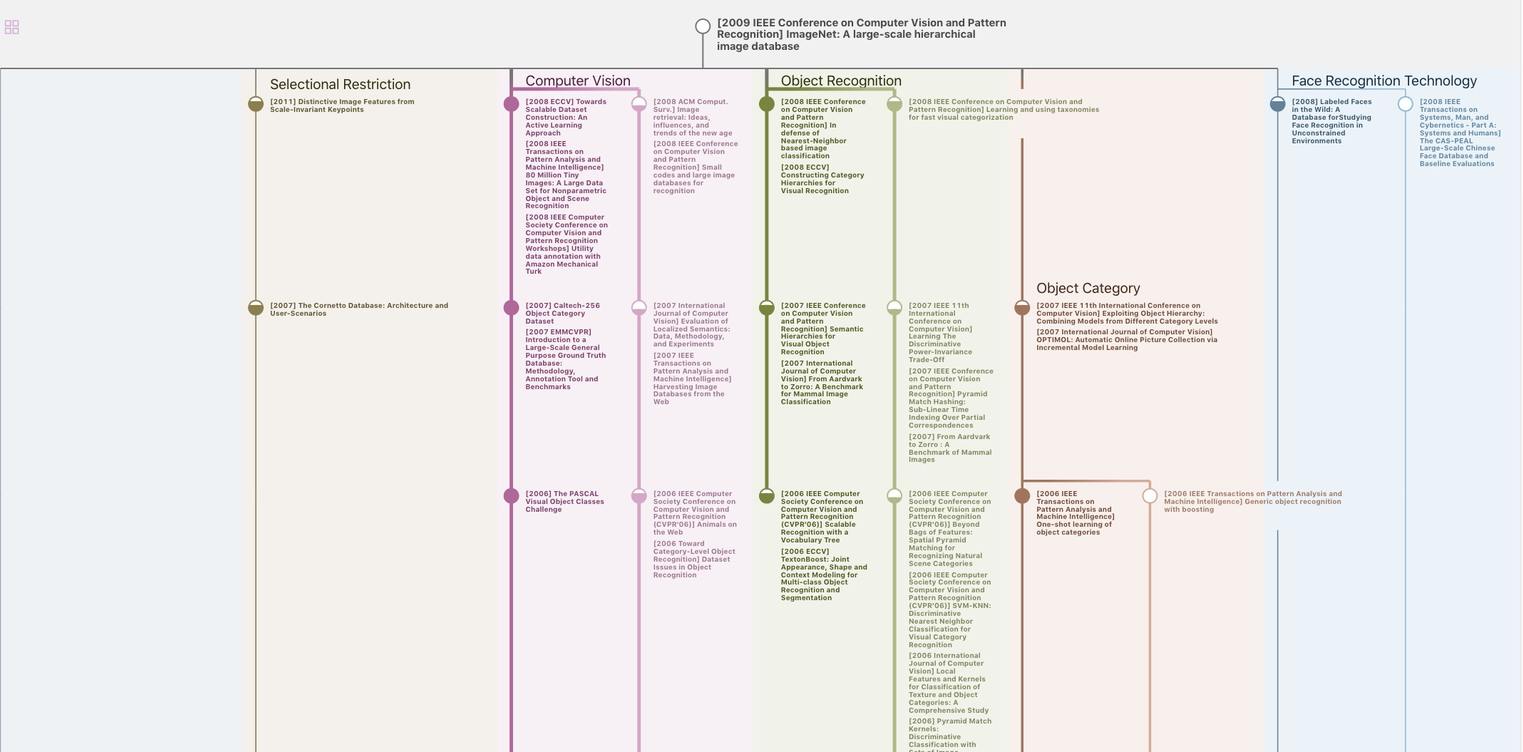
生成溯源树,研究论文发展脉络
Chat Paper
正在生成论文摘要