Secretary and online matching problems with machine learned advice
Discrete Optimization(2023)
摘要
The classic analysis of online algorithms, due to its worst-case nature, can be quite pessimistic when the input instance at hand is far from worst-case. In contrast, machine learning approaches shine in exploiting patterns in past inputs in order to predict the future. However, such predictions, although usually accurate, can be arbitrarily poor. Inspired by a recent line of work, we augment three well-known online settings with machine learned predictions about the future, and develop algorithms that take these predictions into account. In particular, we study the following online selection problems: (i) the classic secretary problem, (ii) online bipartite matching and (iii) the graphic matroid secretary problem. Our algorithms still come with a worst-case performance guarantee in the case that predictions are subpar while obtaining an improved competitive ratio (over the best-known classic online algorithm for each problem) when the predictions are sufficiently accurate. For each algorithm, we establish a trade-off between the competitive ratios obtained in the two respective cases.
更多查看译文
关键词
online matching problems,secretary
AI 理解论文
溯源树
样例
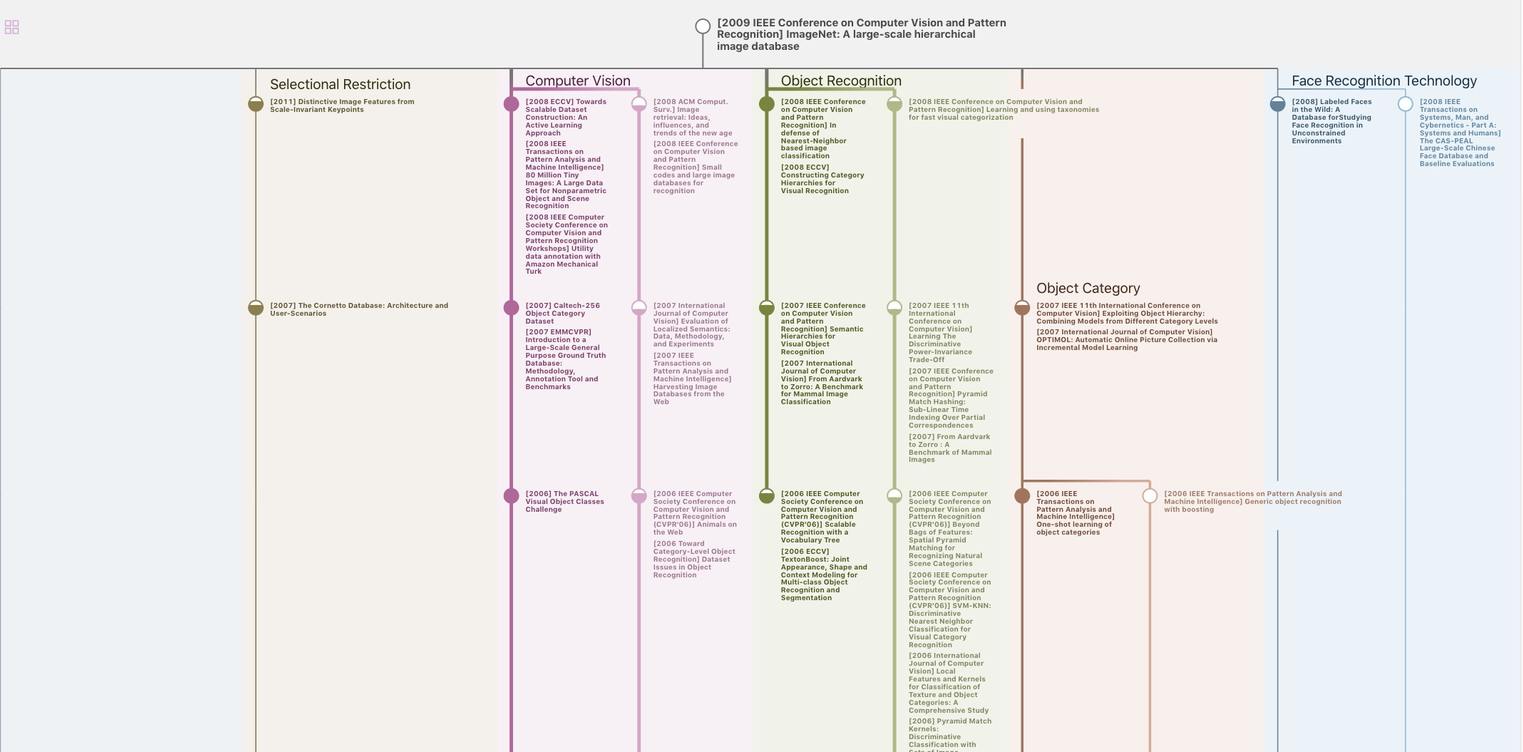
生成溯源树,研究论文发展脉络
Chat Paper
正在生成论文摘要