Application of a Hybrid Machine Learning Model for the Detection and Diagnosis of Multiple Dependent Faults in an Air Handling Unit
Environmental science and engineering(2023)
摘要
The goal of this paper is the detection and diagnosis of dependent multiple faults (MDFDD) in an air handling unit (AHU) of an institutional building. Three machine learning models are developed using experimental data, the artificial neural network, decision tree, and random forest. The models predict the air temperature that should be measured during normal operation conditions in AHU. Artificial faults are generated in this paper. If the residual of measurement and predicted value by ML models exceeds the threshold, a fault symptom is detected. The threshold is calculated by using the total sensor uncertainty and average root-mean-squared-error of the prediction using ML models over the training data set. With the aggregation of these two parameters the threshold is defined for the target sensor. Once the fault symptoms are detected, some rule-based models are used for the diagnosis. The results revealed good performance of the proposed model on the MDFDD.
更多查看译文
关键词
hybrid machine learning model,multiple dependent faults,machine learning model,machine learning,detection
AI 理解论文
溯源树
样例
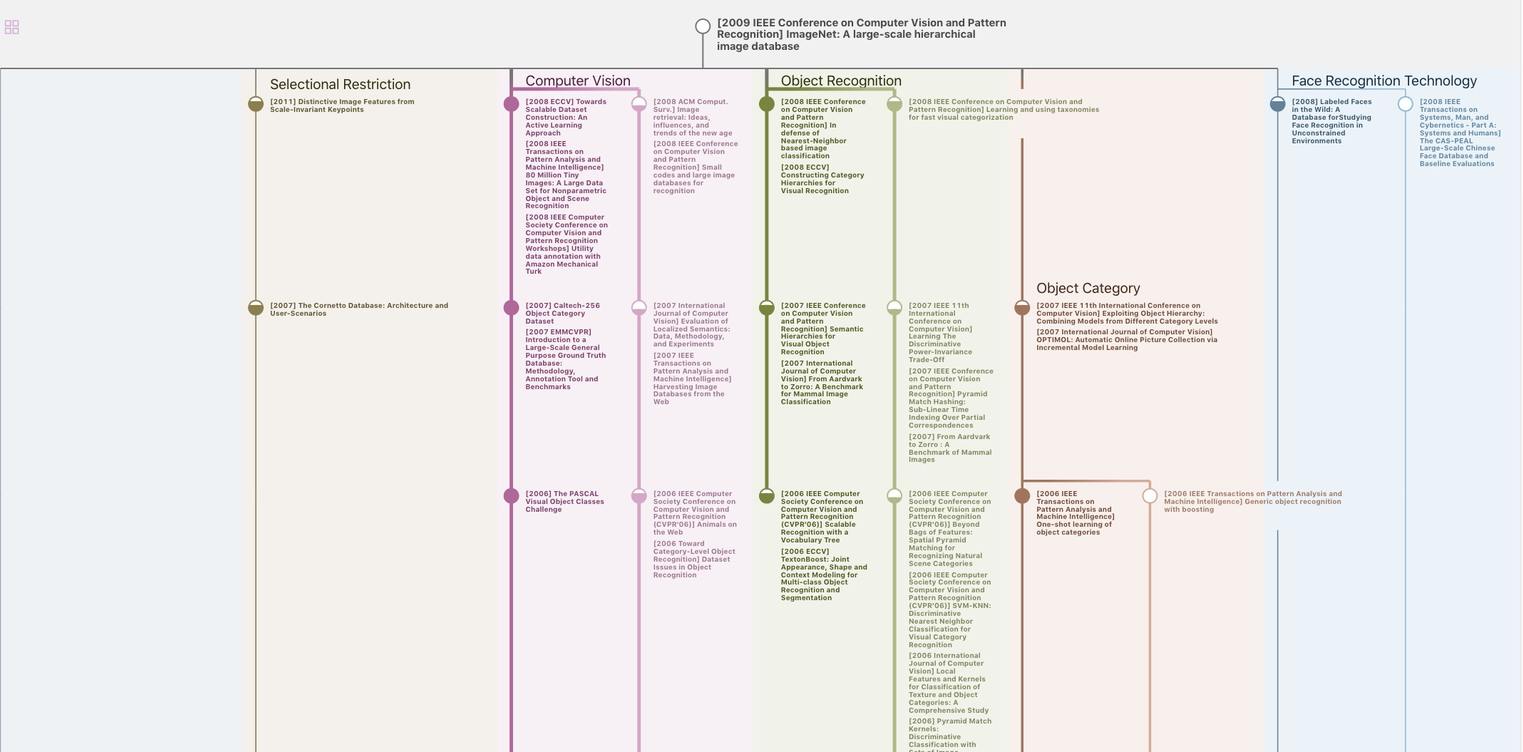
生成溯源树,研究论文发展脉络
Chat Paper
正在生成论文摘要