AL4SLEO: An Active Learning Solution for the Semantic Labelling of Earth Observation Satellite Images—Part 2
Springer tracts in nature-inspired computing(2023)
摘要
In the previous chapter (Part 1), a cascaded Active Learning method was proposed as an efficient solution to three problem cases being typical for Earth observation images: (1) Multi-label semantics, (2) Multi-sensor semantic labelling, and (3) Multi-temporal semantic labelling. In this Part 2, the method is demonstrated using the data acquired by different space-borne sensors such as: TerraSAR-X, Sentinel-1, WorldView-2, and Sentinel-2. The accuracy of $$90\%$$ obtained for the proposed cascaded Active Learning method was compared with traditional or deep learning methods (e.g., k-NN, Latent Dirichlet Allocation (LDA), Convolutional Neural Network (CNN)), and the results demonstrate that the proposed method is at least equal to or better than the methods we used for comparison. This active learning method can be used successfully for benchmark generation of large Earth observation (EO) data sets.
更多查看译文
关键词
earth observation satellite images—part,active learning solution,semantic labelling,active learning
AI 理解论文
溯源树
样例
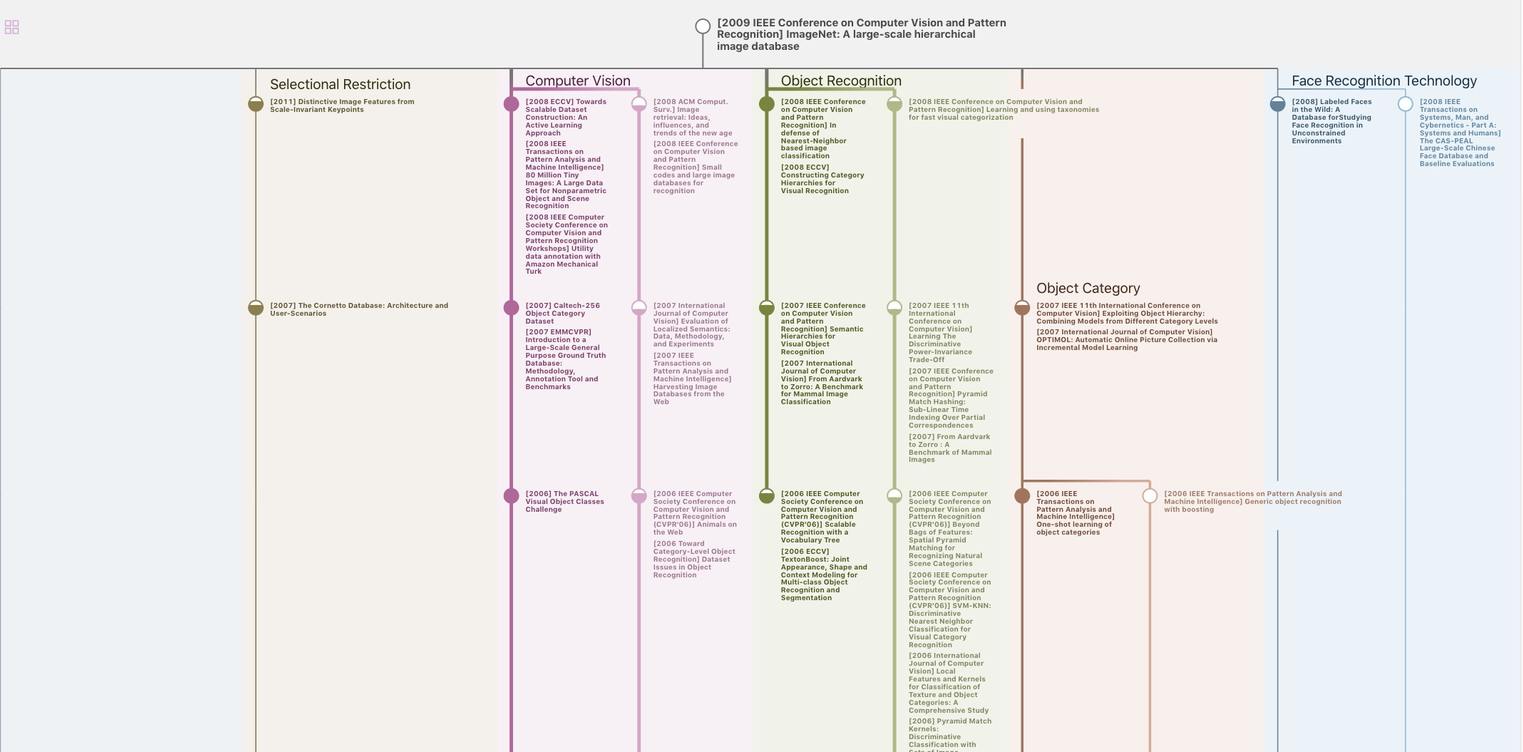
生成溯源树,研究论文发展脉络
Chat Paper
正在生成论文摘要