Intrusion Detection with Black Hole Feature Selection
Smart innovation, systems and technologies(2023)
摘要
The present work describes the application of two different Black Hole algorithms for feature selection in intrusion detection systems. The first algorithm employs a binary algorithm in which the population of stars is denoted by a feature vector of size equivalent to original attribute size in the dataset. In this algorithm, the ones denote attributes selected and zeros denote attributes not selected. Each star is represented by the subsets which are evaluated by the random forest classifier and the best star is designated as the Black Hole. The stars iteratively move towards the Black Hole, and the best subset of features are obtained after convergence. The second algorithm is called the Real Coded Black Hole algorithm wherein the elements of the feature vector contain values ranging from zeros to one. Here, a feature in a given star that possesses a value greater than or equal to 0.5 (threshold) is selected in the feature subset which represents that star. The feature subsets associated with each star are then evaluated using a random forest classifier, while the rest of the algorithm follows the same steps as the Binary Black Hole algorithm. These two Black Hole algorithms are evaluated using three different datasets which include NSL-KDD, CIC-IDS2017 and the Aegean Wi-Fi intrusion dataset. The simulations in the proposed algorithms are compared with existing feature selection algorithms, and it is observed that proposed algorithms provide significantly better results.
更多查看译文
关键词
black hole feature selection,feature selection,black hole,detection
AI 理解论文
溯源树
样例
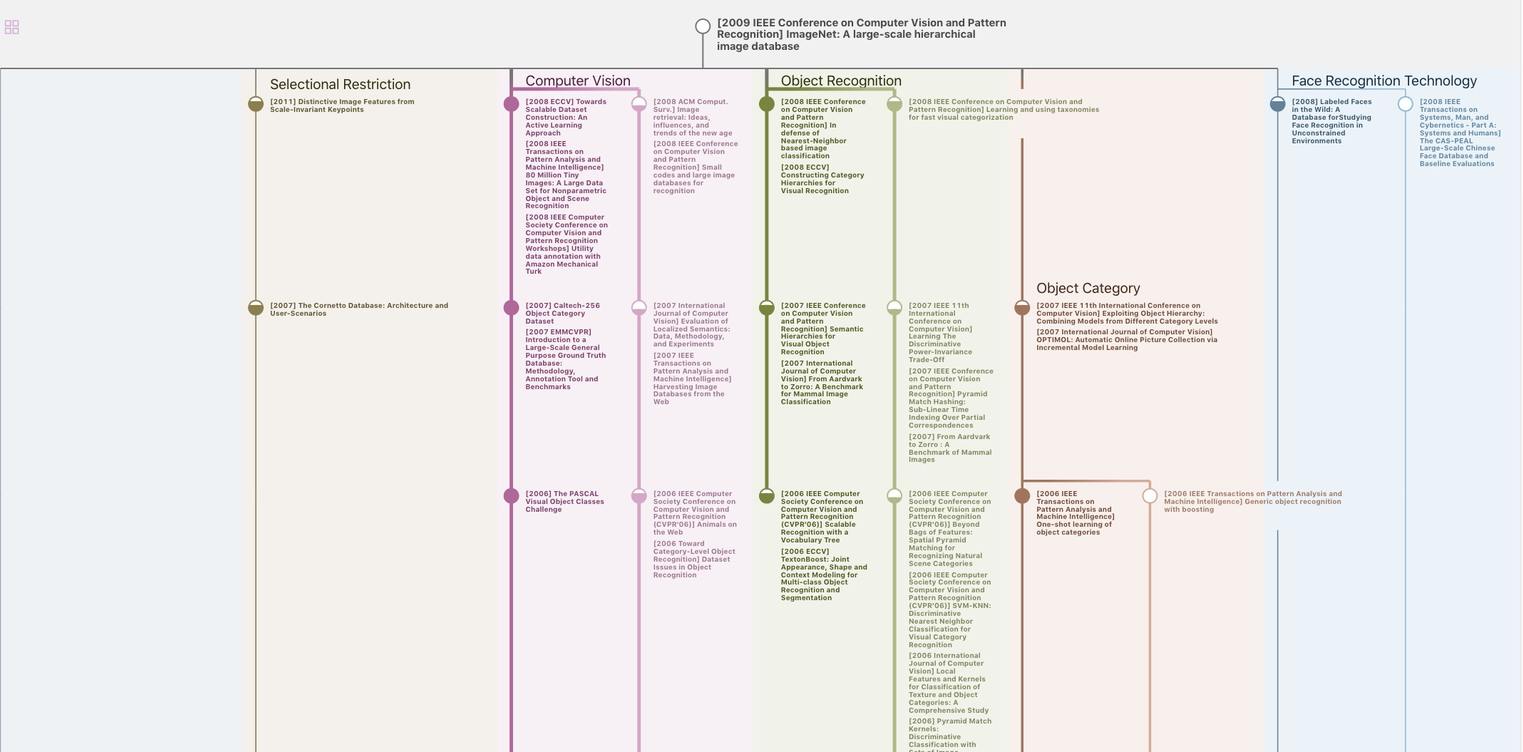
生成溯源树,研究论文发展脉络
Chat Paper
正在生成论文摘要