Tipping the Balance: Imbalanced Classes in Deep-Learning Side-Channel Analysis
IEEE DESIGN & TEST(2024)
摘要
Machine learning, and more recently, deep learning, have become a standard option for profiling side-channel analysis (SCA) to evaluate the worst-case security. Machine learning-based SCA has advantages over previous approaches like the template attack [1], especially in practical settings where the number of training traces is limited. The advantages of deep learning-based approaches are even more pronounced as such techniques can break protected implementations without feature selection and by using relatively small models (neural networks), [2]. However, the use of popular device leakage models brings in the issue of imbalanced datasets. For instance, Hamming weight or distance model follows a binomial distribution resulting in significantly more training samples in central classes. Further, evaluating the performance of machine learning-based SCA with standard machine learning metrics like accuracy can be misleading. Unfortunately, this problem is not trivial to circumvent by “just” using the SCA metrics as the training process with them is difficult.
更多查看译文
关键词
Training,Measurement,Machine learning algorithms,Prediction algorithms,Deep learning,Support vector machines,Ciphers
AI 理解论文
溯源树
样例
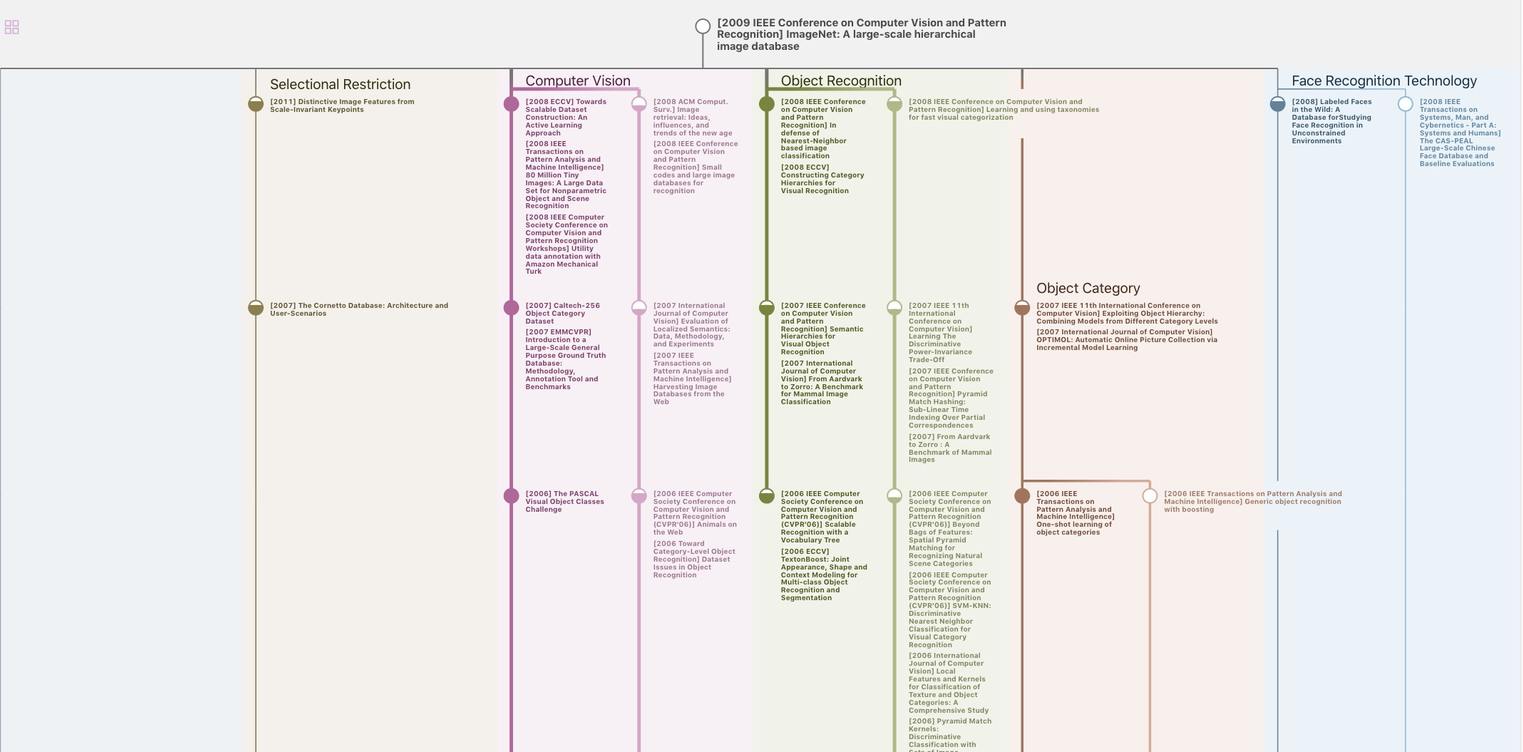
生成溯源树,研究论文发展脉络
Chat Paper
正在生成论文摘要