Infrared-Visible Synthetic Data from Game Engine for Image Fusion Improvement
IEEE transactions on games(2023)
摘要
Limited by the shooting scenes and angles of fixed cameras, the existing datasets generally lack many detailed pedestrian models in diverse scenarios. Existing deep learning-based image fusion methods, for this reason, bring about overfitting or insufficient information of fusion results in varying degrees. To address this challenge, A new infrared-visible pedestrian synthetic dataset (GIVF) with a synthetic data tagger (GSDT) is constructed and an improved end-to-end image fusion network (FSGAN) is proposed to validate infrared and visible fusion. In the model, the method uses an auxiliary network to extract features that complement the cascade network of the main path, effectively improving the ability to extract pedestrian texture details. Experimental results show that FSGAN can be well applied to GIVF. By conducting extensive comparative experiments with eight state-of-the-art image fusion methods. FSGAN shows better performance than those comparison methods, especially in the two evaluation indexes visual information fidelity (VIF) and structural similarity measurement (SSIM). Besides, by comparing the quantitative analysis results of various methods, and evaluating the fusion results of real images in complex environments on other three datasets, we conclude that FSGAN can be better applied to GIVF datasets than other popular methods, and has outstanding performance in generalization.
更多查看译文
关键词
Image Fusion,Generative Adversarial Network,Synthetic DataSet,Infrared and Visible Image,Feature Supplement
AI 理解论文
溯源树
样例
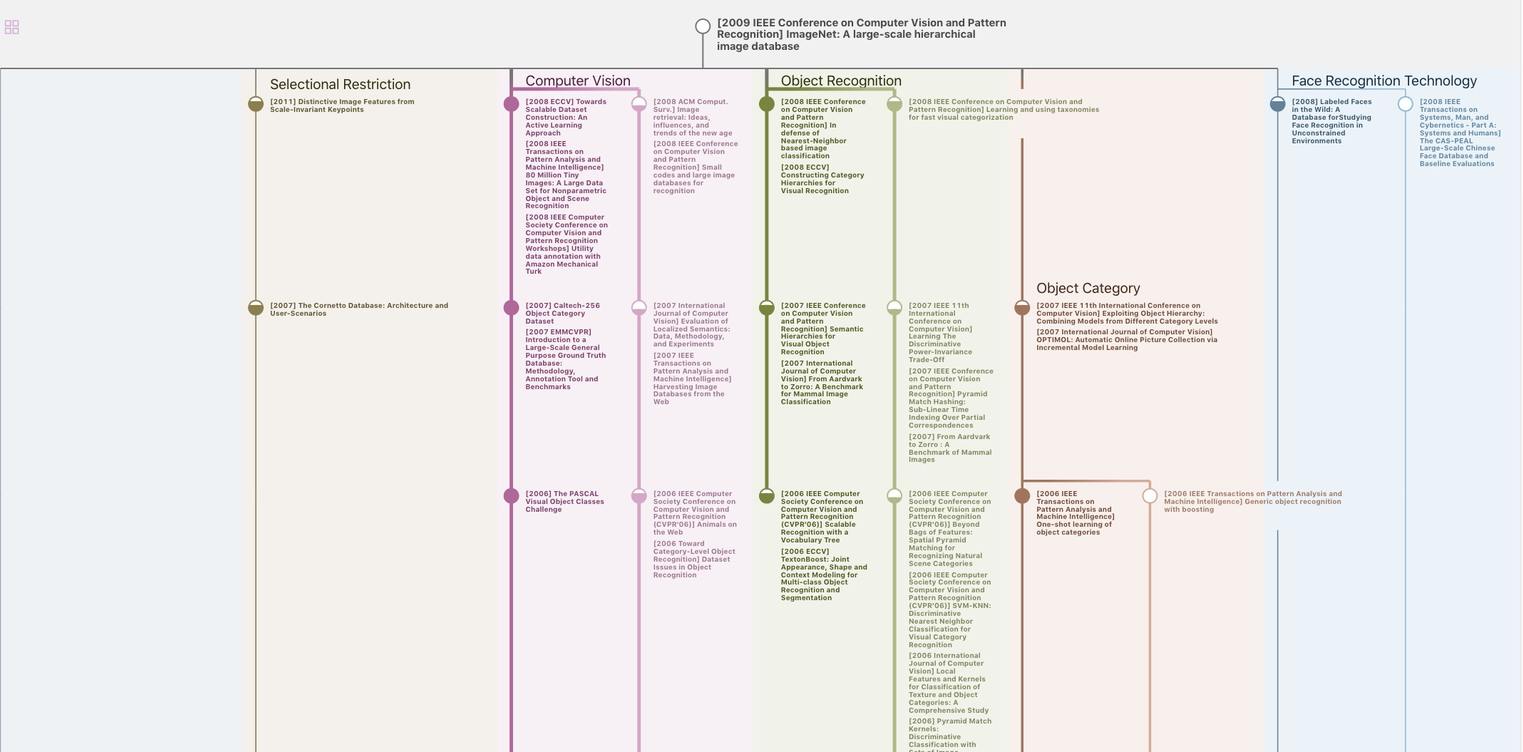
生成溯源树,研究论文发展脉络
Chat Paper
正在生成论文摘要