Contextual Conservative Q-Learning for Offline Reinforcement Learning
arXiv (Cornell University)(2023)
摘要
Offline reinforcement learning learns an effective policy on offline datasets without online interaction, and it attracts persistent research attention due to its potential of practical application. However, extrapolation error generated by distribution shift will still lead to the overestimation for those actions that transit to out-of-distribution(OOD) states, which degrades the reliability and robustness of the offline policy. In this paper, we propose Contextual Conservative Q-Learning(C-CQL) to learn a robustly reliable policy through the contextual information captured via an inverse dynamics model. With the supervision of the inverse dynamics model, it tends to learn a policy that generates stable transition at perturbed states, for the fact that pertuebed states are a common kind of OOD states. In this manner, we enable the learnt policy more likely to generate transition that destines to the empirical next state distributions of the offline dataset, i.e., robustly reliable transition. Besides, we theoretically reveal that C-CQL is the generalization of the Conservative Q-Learning(CQL) and aggressive State Deviation Correction(SDC). Finally, experimental results demonstrate the proposed C-CQL achieves the state-of-the-art performance in most environments of offline Mujoco suite and a noisy Mujoco setting.
更多查看译文
关键词
reinforcement q-learning
AI 理解论文
溯源树
样例
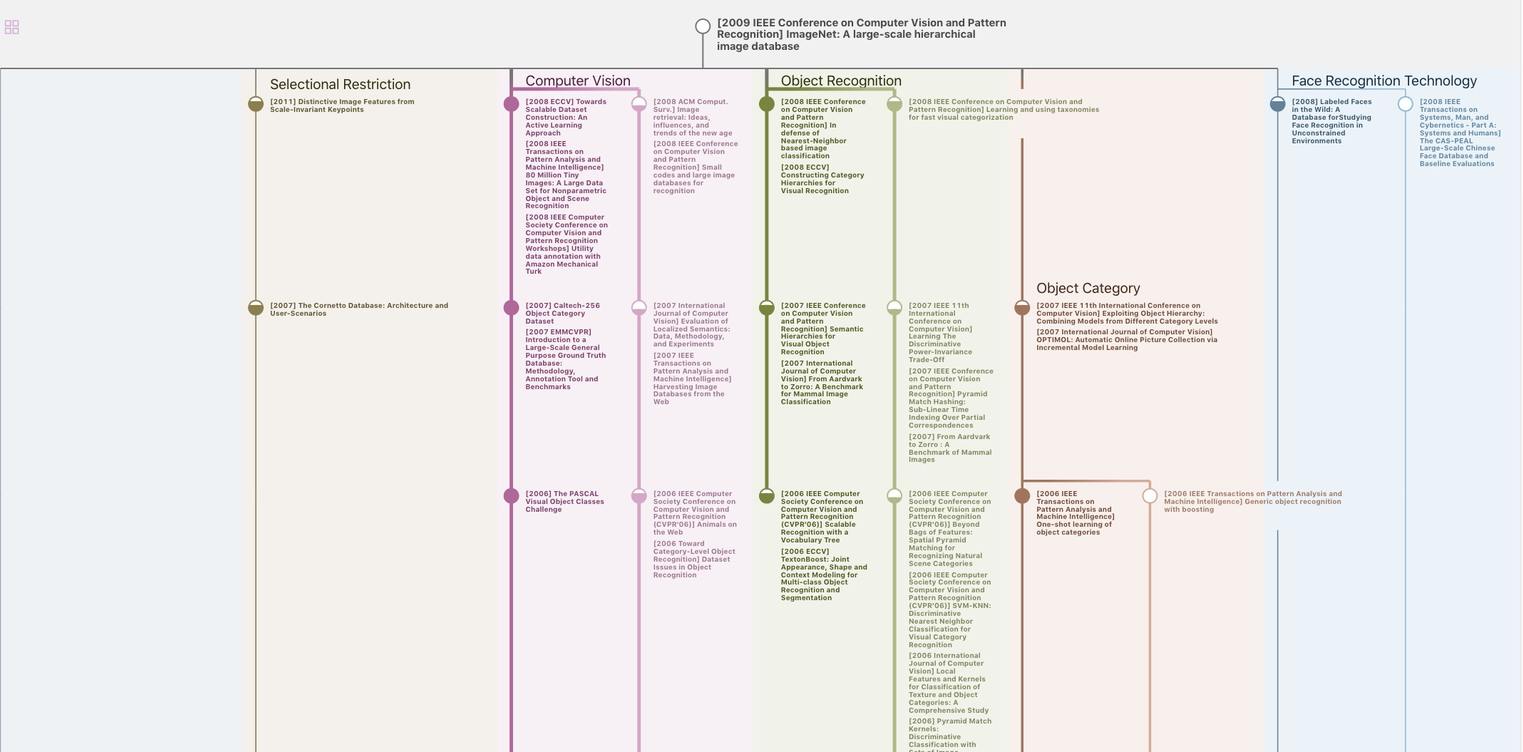
生成溯源树,研究论文发展脉络
Chat Paper
正在生成论文摘要