Lightweight Imitation Learning for Real-Time Cooperative Service Migration
IEEE TRANSACTIONS ON MOBILE COMPUTING(2024)
摘要
Due to the revolution of communication technology, the rapidly increasing number of mobile devices in edge networks generates various real-time service requests, requiring a considerable volume of heterogeneous resources all the time. However, edge devices with limited resources cannot afford substantial learning cost, while migrating services requires heterogeneous resources, especially for dynamic networks. To address these issues, we first establish a cooperative service migration framework and formulate a bi-objective optimization problem to optimize service performance and cost. By analyzing the optimal migration ratio of service cooperative migration, we propose an offline expert policy based on global states to provide optimal expert demonstrations. To realize real-time service migration based on observable states, we design a lightweight online agent policy to imitate expert demonstrations and leverage meta update to accelerate the model transfer. Experimental results show that our algorithm is exceptional in training cost and accuracy, and has significant superiors in multiple metrics such as the service latency and payment under different workloads, compared to other representative algorithms.
更多查看译文
关键词
Real-time systems,Task analysis,Games,Costs,Mobile computing,Training,Robots,Dynamic wireless network,imitation learning,resource cooperation,service migration
AI 理解论文
溯源树
样例
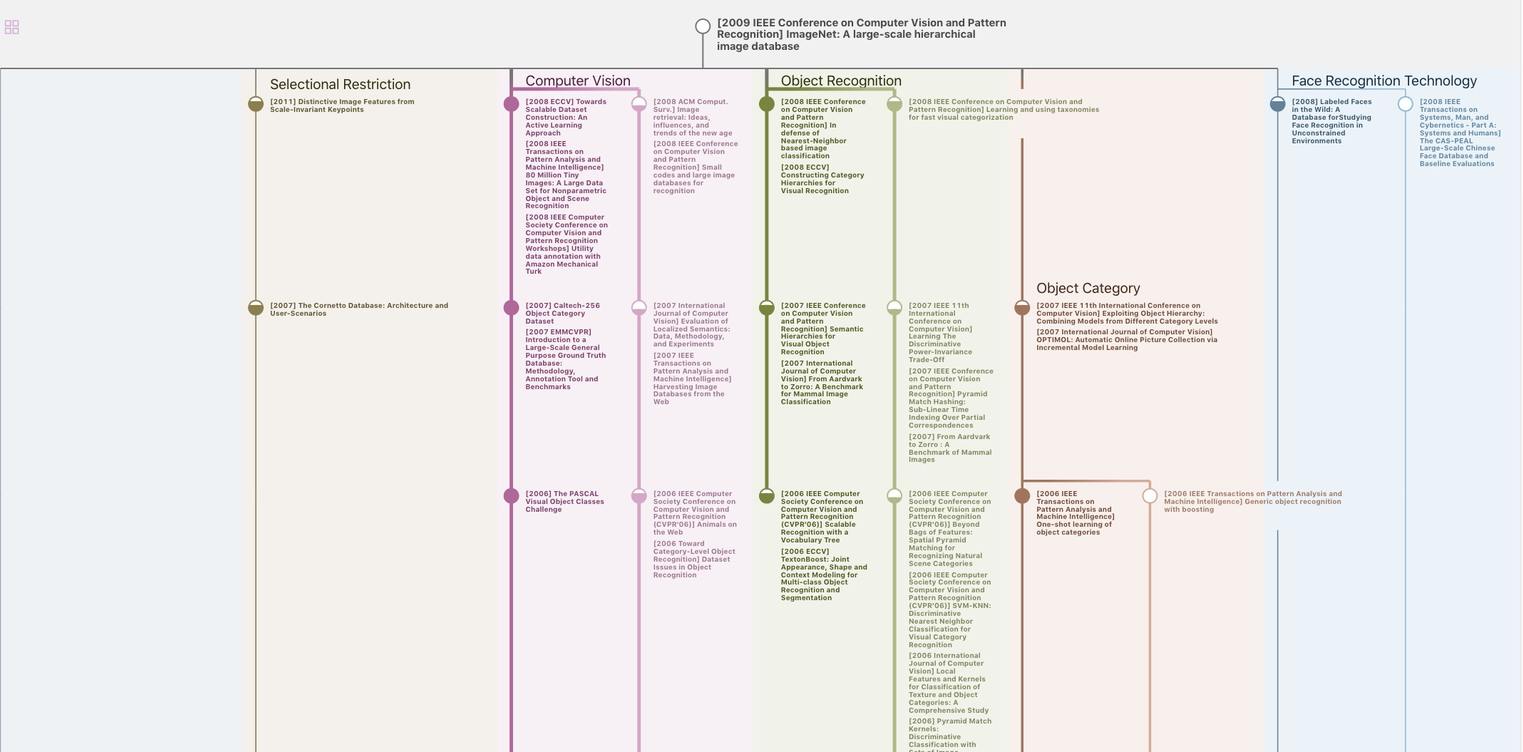
生成溯源树,研究论文发展脉络
Chat Paper
正在生成论文摘要